This article was written by Nisha Sharma, pursuing the Diploma in Content Marketing and Strategy Course from Skill Arbitrage, and edited by Koushik Chittella.
Table of Contents
Introduction
The dynamism of retail businesses is a very well-known fact everywhere. The ever-fast-changing businesses, with many complex activities on the ground, involve multiple stakeholders, intricate logistics, and different levels of demand uncertainty. Owing to technological advancements, the retail industry has been going through a dramatic shift in gaining deeper insights into consumer behaviour, anticipating demand fluctuations, or streamlining inventory management to reduce costs and improve customer satisfaction.
Integrating advanced technologies like artificial intelligence (AI) into supply chain and retail inventory management offers retail businesses unprecedented opportunities for strategic decision-making to increase efficiency, optimise processes, and keep meeting the ever-changing demands of consumers. AI’s consumer demand prediction helps retailers deliver the right products to end consumers in stores and helps manufacturers predict the quantity of raw materials to be considered for production. Retailers gain deeper insights into consumer behaviour for predicting demand fluctuation and streamlining inventory management, resulting in reduced costs and improved customer satisfaction with the use of AI methods. Thus, AI’s role in the end-to-end supply chain and retail inventory management appears promising.
AI-powered predictive analytics increases efficiency in inventory management for the speedy fulfilment of consumers’ demands for personalised experiences and seamless interactions. Retailers are under pressure to cater to the personalised demands of consumers while also maintaining profitability and sustainability. From demand forecasting to inventory optimisation to the delivery of the product, AI’s predictive analytics feature assists retailers in analysing vast amounts of accumulated data, automating routine activities, and optimising decision-making processes. In the following accompanying sections, we are going to drill down into the role of AI-driven predictive analytics while managing inventories in the retail industry.
Understanding Retail Inventory Management
It is the process of streamlining the demand of the consumer and the supply of the retailer while managing the excess of both the demand and the supply, reducing the associated costs, and maximising the liquidity in profits. Effective retail inventory management minimises inventory carrying costs and gives a better understanding of sales patterns. Retailers have more data to facilitate their business operations through the use of inventory management systems. The process of retail inventory management enables retailers to have access to information on the following:
- Product locations
- Quantities of the product to be considered
- Consumer behaviour towards the product
- Maintaining the stock of the product
- Environmental factors affecting the sale of the product
- Performance of the products by location and sales medium
- Profit margin gained by the product
Usage of retail inventory management
Following are the reasons why inventory management is a necessity in today’s retail industry:
- Reduces the costs of having excess inventory
- Minimises the potential for product to be out-of-stock
- Improves the Profit Margin
- Prevents product from wastage and expiration
- Enhances multi-channel and omnichannel performance and order fulfilment
- Improves connectivity between the processes and increases efficiency
- Reduces the unavailability of the products in demand
- Makes Supply Chain Management easy
- Boosts customer satisfaction
- Improvement of prediction accuracy
10 basic steps in retail inventory management
Below is an image representing 10 Basic Steps in Retail Inventory Management:
Source: Oracle Netsuite
The above-mentioned steps in the illustration are as follows:
Step 1: Create a centralised record of all products
Step 2: Identify stock location
Step 3: Do regular and accurate stock counts
Step 4: Combine sales and inventory data
Step 5: Create a purchasing process
Step 6: Establish a method for markdowns
Step 7: Build a stock-receiving process
Step 8: Create a system for returns
Step 9: Determine a dead stock procedure
Step 10: Pick your inventory KPIs (Key Performance Indicators)
Role of AI in retail inventory management
AI’s power and capabilities are not hidden anymore. With time, one of the fastest-evolving technologies (AI, in this case) is also becoming powerful, and its contribution to businesses is commendable; they cannot shy themselves away from it. It is quite imperative to mention that AI has started making its presence in retail inventory management as well. As per an article published by Precedence Research, experts predict that there is going to be a steep rise in global AI in the retail market from just $8.41 billion in 2022 to $45.74 billion in 2032. In the same report, it is mentioned that, based on application, the retail inventory management segment captured around 16% of revenue share in 2022.
Keeping track of stock levels, predicting customer demands, and accordingly maintaining optimal levels of inventories in real-time are some of the responsibilities that AI is capable of performing in the current age. This helps retailers reduce costs, save money, and keep in-demand products in stock, leading to customer satisfaction and retention, thus increasing sales and profitability.
Benefits of AI in retail inventory management
Some of the distinct benefits that AI provides in retail inventory management are
- Optimised Inventory avoids stock excess or stock deficiency
- Accurate forecasting resulting from detailed data analysis
- Reducing waste by identifying slow-moving or excess stock
- Minimising holding costs by ensuring that businesses stock the right quantity of products
- Efficient Automation saves time and resources
- Real-time insights for enhanced and quick decision-making
- Improved customer satisfaction by making the product available when they need
Challenges faced by the usage of AI
- Data quality and availability. The quality of data determines the quality of prediction and, thus, the quality of decision-making.
- Cost of implementation. Implementing AI-based solutions can be hefty on the companies’ pockets, as this involves expensive technological infrastructure supported by trained staff.
- Skilled personnel. Recruiting specialised skill personnel or upskilling existing ones can be challenging for some businesses.
- Integration with existing systems. Integrating AI solutions with existing systems can be complicated, and ensuring a seamless connection is a critical consideration.
- Security and privacy. Using robust AI to counter cyber threats should be considered while handling sensitive data, which can otherwise be alarming in terms of security and privacy.
Understanding predictive analytics in retail businesses
Predictive analytics: meaning
Predictive analytics is the practice of using historical data to analyse and forecast customer demand and company performance by running a series of practical experiments to improve customer experiences and satisfaction. Predictive analytics is used to design promotions for targeted, interested shoppers and provide them with valuable suggestions and information. Increased sales leading to maximised profit, fine-tuned targeting for marketing campaigns, a better customer experience leading to increased customer loyalty, and better staff scheduling are some of the common benefits of predictive analytics in retail businesses.
Benefits of using Predictive Analytics
The following illustration shows the data-driven benefits of using predictive analytics for retailers:
The explanation of the above illustration about the benefits that predictive analytics offer is as follows:
- Customer retention & identification: It helps retailers identify and retain existing customers and also identify prospects to attract them with valued offers.
- Inventory planning and risk mitigation: Retailers can use predictive analytics to plan inventory well to avoid losses.
- Personalised customer service: It assists retailers in assessing consumers to provide them with services tailored to their needs.
- Accurate real-time insights: With the help of predictive analytics, retailers can continuously evaluate the purchasing behaviour of their consumers to make the right decisions.
Major challenges
The major challenges here would be:
- Determining the right pricing;
- Using real-time data to decide on the quantity, location, and type of stock to be maintained
- Recommendation engines analyse enormous available data sources and extract and present decision-making information.
- Revenue forecasting based on historical purchasing behaviour.
Integrating AI and predictive analytics
As per a report featured by Chris Kelly on LinkedIn: “A major retail chain implemented AI-driven predictive analytics for inventory management. The system analysed years of sales data, seasonal trends, and purchasing behaviours. The result? A 20% reduction in stockouts and a 15% decrease in excess inventory.”
There is a constant flux experienced by retailers while managing inventory. This could be due to a lone or a combination of issues like unpredictable demand, inefficient procurement, inventory imbalance, store replenishment, on-shelf availability, or warehouse replenishment. AI-driven predictive analytics empowers retailers to make data-driven decisions for improved operational efficiency and maximum profitability. So that the visit of the customer to the retail outlets becomes a wonderful and fulfilling experience for them and the retailers, optimisation of the inventory is very significant. The stores’ shelves having all kinds of attractive product items are the reasons for the happiness of the customers and are crucial for successful retailers. Stock deficiency can hurt finances, leading to loss of revenue and customers. Stock excess leads to an increase in storage space along with the carrying cost. Optimisation is important to fill the gap between demand and supply to avoid things like stock deficiency and stock excess.
The Inventory Management System powered by AI equips retailers to maintain inventory levels optimally in real-time, predict customer demand, minimise stock excess and stock deficiency, and thus ensure the availability of the right products at the right time, leading to increased sales. AI-driven predictive analytics carries the potential to predict demand and adjust inventory levels accordingly following the analysis of historical and real-time data, existing market trends, environmental factors, and social media sentiments. Retailers feel empowered to have a competitive advantage by gaining actionable insights into the rapidly fluctuating market and can now anticipate future trends and make informed decisions accordingly. AI-driven predictive analytics provide retailers with vast datasets for analysis, patterns, and trends in consumer behaviour to make accurate predictions. Retailers can use AI-powered predictive analytics to
- forecast demand
- forecast procurement optimisation
- optimise inventory management
- return forecasting
- predict store replenishment
- assortment optimisation
- on-shelf product availability
- promotion forecasting
- predict warehouse replenishment
AI-driven predictive analytics in retail is the result of the efforts of a conglomeration of technologies like machine learning algorithms, natural language processing (NLP), and computer vision. These three technologies together empower retailers to extract valuable insights from data, enhance customer experiences, and optimise operational processes. Systems can learn from historical and real-time data and improve their predictions over time with the help of machine learning algorithms. Retailers can use machine learning for product recommendations, pricing optimisation, and fraud detection.
NLP enables systems to interact in natural language while improving communication between retailers and customers. Chatbots and virtual assistants are empowered by NLP, which enhances customer support and engagement in online and offline environments. Analysing customer reviews, social media interactions, and feedback and providing insights into customer sentiments are also features supported by NLP. Retailers can adjust strategies based on sentiment analysis, responding to both positive and negative feedback.
Systems can analyse and interpret visual data, contributing to a deeper understanding of customer behaviour because of the underlying activities of computer vision. Whether it is shelf monitoring, tracking customer movements, or implementing innovative in-store experiences, retailers use computer vision to accomplish these tasks. Customers can find products by uploading images, enhancing the search and discovery process using the visual search capabilities of computer vision.
Benefits and outcomes of using AI-driven predictive analytics
There are several benefits to using AI-driven predictive analytics while managing retail inventory:
- Enhanced Forecasting Accuracy: The forecasting errors are reduced, and accuracy is improved, leading to better inventory planning and reduced stockouts.
- Cost Reduction: Minimised carrying costs and reduced excessive safety stock due to optimised inventory levels.
- Increased sales and customer satisfaction: Avoiding stockouts and the availability of the right products at the right time results in increased sales and enhanced customer satisfaction, thereby improving the overall shopping experience.
- Improved Operational Efficiency: Streamlining processes, reduced manual intervention and provided real-time insights, allowing retailers to focus on strategic initiatives.
- Scalability and adaptability: An AI model can tune itself to changes in patterns of demand, seasonal variations, and market fluctuations.
Case studies of successful implementations of AI
Below are a few examples of successful AI implementations at world-renowned big giants:
- Amazon uses machine learning algorithms to process users’ browsing behaviour, purchases, and preferences. Remarketing these specific target audiences by offering customised product suggestions has increased the company’s sales along with its customer base.
- Alibaba’s AI chatbots on its e-commerce platforms give users personalised shopping experiences through customised assistance and recommendations. By streamlining the shopping process, offering real-time support, and improving overall customer experience, customer satisfaction is enhanced.
- AI is predominantly used by Walmart, particularly for inventory management and customer service. By constantly evaluating customer buying habits and the inventory position, any excess stock is reduced by optimising inventory levels. The use of AI bots enhances storefront stock monitoring and control.
- Starbucks uses AI algorithms to make sense of patterns in customer orders, preferences, and historical information, which helps in the accurate anticipation of what customers order, reducing their waiting times, making operations more efficient, and enhancing in-store personalisation.
- Zara has adopted AI to analyse real-time sales data, social media inclinations, as well as external elements to predict demand. AI has empowered Zara to optimise inventory levels, thereby eliminating redundant stock while tracking consumer tastes more responsively in a bid to have flexible inventory management operations.
Future of AI-driven predictive analytics
AI-driven predictive analytics has already proven to be promising in the world of retail inventory management. This revolutionary implementation is meant to assist retailers in managing inventory while forecasting demand, managing stock levels, and catering to fluctuating and evolving consumer preferences and demands. AI-driven predictive analytics lets retailers address potential equipment failures before they disrupt inventory operations. In this way, retailers can minimise the shutdown, optimise resource allocation, and ensure uninterrupted inventory flow. The evolving components of AI (machine learning, NLP, and computer vision) are going to produce sophisticated algorithms that aspire to enhance retail inventory forecasting, providing accurate demand predictions and comprehensive market trend analysis.
The evolving AI technology is going to seamlessly and undisputedly integrate with Enterprise Resource Planning (ERP) systems, aligning procurement, inventory control, broader supply chain mechanisms, marketing, and after-sales services for retaining clients or customers and maintaining long-term relationships with them. AI-driven predictive analytics efficiently supports dissecting and understanding customer behaviour, preferences, and purchasing patterns, which helps manage the inventory well. This approach not only assists retailers in pricing strategies but also supports them in refining promotional activities to align more closely with consumer demands. Such technological advancements play a pivotal role in reaching data-centric, highly adaptive inventory management paradigms that vouch for operational efficiency and agility, customer satisfaction, and ultimately, maximised profitability in retail.
Conclusion
In this article, we have explored the dynamics of inventory management in retail while recognising the emerging technological trends of AI-driven predictive analytics. AI-driven predictive analytics support retailers in unprecedented ways for handling regular inventory-related issues like unpredictable demand, inefficient procurement, inventory imbalance, store replenishment, on-shelf availability, or warehouse replenishment. The fusion of advanced technologies like machine learning algorithms, NLP, and computer vision has enhanced the strategic decision-making capabilities of retailers to cater to the immediate needs of consumers, reduce unnecessary costs, and increase the profit thereof. With constant technological advancements, retailers are increasingly participating in learning and adopting AI-driven predictive analytics for anticipating market trends and predicting consumer behaviours to provide them with personalised services. AI-driven predictive analytics also helps retailers in decision-making by exploring demand patterns, market trends, pricing strategies, and overall operational efficiency based on historical and real-time data. One of the beautiful aspects of implementing AI-driven predictive analytics is that they are naturally and vigorously adaptive to changing patterns of demand, seasonal variations, and market fluctuations. These technologies can integrate seamlessly into the existing system, empowering retailers to understand their customers and create innovative solutions such as personalised visual searches and automated checkout experiences for them. We also discussed the prospects of the seamless integration of AI-driven predictive analytics with efficient and large systems like ERP.
References
- https://www.researchgate.net/profile/Satish-Kathiriya/publication/379048200_Strategic_Innovations_and_Future_Directions_in_AI_driven_Retail_Inventory_Management_A_Comprehensive_Review_and_Pathway_Analysis/links/65f890f232321b2cff8c3f55/Strategic-Innovations-and-Future-Directions-in-AI-driven-Retail-Inventory-Management-A-Comprehensive-Review-and-Pathway-Analysis.pdf
- https://medium.com/simplegpt/case-study-ai-driven-predictive-analytics-for-inventory-management-in-retail-297de6a65d84#id
- https://katanamrp.com/blog/ai-for-inventory-management/
- https://www.linkedin.com/pulse/ai-inventory-management-predictive-analytics-christopher-kelly–05q3c/
- https://www.researchgate.net/publication/378293870_AI-DRIVEN_PREDICTIVE_ANALYTICS_IN_RETAIL_A_REVIEW_OF_EMERGING_TRENDS_AND_CUSTOMER_ENGAGEMENT_STRATEGIES
- https://hypersonix.ai/blog/optimising-retail-operations-with-predictive-analytics-and-ai/
- https://pavion.com/resource/how-ai-is-transforming-inventory-management-in-retail-operations/
- https://www.sumoanalytics.ai/retail
- https://www.fepbl.com/index.php/ijmer/article/view/772
- https://www.researchgate.net/publication/378143631_ANALYZING_THE_ADOPTION_AND_INFLUENCE_OF_AI_IN_RETAIL_SUPPLY_CHAIN_OPERATIONS
- https://www.ultralytics.com/blog/ai-for-smarter-retail-inventory-management
- https://throughput.world/blog/ai-in-the-retail-industry/#:~:text=Walmart,store%20inventory%20tracking%20and%20management
- https://www.netsuite.com/portal/resource/articles/inventory-management/retail-inventory-management.shtml
- https://profitbooks.net/retail-inventory-management-best-practices/
- https://mastechinfotrellis.com/blogs/ai-analytics-and-data-sciences/predictive-analytics-in-the-retail-industry
- https://www.precedenceresearch.com/artificial-intelligence-in-retail-market
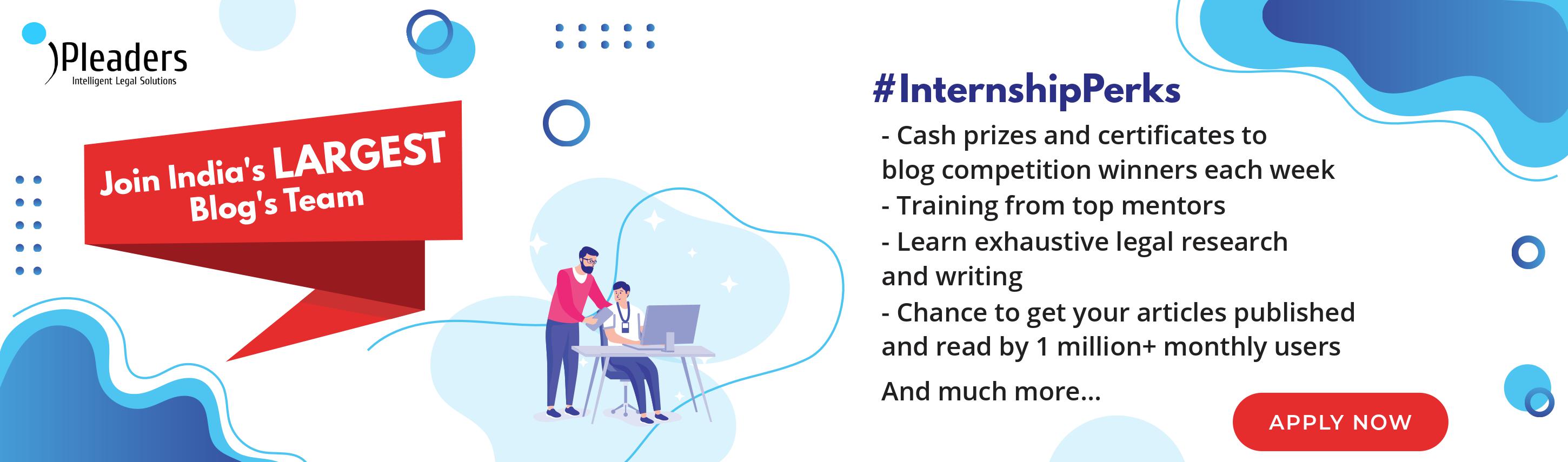