This article has been written by Pallav Bhuyan pursuing a Training program on Using AI for Business Growth course from Skill Arbitrage.
This article has been edited and published by Shashwat Kaushik.
Table of Contents
Introduction
AI, or artificial intelligence, is the capacity of machines to do work or solve problems that humans find difficult to do or solve. AI is revolutionising the agriculture industry. Agriculture depends on various factors like weather, soil condition, irrigation, the amount of fertiliser to use, controlling pests, and the right time to sow and yield the crop. Now that programmed machines are intelligent enough to detect these factors or improve the underlying functionality of these factors, they can directly improve crop yield, both in quality and quantity.
The AI-powered solution helps farmers produce more with fewer resources and improves the quality of the crop produced. In the article, we will see more elaborately how AI improves agriculture.
AI in weather detection
Agriculture heavily depends on the weather factor. The weather plays an important role in determining the right time to sow the seeds. Now, AI is used to detect future weather. Our economies are becoming increasingly dependent on weather, especially with the rise of renewable energy and growing climatic changes resulting in heat waves, hurricanes, droughts, and floods. The field of weather forecasting could gain a lot from the addition of AI. AI models can create forecasts in just seconds and moreover, they are more accurate than traditional weather forecast systems. Accurate weather forecasting helps the farmer take steps to safeguard their crops from some natural calamities, such as more than normal rainfall or droughts. The farmers can choose the right time to sow the seeds, which will be very beneficial for the crop. With the help of AI-based weather forecasting, farmers can access accurate data on rainfall, which helps them to decide the right time and amount of irrigation, fertiliser application and sowing time of seeds, and reaping time of crops and fruits.
AI in soil management
Soil is one of the most important factors in successful agriculture. The right amount of nutrition and minerals is crucial for proper crop growth and yield. By testing the sample soil of the land, the required amount of mineral deficits was determined using AI science. The challenge lies in the fact that the nature of the soil changes with time and places and regions. The AI must be fed with the ever changing data so that it can analyse accurate results. The AI is smart enough to determine the changing factors and suggest accurate and precise decisions to be made to make a sample of soil rich for farming. So, we can improve the quality of the soil by adding or removing the required amount of whatever minerals or nutrients the soil needs for the maximum yield of that particular crop.
Weed management and disease monitoring
Weed management is an important part of successful crop production. Weeds are the unwanted wild plants and shrubs that grow along with our crops and utilise and steal much of the resources like nutrients, water, and space from the crop, thus hampering the crop yield and quality as well. So, it is very important for successful farming to remove the weeds without harming the valuable crops. However, medicines used to kill weeds, called weedicides, can harm the crop as well. AI plays an important role in using the right weedicides at the right place at the right time and in the right quantity and composition so that the main crop remains unharmed and the weeds get killed and removed. Identification of the weed is also a challenge for the farmer.
The use of drones to spread weedicides accurately and uniformly has been a revolution.
Some of the machine learning techniques used for weed identification are CNN, DCNN, SVM, ANNs, KNN, ShuffleNet-v2, and VGGNet.
AI-driven platforms use advanced computer algorithms to identify and manage weeds with remarkable accuracy. AI is used to identify, target and kill weeds without harming the valuable crop.
Cameras and machine learning algorithms are used to distinguish between crops and weeds based on differences in shape, size, and colour. Once weeds are identified, AI-driven systems are used to spray herbicides and weedicides targeting only the weeds. New and continuous update of the AI system is also necessary for accurate results. AI also powers autonomous robots that can physically remove weeds from the ground.
Use of AI in improving irrigation system
There is growing freshwater scarcity all over the world. The traditional irrigation method has not been that efficient in catering to the ever-growing agricultural demand and climate changes. So a smart irrigation system is being introduced. It uses AI and ML (machine learning), which use real-time data from various sources like soil moisture sensors and weather forecasts to tailor water schedules and the extra amount of water needed from irrigation.
It not only optimises water usage and helps in water conservation but also contributes to sustainable agriculture, thus improving crop yields, reducing operational costs, and minimising environmental impact. Smart irrigation emerges as a vital solution for enhancing food security and the preservation of natural resources. Smart irrigation systems are adaptable to changing climatic conditions. Moreover, it can be tailored to the needs of the specific crop type, soil condition, and weather condition; thus, the customisation helps in determining the amount of water needed for the optimal growth of the respective crop.
Implementation of smart greenhouse model
A smart greenhouse model is a greenhouse that uses the Internet of Things and a cloud-based system with sensors to improve the productivity of vegetables, fruits and plants and automatically monitor the greenhouse. It increases efficiency and reduces the need for human interventions. It has sensors to monitor temperature, humidity, and moisture levels and automate the use of mechanical appliances like ventilation, irrigation, and heating.
The model requires much data science research, like the nature of the crop, crop information, crop growth information, crop moisture information, land area information, etc. The data model is made and analysed. The intelligent agricultural greenhouse mainly relies on the large data sets and intelligent control of the cloud platform, where information is stored in global storage accessible to all systems and continuously updated in real-time but monitored.
The smart sensors with cloud technology help in continuous, real-time, and accurate monitoring of the greenhouse environments (temperature, moisture, and humidity) and automatically trigger on and off the lights, heating or cooling system, irrigation pumps, etc. This results in shorter crop growth and a large harvest yield.
Yield mapping and predictive analytics
Around the world, the impact of climate change on crop yields is enormous. To help farmers make informal decisions, there is a need for an interactive prediction system. Random forest algorithms, along with various other techniques, are used for data mining for crop yield prediction. Machine learning is the key technique used for this purpose. Statistical methods are also used for crop yield prediction. Machine learning techniques are used to obtain the following goals:
- Increase the accuracy of crop yield prediction.
- Provide an easy-to-use user interface.
- Analyse different climate parameters such as cloud cover, rainfall, temperature, etc.
This will help farmers know the yield of their crop before cultivating and make appropriate decisions, and data mining is useful for predicting crop yield.
Data is the most important part of any machine learning process. Collecting the data in a diverse climate-changing environment is the most challenging task. Researchers and scientists from all over the world worked collaboratively to collect various forms of data from various sources, manage and analyse them, and feed the machines with that anonymous set of data so that they could create a productive and accurate solution. These are sometimes called smart farms. The use of technology for farming purposes to obtain greater yields.
Conclusion
The integration of artificial intelligence in agriculture has the potential to revolutionise the farming industry. It improves efficiency, productivity, and sustainability. It also makes it more effective by replacing humans with intelligent and effective machines for doing complex and delicate tasks of farming like sowing seeds and spraying pesticides and weedicides. AI also helps farmers make data-driven decisions, optimise resource allocation, and enhance crop yields. However, some challenges need to be addressed, such as cost, access, data gathering and privacy, skill gaps, and infrastructure compatibility, as well as the ever-changing climate conditions and demands. Global warming is also posing a threat to humanity as well as agriculture. By addressing these challenges, AI can be successfully integrated into agriculture, leading to improvements in food security, economic growth, and environmental sustainability. AI not only improved the quantity of crops but also the quality as well. Though AI has come a long way in improving crop yield and agricultural resource management, a lot more research and implementation are yet to be done.
Many uneducated rural populations in many parts of the world are unaware of the possibilities that AI can bring to improving crop yields and agricultural resource management. Many are afraid to implement because of cultural and religious factors. The need of the hour is to engage more and more farmers in believing in the true value of AI and implementing it with full trust, with the help of the governing body as well.
References
- https://www.researchgate.net
- https://thecannabiswatcher.com/pages/articles/industry/2023/12/3/weedsly-revolutionizing-weed-management-artificial-intelligence
- www.sciencedirect.com
- https://www.academia.edu/44885925/Crop_Yield_Prediction_Using_Machine_Learning_Techniques
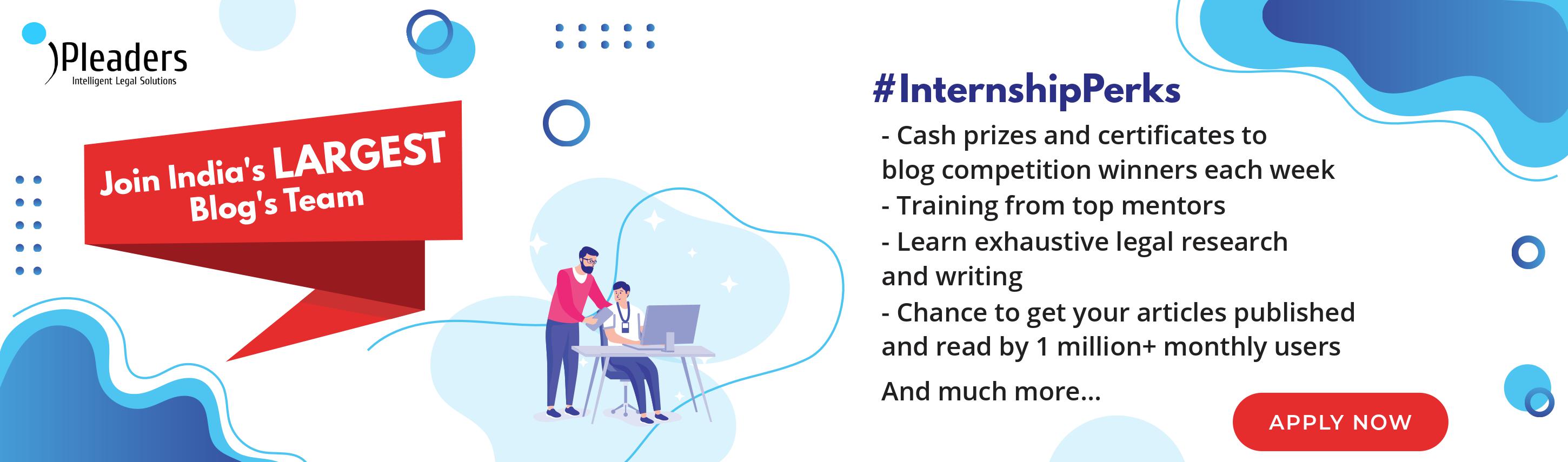