This article has been written by Tanya Dua pursuing a Diploma in Patent Law & Analysis (USPTO): Searches, Specifications, Prosecution, Litigation and Licensing from LawSikho.
Table of Contents
Introduction
The discovery of a drug occurs when there is a disease or condition for which suitable medicinal conditions are unavailable. Initially, the discovery occurs at the academic level by setting up data that can possibly be useful in curing the disease. This helps scientists to set up a base for producing suitable medicine for curing the disease.
What is AI
AI, i.e., artificial intelligence, is a boom in human mankind. It is a combination of human ability and technology, or it is when technology is modified in a way that makes it work just like a human brain. Still, it is not just limited to the brain of a single human being; it is a cluster of thousands of human brains at a given point in time.
For a few decades, AI was limited just to computers, but as time passed, it has now been totally incorporated into our lives. Nowadays, AI is used for every big and small activity, from helping with household chores to operating a person and predicting the future, just with the specific data and instructions given to it. AI is designed in a way that for every single research project, AI combines the data of thousands of researchers to provide a single output.
AI has made medical terminologies way easier due to the ability of AI to give precise and accurate outcomes by analysing the stored data and commands given, as well as the ability of AI to reach places that are way more difficult for humans to counter with. Several branches of AI have been involved in the different actions that take place in every aspect of life. Branches of AI include machine learning, robotics, computer vision, etc.
The potential of artificial intelligence in drug development
Artificial intelligence (AI) has revolutionised various industries, and its potential in drug development is immense. AI offers a range of tools and techniques that can enhance the efficiency and effectiveness of drug discovery and development processes.
Drug discovery:
- AI can analyse vast amounts of biological data, including genomic information, protein structures, and disease pathways, to identify potential drug targets. This process is much faster and more efficient than traditional methods, which often rely on manual labour and serendipity.
- Machine learning algorithms can predict the structure-activity relationships of compounds, enabling the rapid identification of lead molecules. This is a critical step in drug discovery, as it helps to narrow down the field of potential candidates for further testing.
- Virtual screening techniques powered by AI can sift through large libraries of compounds to identify those most likely to bind to a specific target. This process is much faster than traditional high-throughput screening methods, which can take weeks or even months.
Drug design:
- AI algorithms can design new molecules with desired properties, such as high affinity for a target, specificity, and low toxicity. This is a complex and challenging task, but AI is well-suited to it due to its ability to handle large amounts of data and to learn from experience.
- Generative models can create novel molecular structures not found in nature, expanding the chemical space for drug discovery. This is a powerful tool for discovering new drugs that are more effective and have fewer side effects.
- AI can also optimise existing drugs to improve their potency, efficacy, and safety. This is a valuable tool for drug companies, as it can help them to extend the lifespan of their products and to improve the lives of patients.
Clinical trials:
- AI can analyse clinical trial data to identify patterns and trends that may not be readily apparent to human researchers. This can help to improve the design of clinical trials and identify potential problems early on.
- Machine learning algorithms can predict patient outcomes and responses to treatment, enabling personalised medicine approaches. This can help to ensure that patients receive the most effective treatment for their individual needs.
- AI can also be used to design more efficient clinical trials, reducing the time and cost of drug development. This is a critical factor in bringing new drugs to market faster and at a lower cost.
Drug safety:
- AI can analyse large volumes of safety data to identify potential adverse effects and drug interactions. This is a critical task, as it helps to ensure the safety of drugs for patients.
- Natural language processing techniques can extract information from medical literature and electronic health records to identify safety signals. This can help to identify potential problems early on, before they cause harm to patients.
- AI can also be used to develop predictive models for drug toxicity, allowing for proactive risk management. This can help to prevent adverse events and to ensure the safety of drugs for patients.
Drug manufacturing:
- AI can optimise manufacturing processes for drugs, reducing costs and improving quality. This can help to make drugs more affordable for patients and improve the efficiency of the drug supply chain.
- Machine learning algorithms can monitor production lines for anomalies and potential quality issues. This can help to identify problems early on, before they cause significant damage.
- AI can also be used to automate quality control processes, ensuring the consistency and safety of drugs. This can help to ensure that patients receive high-quality drugs that are safe and effective.
Overall, AI has the potential to transform drug development by making it faster, more efficient, and more effective. As AI technologies continue to advance, we can expect even greater innovations in drug discovery, design, clinical trials, safety monitoring, and manufacturing.
Steps involved in drug discovery
Identification of target and then validating
The first step involved in drug discovery is identifying the target. The target here refers to the site that is the root cause or involved majorly in causing the disease. Once the target is identified, it becomes easier for the scientists to evaluate the type of drug that will bind the site efficiently.
The phenomenon known as “Lock and Key” is involved in binding the drug with the site of action. This phenomenon states that just like a lock can be opened with a single type of key, in the same way, the drug that will be more effective will be the one that properly fits and binds with the site of action.
So, it is very important to have the right drug, as it depends upon the drug to enhance or inhibit the ability of the target.
Identification of the right drug can be done by:
- Firstly, testing the compounds, which can be helpful.
- Then evaluating the previously known drugs that could cure the new disease discovered.
- Manipulating the drug according to the information gained while examining the disease.
Preclinical research
After the formulation of the drug, it becomes important to check the toxicity level of the drug. There is a high possibility of toxicity for human beings. The preclinical research can be conducted by both methods: In-vitro and In-vivo
- In-vitro: In this process, the study is performed inside the laboratory on the cells, tissues, or other parts of an organism.
- In-vivo: In this process, the study is performed on living organisms, specifically laboratory animals, which may include genetically modified mice, frogs, cats, fishes, etc.
Toxicity level can be evaluated by the above methods, and it also includes giving a high dosage of the drug to animals to check the changes and responses taking place in the animal’s body, behaviour, and functioning.
After the data is obtained from the above observation, it is provided to regulatory authorities to conduct clinical trials on human beings.
Further, after the toxicity levels, other important factors are also determined, like:
- Amount of drugs appropriate for human use.
- How the drug is absorbed, distributed, metabolised, and excreted.
- Method by which the drug must be administered in the body, i.e. orally or by injecting.
- Effects of drugs on different groups of people (Age, Sex, Gender)
- The environmental factor is also taken into consideration.
- How the drugs will react under the influence of the other drugs.
Clinical trials
After the trials on the animals, the next step involved in drug discovery is clinical trials, which refer to the study or trials that involve human beings. To conduct trials appropriately, some protocols have been set. On the other hand, the researchers go through the previous data available, and then they decide:
- Who and how many people can participate?
- How long will it take to achieve all the data required?
- The dosage and method by which the drug must be given?
- What data needs to be collected, reviewed, analysed, and reported?
Regulatory approval
After the successful clinical trial, the drug is then made to enter the market for usage. The final data is a combination of the data collected previously (by preclinical trials) as well as real-world evidence, and then it is filed with a marketing authorisation submission for approval for public use.
The report must include:
- Proposed labelling;
- Saftey updates;
- Drug abuse information;
- Patent information;
- Any data from the studies that may have been conducted outside the country;
- Institutional review board compliance; and
- Direction of use.
Post-market safety monitoring
The actual picture of any drug forecast when it has been released in the market and has been used by people for a few years now.
This monitoring helps in obtaining data on the following:
- Modifications that are required based on the results.
- What serious side effects can take place?
- Modifications of dosage if needed
- If it counteracts with any other type of medications
- What other alternates are available in the market?
FDA (Food and Drug Administration) is responsible for safeguarding public health, so it closely keeps an eye on the companies to monitor the safety of the drugs properly, using the FDA Adverse Event Reporting System database.
Artificial intelligence in drug discovery
AI can be used in various steps for the discovery of the drug:
AI in the identification of target: Several approaches are involved:
- With the involvement of AI in drug discovery, AI models such as machine learning make it easy to go through a large number of genomic data points at a time, which eventually makes it easier to reach the appropriate drug in a short span of time.
- It becomes easier with the help of AI to remove the complexity of the interactions, like protein-protein interaction, to help ease the identification of target sites.
- AI covers a large range of compounds that could have a chance of binding to the target and, as an outcome, can quickly predict the most accurate compound.
- AI is also helpful in confirming the role of the target in the evolution and progression of the target.
- It has become possible to have personalised drugs from patient to patient based on their genomic and clinical data.
AI in preclinical research
- The datasets used by AI, like machine learning, help in predicting the toxicity levels of a compound using pre-existing data.
- AI is used in making models that, to a large extent, mimic human anatomy, which makes it easier for scientists to test compounds, set dosages, determine the effects of compounds, etc.
- It helps in predicting the pharmacokinetics of the drug, i.e., absorption, distribution, metabolism, and excretion, which ultimately leads to the prediction of how the drug will behave upon entering the human body.
- It is due to this that the need for testing drugs on animals has been reduced.
AI in clinical trials
- Using AI, it has become easier to analyse the patient’s genomic, clinical, and medical data before testing any drug on them. So, in return, it became easier to choose the right candidate for clinical trials.
- During the trial method also, AI is useful in the modification of the amount, type, and precautions needed to be taken during the period of trials itself.
- Clinical trials have always been a time-consuming process, but due to AI, it has become a fast process due to the ability to automate drug integration and report generation.
- It also helps to know the long-term side effects of the drugs.
- The accuracy of the drug development has been increased.
- The quality of the drug formation has been improved.
AI in regulatory approval
- Due to the updating of time at every interval, it has become easier to submit the analysis that has been done throughout the trials.
- AI can predict missing information, summarise all the data, and can automatically detect errors.
- Provides insights that are useful for the regulatory bodies to improvise and make decisions regarding.
- It also ensures that the drug generated is correct according to the standards.
AI in post-market safety monitoring
- Helps in monitoring the long-term effect of the drug.
- AI can keep a record of all the patient data, from medical conditions to life insurance and smart appliances (which are used to monitor day-to-day activities) and alert the patient much before an incident takes place.
- AI helps in tracking the records of patients with drug-executing companies.
- AI can also detect the activities of a patient through their social media and search history related to drugs in any way.
- It helps in keeping a record of patient problems and feedback across the globe.
- Can also predict the interaction between any other medication.
Challenges of using AI
Artificial intelligence (AI) has the potential to revolutionise drug discovery, but several limitations and challenges need to be addressed:
- Data requirements:
- AI models require vast amounts of high-quality data for training and validation.
- Collecting, cleaning, and preparing this data is time-consuming and expensive.
- Data acquisition can be particularly challenging for rare diseases or conditions with limited patient populations.
- The data used in datasets may not be representative of the real world, leading to biased or inaccurate results.
- Data bias:
- The data used to train AI models can be biased due to factors such as demographics, socioeconomic status, or underlying health conditions.
- Biased data can lead to models that make unfair or inaccurate predictions.
- For example, if a dataset is predominantly composed of data from a specific population group, the model may not perform well when applied to other populations.
- Lack of interpretability:
- AI models often lack interpretability, making it difficult to understand how they arrive at their predictions.
- This lack of transparency can make it challenging to identify and correct errors in the models and to ensure they make decisions based on relevant factors.
- The inability to interpret model predictions can also hinder regulatory approval processes.
- Continuous data updates:
- AI models need to be continuously updated with new data to maintain their accuracy and effectiveness.
- This can be a significant challenge, especially in rapidly evolving fields like drug discovery.
- Failure to update models regularly can lead to outdated or inaccurate predictions.
- Privacy concerns:
- AI-based drug discovery often involves processing large amounts of sensitive patient data.
- Ensuring the privacy and security of this data is critical to maintaining public trust and avoiding ethical violations.
- Robust data protection measures and compliance with privacy regulations are essential.
- Infrastructure and knowledge gaps:
- Effective use of AI in drug discovery requires advanced computational infrastructure and specialised knowledge in both AI and drug discovery.
- Many industries lack the necessary resources and expertise to fully leverage AI for drug development.
- This can hinder the adoption and implementation of AI-powered drug discovery tools and platforms.
To overcome these challenges and fully harness the potential of AI in drug discovery, ongoing research and collaboration are needed to:
- Develop more robust and interpretable AI models.
- Mitigate data bias through careful data selection, preprocessing, and validation.
- Ensure data privacy and security through robust data protection measures and compliance with ethical guidelines and regulations.
- Provide the necessary infrastructure and training to researchers and professionals to enable widespread adoption of AI in drug discovery.
Conclusion
The intersection of AI and drug discovery has revolutionised the lives of human beings. Due to the large datasets used by AI, the discovery of drugs has become easier, cost-effective, highly accurate, decreased level of toxicity, and highly tailored. Although even after so many positive effects of AI, there are some negative effects, like computational errors, over-reliance, ethical issues, etc.; if these issues are resolved, AI can transform drug discovery to a whole new level.
References
- https://Www.Ncbi.Nlm.Nih.Gov/Pmc/Articles/Pmc7577280/,
- https://Www.Ppd.Com/What-Is-A-Cro/Drug-Discovery-And-Development/,
- https://Www.Cell.Com/Trends/Pharmacological-Sciences/Fulltext/S0165-6147(23)0
- https://Www.Sciencedirect.Com/Science/Article/Pii/S1740674906000503
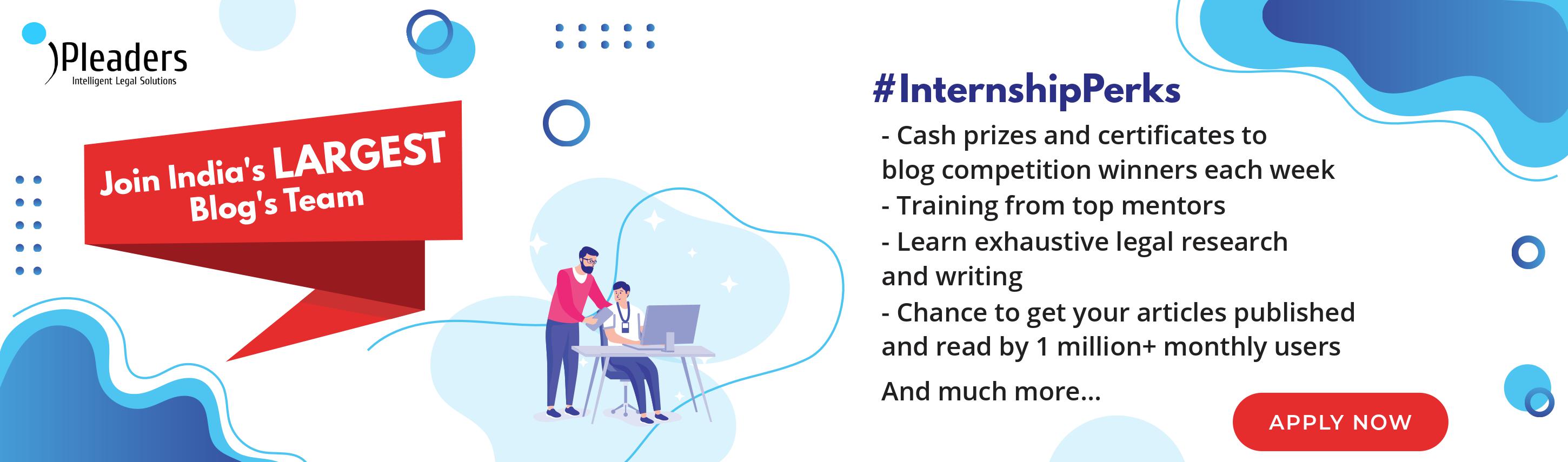