This article has been written by Santos Kr Sahoo pursuing a Training program on Using AI for Business Growth course from Skill Arbitrage.
This article has been edited and published by Shashwat Kaushik.
Table of Contents
Impact of COVID-19
The first year of the COVID-19 pandemic saw more than a 25% rise in cases of anxiety and depression across the globe (UN News). This rapid increase occurred because the pandemic induced different stressors, such as social distancing, economic strains and health concerns.
Even though demand is increasing, access to mental health care remains inadequate. For instance, globally, over seventy percent of individuals with psychosis are not treated. In low-income countries, this treatment gap is more severe (UN News). Major causes of global disease burden consist of mental illnesses, which result in 1 in every 6 years of life with a disability. In addition, people suffering from serious mental illness have a reduced life expectancy, dying ten to twenty years before they would normally die due to general population mortality (World Health Organisation (WHO)).
Therefore, what these figures mean is that there is an urgent need for improved global mental health services and support systems today. The continued efforts by international bodies such as the WHO can help solve these problems, improving worldwide outcomes for mental health situations.
There have been tremendous developments in artificial intelligence (AI) in the last couple of years that have resulted in paradigm shifts in a number of industries, including health care. One key area where AI is making significant strides is mental health diagnosis. Worldwide, there are millions of people with different types of mental health disorders, from depression and anxiety to more serious ones like schizophrenia and bipolar disorder. Diagnosis using traditional means for such cases mainly depends on self-reported symptoms and observations by clinicians, which can be subjective as well as biassed. Through its capacity to scrutinise mass data sets and recognise patterns, AI provides a new opportunity for more accurate and earlier detection of psychological conditions.
Current state of mental health diagnosis
Mental health has been taboo for a long time, and its diagnosis has not seen much improvement.
If you look around the world, people are having trouble keeping up with the ever changing, demanding lifestyle and showing off socialisation. Where there is very little people-to-people interaction, social events, family bonding, following traditions, culture due to technological advancement and better communication, and opportunities, on the other hand, people are moving to new countries and new places that are not in their family territories. This gives rise to people’s isolation and depression and often results in mental trauma, causing suicidal cases to increase. People often find themselves lonely, with no one to talk to and no buddy to share their plight, creating a very unhealthy and hostile environment. This leads to an increase in cases of mental health issues. This needs to be addressed urgently worldwide and, most importantly, in a civilisation like India, where you see different cultures, traditions, and religious beliefs. With the advancement of artificial intelligence, could we take advantage of AI to address this issue?
Traditional diagnostic methods in mental health
Prior to the advent of advanced technologies, mental health diagnosis primarily relied on clinical interviews, self-report questionnaires, and psychometric tests administered by specialists, such as psychiatrists or psychologists. While these methods have proven valuable in assessing symptoms and conditions, they are not without their challenges:
Clinical interviews:
- Subjective bias: The diagnostic process heavily depends on the patient’s ability to effectively communicate their symptoms and experiences. However, subjective biases can influence both the patient’s reporting and the clinician’s interpretation, potentially leading to misdiagnosis or overlooking crucial information.
- Time limitations: Clinical interviews are often constrained by time limitations, which may hinder the clinician’s ability to explore symptoms in depth. This can result in a superficial understanding of the patient’s condition and missed opportunities for comprehensive assessment.
Self-report questionnaires:
- Limited insight: Self-report questionnaires rely on patients’ self-awareness and ability to accurately identify their symptoms. However, individuals with certain mental health conditions, such as depression or schizophrenia, may lack insight into their own experiences, leading to inaccurate or incomplete responses.
- Social Desirability Bias: Patients may consciously or unconsciously provide answers that they believe are socially acceptable or desirable, rather than accurately reflecting their true experiences. This can skew the results and compromise the validity of the diagnosis.
Psychometric tests:
- Symptom interpretation variation: The interpretation of psychometric test results can vary among clinicians, leading to different diagnostic conclusions. Subjective factors, such as the clinician’s theoretical orientation or personal experiences, can influence how they interpret the test results.
- Cultural and contextual factors: Psychometric tests are often developed and standardised within specific cultural and linguistic contexts. Applying them to individuals from diverse backgrounds without considering these factors can lead to misinterpretations and inaccurate diagnoses.
These challenges highlight the limitations of traditional diagnostic methods in mental health and underscore the need for a multifaceted approach that incorporates both subjective and objective assessment tools.
Other problems associated with traditional methods
- Subjectivity: Diagnostic results may vary depending on the expertise and standpoint of the clinician.
- Stigma and underreporting: People suffering from mental problems might refuse to reveal their symptoms because of the fear associated with this status or the social perception inherent in it.
- Accessibility: There is a scarcity of personnel engagement within mental health care systems, leading to late intervention in diagnosing conditions and consequently providing treatment.
- Comorbidity: The diagnosis of many mental illnesses is complicated by overlapping symptoms between them.
Methodologies of AI in mental health diagnosis
With the help of advanced patterns of ML/Deep Learning and self-learning models, AI could play a significant role in analysing and helping with mental health analysis and further customising as per the demographic, cultural and geographical patterns. So let’s analyse how it works:
Machine learning algorithms
AI is the area of machine learning that creates computer programs to learn and make predictions. In mental health, interesting patterns are unearthed through the analysis of large data sets by ML (machine learning) algorithms, which may not be immediately apparent to human clinical analysis.
- Supervised learning: This approach involves training algorithms on labelled datasets where the outcomes (e.g., presence of a mental health disorder) are known. Common algorithms include decision trees, support vector machines, and neural networks.
- Unsupervised learning: These algorithms identify hidden patterns in data without prior labelling. Clustering techniques and anomaly detection are often used to discover subtypes of mental health disorders.
- Natural language processing (NLP): NLP techniques analyse textual data from clinical notes, social media posts, and other sources to detect linguistic markers of mental health conditions.
Deep learning
The sub-branch of ML under discussion is known as deep learning, which derives meaning from data through neural networks with layers. Of these more complex layers, there are two that are most relevant given the data type: for imaging data, convolutional neural networks (CNNs) and for sequential data (like speech or text), recurrent neural networks (RNNs). Advanced generative models can learn a higher level of decision interpretation and improve the diagnostic accuracy of mental health significantly.
Data sources
AI models for mental health diagnosis leverage diverse data sources: AI models for mental health diagnosis leverage diverse data sources:
- Clinical records: The use of EHRs in clinical research offers abundant datasets that include patient history, diagnosis, therapeutic plans, and outcomes.
- Behavioural data: With the new digital age, wearables and smartphones can track the behaviour of the patient, including activity and sleep patterns, social interaction, and other aspects of daily life.
- Speech and text data: The use of natural language analysis to study features of the spoken language and texts retrieved from social networking sites, blogging sites, and communication apps can identify markers of mental health disorders.
Applications of AI in mental health diagnosis
Early detection and screening
AI can help diagnose mental health disorders at their initial stage when there are minor deviations from normal behaviour or speech that indicate the disease. For instance, ML algorithms are capable of tracing state-run social media accounts and analysing the posts to identify symptoms of depression or anxiety in their early stages. Such a condition ought to be detected early so as to enable early treatment or management of the disorder so as to make sure that it does not worsen.
Personalised treatment plans
AI can assist in developing the appropriate treatment strategies and care plans since it is possible to use the data collected from the patient to determine the kinds of treatment that are likely to be effective in the patient’s case. Such an approach also enables consistency and quality in healthcare delivery because it bases one’s treatment on the patient’s individual needs.
Remote monitoring and telepsychiatry
Tools in AI make it possible to track the mental health of the patient on an ongoing basis while being in distant places. By incorporating AI, telepsychiatry platforms can more expansively provide diagnostic and therapeutic care to patients who are in regions with little psychiatric attention.
Enhancing psychotherapy
This is where AI can assist psychotherapy in that AI can facilitate technology that allows therapists to evaluate session’s material by using tools that would help them recognise patterns that might not be conspicuous throughout sessions. Automatic sentiment analysis techniques implemented within the NLP tool can analyse the objective emotions and topics that are being discussed in the therapy sessions to help a therapist in the process of therapeutic interventions.
Predictive analytics
Predictive analytics models can forecast the risk of developing mental health disorders based on historical data. For instance, AI can predict the likelihood of relapse in patients with chronic conditions such as bipolar disorder or schizophrenia, allowing for proactive management.
Benefits of AI in mental health diagnosis
Improved accuracy and objectivity
AI is useful in supplementing diagnostic accuracy in mental health since bias in diagnosis often occurs and in examining a large number of factors that may not easily come to the attention of clinicians. This is so because objective analysis narrows down the range of distinction by having fewer elements of chance that may make the different diagnosis not consistent.
Scalability and efficiency
This is also true because the algorithms used in diagnosis tools based on artificial intelligence can perform tasks faster than humans, which creates the possibility of screening the general population. These advantages are especially useful in increasing the availability of specialist practitioners and diagnostic services, which are scarce in mental health practice.
Cost-effectiveness
An automated diagnostic model can lead to a decrease in expenses required for the mental health care needed since it is likely to employ fewer people in contrast to the traditional models for diagnosis and it might help in early diagnosis, therefore avoiding more expensive treatments in the future.
Enhancing patient engagement
There is also a means wherein patients can participate in their own care since interactive tools can be made with AI to answer, for example. Self-assessment applications related to mental health can provide timely intervention and resources, thus enabling the patient to assume additional responsibility regarding the treatment process.
Demographic factors
Although mental health mostly depends on external parameters, certain demographic indicators, including age, sex, economic status, and ethnicity, can also be decisive. The rates at which men and women, people from different age and ethnic groups, and people from different geographical regions are affected by mental disorders may also be different from each other and may also have different levels of access to health care.
- Age: In regards to the nature of mental health, symptoms differ in children from those in adolescents and adults. For instance, while adolescents may struggle with anxiety and depression, seniors are likely to be dealing with dementia and loneliness.
- Gender: Special emphasis should be placed on the fact that different genders entail different rates and forms of mental health disorders. Female individuals show a higher rate of depression and anxiety, while male individuals might show more tendencies towards substance use and anti-social behaviour.
- Socioeconomic status: They found out that people at the lower end of the socioeconomic scale of living experience more stress, have limited or no access to professional mental health assistance, and are more likely to be diagnosed with mental health issues.
Geographic factors
Geographic location can significantly impact mental health. Urban and rural settings offer different environments that affect mental health in various ways.
- Urban areas: While urban areas may provide better access to mental health services, they are also associated with higher levels of stress, social isolation, and environmental factors like noise and pollution that can negatively impact mental health.
- Rural areas: Rural areas may face a scarcity of mental health professionals and services, leading to untreated mental health issues. However, the close-knit communities in rural areas can offer better social support networks.
Cultural mindset
Culture comprises an essential component of mental health because cultural beliefs and practices directly influence perceptions of mental health. Mental health disorders, stigma, and the treatment models also depend on the specific culture within a society.
- Stigma: Subsequently, mental health issues are still not well embraced in many cultures due to the stigma associated with it, and people with such conditions are less likely to seek medical attention. This means that many people will not seek early treatment and, therefore, could be quickly and easily cured of their condition.
- Cultural practices: Cultural practices upheld by societies and the view they hold concerning mental health affectshow they perceive and handle the issue.
- Language and communication: That is because culture and language can determine the methods patients use to articulate their symptoms and the interpretation of the progressive stages of the illness.
How demographic and geographic factors can help AI address cultural barriers
Personalised diagnostic models
AI can create diagnostic models based on demographics, even though this factor can be irrelevant in certain cases. By breaking down an enormous amount of data regarding age, sex, economic status, and ethnicity, among other factors, AI opens up the possibility of adjusting the diagnostic tests and treatment regimes according to the patient’s profile.
Remote access and telehealth
Telepsychiatry applications relevant to AI may help ensure that a particular region’s inhabitants receive adequate mental health care in venues that might be beyond the reach of most patients. These platforms offer remotely accessible services to offer mental health assistance to people who have limited access to such services or are located far from such institutions. It also allows for constant remote monitoring following a client’s admission, guaranteeing them perpetual care irrespective of their distance from the healthcare provider.
AI and linguistic cultural globalisation
So, as it can be seen, with the help of NLP tools, it can be developed an algorithm that can learn and compare the distinctions between used languages that will help solve the question of language barriers in the process of mental disorders diagnosis. Moreover, due to the multicultural training set, the results can be viewed as reducing the impact of cultural prejudice in evaluating the explainability of symptoms related to specific cultures in the development of AI.
Reducing stigma through anonymity
The benefit of adopting the use of artificial intelligence in operations that can help patients with mental disorders is that patients can get other people evaluated and find support online for what they would normally feel ashamed to seek treatment for because of the social stigmas that surround mental illnesses. These tools may well be used strategically as an avenue whereby users can express their mental health concerns without emulating embarrassment.
Enhancing cultural competence
Thus, cultural competence in AI systems may be achieved when designing and implementing systems that reflect the findings of cultural psychology and anthropology. This could assist in the development of AI interfaces that better pick up on cultural differences when it comes to mental health attitudes, thoughts and care expectations and thus be culturally responsive and adequate.
The role of data in formulating policies and resource allocation
AI is capable of utilising demographic and geographic data to pattern and find the blind spots in serving mental health. This information can then be used to make decisions at the policy level, which can then translate into the distribution of necessary resources for mental health service delivery in areas where they are needed most. For instance, decision-making can be made easier by AI to know where people need mental health services most and allocate the necessary services where they are required most.
Challenges and ethical considerations
Data privacy and security
Patient data, in particular, is underpinning many AI applications, and their application in clinical settings increases the patient’s privacy and security risks. Protection and security of Mental Health data is essential for patient’s trust and adhering to data protection regulations like GDPR and HIPAA.
Bias and fairness
So, when it comes to individuality and mental health diagnosis, AI models can only be as good as the data with which they are trained, and if these datasets are prejudiced, then the models will reinforce existing prejudices as well. It took me days to find that protecting diversity and applying so-called ‘fairness’ measures are the key to creating faithful AI.
Transparency and interpretability
It identified challenges in the capacity to understand exactly how such diagnostics are reached especially due to the ‘black box’ characteristic of models commonly applied in AI such as deep learning. For the purpose of trust and practical and efficient implementation of artificial intelligence in clinical practice, both clinicians and patients require interpretable and understandable models
Ethical use of AI
With decision-making AI in mental health comes certain risks and concerns that have to be considered during AI practice. For that, the following ethical questions should be answered: the informed consent, the possibility of AI decision-making leading to ethically contentious decisions, the use of diagnostic tools based on AI.
Integration with clinical practice
AI must automatically interface with clinical practice and healthcare platforms within the context of the real world. There is a requirement for clinicians to be educated and trained on how to employ AI tools and products and how to translate the information that they generate to fit within the paradigm of generalized efficient health care delivery.
Future directions
Advances in AI technology
Thus, the constant development of the algorithms and the influence of the AI in making decisions regarding the patients with mental illnesses and the improvement in data analysis capacities are enhancing the role of AI in mental health diagnosis. Some solutions addressed he need to debug AI models, whereas others targeted the interpretability of AI algorithms, like explainable AI (XAI).
Integration of multimodal data
This is finding a deeper understanding of mental health conditions by analysing information from multilateral sources, for example, genetic information, neuroimaging data, data about the environment, etc. The use of multimodal AI models may be the possibility to uncover more significant contextual features that would influence the development of mental health conditions.
Collaboration and interdisciplinary research
Common work effort for AI researchers, clinicians, and other parties can assist in moving the field further. Interdisciplinary collaboration can help to close the gap between research and practical application of instituted technologies, and therefore it is crucial to guarantee the applicability of the AI tools developed on the basis of the results of the study.
Ethical and regulatory frameworks
The development of comprehensive ethical and regulatory frameworks will guide the responsible use of AI in mental health. These frameworks should address issues related to data privacy, bias, and the ethical implications of AI-driven diagnostics.
Patient-centered approaches
Future AI applications in mental health diagnosis should prioritize patient-centered approaches, focusing on improving patient outcomes and experiences. Engaging patients in the development and implementation of AI tools can ensure that these technologies meet their needs and preferences.
The role of AI in mental health
Data collection and analysis
In mental health diagnosis, in particular, the ability of AI to handle huge volumes of data becomes handy. The information could be gathered from various sources, ranging from electronic health records, social media activities, and wearable technology, among others. For example, algorithms based on AI may use posts on social media to identify depression or suicidal tendencies through analysis of the linguistics involved within these texts. Similarly, physiological parameters like heart rate variation can be monitored through wearable devices, which provide the necessary statistics about the patient’s condition when integrated into an algorithm.
Natural Language Processing (NLP)
Natural Language Processing (NLP) is a subfield of AI that focusses on the communication between computers and human language. NLP algorithms can be employed to detect mental health concerns in speech and text. For example, depression can be shown by such changes in speech patterns as slower speaking rates or longer pauses. Text analysis may also point out negative sentiment, increased use of first-person pronouns and other linguistic markers that are known to be associated with mental illnesses.
Machine learning and predictive modelling
Machine learning, which is a part of AI, involves training algorithms on big data sets and making predictions or classifications. In mental health diagnosis for example, machine learning models can be fitted using clinical data to predict if a particular patient will develop a specific mental illness condition. These models can also tell which combination of symptoms or factors has a great influence on certain disorders, thus helping in differential diagnosis.
Benefits of AI in mental health diagnosis
Early detection
AI’s potential for early detection is one of its biggest advantages when it comes to mental health problems. Most mental disorders emerge slowly over time, with subtle early signs that often go unnoticed. By continuously monitoring data, AI can pick up these early signs and signals before they become serious. Early detection can lead to earlier intervention, improving outcomes for patients.
Objective assessment
Subjective assessments are the mainstay of traditional mental health diagnosis, but their application can differ widely from one clinician to another. AI is a more objective way of looking at things by evaluating data in a consistent and unbiased manner. Consequently, it can make diagnoses more accurate and minimise the chances of misdiagnosis.
Personalised treatment
AI aids in individualising treatment plans for patients. It uses information from various sources to determine which treatments will be most effective based on patient profiles. The result is a tailor-made care approach that enhances treatment effectiveness while reducing trial-and-error prescribing.
Real-time monitoring
AI-powered wearable devices can continuously monitor physiological and behavioural data, enabling real-time tracking of mental health conditions. This can help identify sudden changes or triggers, allowing for prompt adjustments in treatment and support.
Access to care
AI-powered mental health tools can expand access to care, particularly in underserved areas with limited mental health professionals. Online platforms and mobile apps equipped with AI can provide remote assessment, support, and guidance to individuals who might not otherwise have access to traditional mental health services.
Cost-effectiveness
AI-driven mental health diagnosis can be more cost-effective than traditional methods, as it can reduce the need for multiple visits to healthcare professionals and provide continuous support remotely.
Reduced stigma
AI-powered mental health tools can help reduce stigma by providing anonymous and confidential access to assessment and support. This can encourage individuals to seek help without fear of judgement or discrimination.
Continuous learning and improvement
AI algorithms can continuously learn from new data, allowing them to adapt and improve over time. This means that AI-powered mental health diagnoses can become increasingly accurate and effective as more data is analysed.
Challenges and ethical considerations
Data privacy
The use of AI as an instrument for diagnosing psychological disorders poses serious issues related to privacy. It is important that sensitive information, such as personal health records and social media content, be treated with caution so that patient confidentiality can be protected. Therefore, robust data security measures must be put in place, along with appropriate informed consent by patients so as to effectively address these anxieties.
Algorithm bias
AI models perform only as well as the data they have been trained on. Where training sets suffer from bias or poor representation, AI models may perpetrate these biases, thus creating unfairness in their selection processes. Hence, there is a need for inclusive and diverse training datasets that represent different demographics and backgrounds if we want AI algorithms that produce fair results in mental health diagnosis contexts.
The human element
Although AI is capable of assisting in diagnosis, it should not substitute the human touch in mental health care. AI may assist with diagnosis but it cannot replace the human quotient in mental healthcare. It should be an adjunct to, rather than a replacement for, human care.
Case studies
Depression detection
One remarkable use of AI in mental health is its role in detecting depression. Researchers have created machine learning models that can analyse users’ social media posts and detect those who are at risk of depression. These models look out for linguistic markers such as negativity, decreased social presence, and changes in posting habits. Research has revealed that these models can predict depression with great accuracy, sometimes even before a clinical diagnosis is made for an individual.
Predicting Schizophrenia
AI is also being used to predict the onset of schizophrenia. By combining genetic data, brain imaging and clinical records, AI models can identify people who are most likely to develop schizophrenia. Early detection offers monitoring and early treatment, which improve the long-term outcomes of patients.
The future of AI in mental health
The future of AI in mental health holds tremendous promise for revolutionising diagnosis and treatment. As technology advances, AI will play an increasingly pivotal role in numerous aspects of mental healthcare.
One exciting area of exploration is the integration of AI with virtual reality (VR) to create immersive therapeutic experiences. VR can transport individuals into simulated environments, where they can safely explore their emotions and behaviours in a controlled setting. AI can enhance this experience by providing real-time feedback and guidance, tailoring the therapy to the individual’s needs and progress. For example, AI-powered VR therapy could help people with social anxiety practice interacting with others in a virtual environment, gradually building their confidence and skills.
Another potential application of AI in mental health is monitoring treatment adherence. Medication management is a crucial aspect of many mental health treatment plans, but non-adherence can significantly impact outcomes. AI-powered systems can track medication intake through various methods, such as smart pill bottles or smartphone apps. They can then send reminders, provide educational resources, and alert healthcare professionals if a patient misses a dose. This can help improve medication adherence, leading to better treatment outcomes.
Moreover, future advancements in AI may enable the development of more sophisticated algorithms capable of understanding complex human emotions and behaviours. Current AI models are primarily trained on text and image data, but they often struggle to capture the nuances of human emotions and interactions. As AI technology evolves, it could incorporate more diverse data sources, such as facial expressions, vocal patterns, and physiological signals. This could lead to AI systems that can better understand and respond to the emotional needs of individuals, enhancing the efficacy of AI-powered mental health interventions.
In addition to these specific applications, AI is also likely to impact the mental health field in broader ways. For example, AI could be used to analyse large datasets of mental health records to identify patterns and trends that can help researchers and clinicians better understand the causes and progression of mental illness. AI could also be used to develop personalised treatment plans, tailoring interventions to the unique needs of each individual.
As AI technology continues to advance, its potential to transform mental health care is limitless. By harnessing the power of AI, we can create more effective and accessible mental health interventions, empowering individuals to take control of their mental well-being and live fulfilling lives.
Integration with healthcare systems
To effectively integrate AI into mental health care, it needs to seamlessly fit into existing healthcare systems. This means that technologists, clinicians, and policymakers must collaborate to ensure that AI tools are user-friendly, based on evidence, and compliant with regulatory standards.
Continuous learning and adaptation
AI models need to be continuously updated and improved to keep up with the latest research and clinical practices. This involves ongoing data collection, validation, and fine-tuning of algorithms to maintain their accuracy and relevance.
Conclusion
AI holds great promise for revolutionising the diagnosis of mental health conditions. By enabling early detection, providing objective assessments, and offering personalised treatment, AI has the potential to enhance outcomes for millions of individuals struggling with mental health disorders. However, addressing challenges related to data privacy, algorithm bias, and the importance of human empathy is crucial for the ethical and effective implementation of AI in mental health care. As technology advances and research progresses, AI will undoubtedly play an increasingly significant role in the diagnosis and treatment of mental health, paving the way for a future where mental health care is more precise, personalised, and accessible.
References
- Esteva, A., Robicquet, A., Ramsundar, B., et al. (2019). A guide to deep learning in healthcare. Nature Medicine, 25, 24–29.
- Shatte, A. B., Hutchinson, D. M., & Teague, S. J. (2019). Machine learning in mental health: A scoping review of methods and applications. Psychological Medicine, 49(9), 1426-1448.
- Inkster, B., Stillwell, D., Kosinski, M., & Jones, P. B. (2016). A decade into Facebook: Where is psychiatry in the digital age? The Lancet Psychiatry, 3(11), 1087-1090.
- Bedi, G., Carrillo, F., Cecchi, G. A., et al. (2015). Automated analysis of free speech predicts psychosis onset in high-risk youths. npj Schizophrenia, 1, 15030.
- McKernan, L. C., Nash, M. R., Carr, B. G., et al. (2019). Digital mental health: The use of social media in mental health diagnosis and treatment. Journal of Psychiatric Research, 113, 68-75.
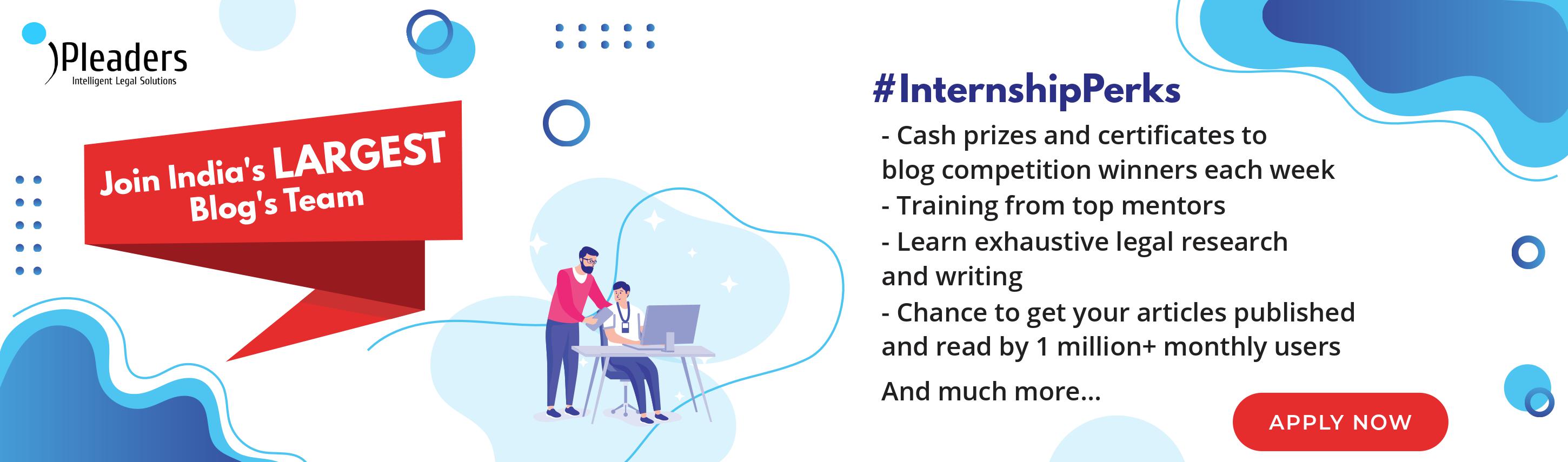