This article has been written by Sirisha Govindarajula pursuing a Remote freelancing and profile building program from Skill Arbitrage.
This article has been edited and published by Shashwat Kaushik.
Table of Contents
Introduction
Data has become a resource that every business aims to utilise efficiently in this era of digital transformation. With Artificial Intelligence (AI) techniques becoming widespread in business analytics, companies can now gain deep insights from their data, automate intricate operations and forecast future outcomes with much ease. This article aims to present an overview of the tools and techniques of AI that have become indispensable for business analytics today.
What is AI
Artificial intelligence, in its broadest meaning, refers to intelligence exhibited by machines, especially computer systems, which are simulated to think like a human brain, see like a human eye and learn like a human.
AI has been the topic of research in the field of computer science over the years and it has found its moment in time now. AI is no longer science fiction; it is not a thing of the past. It is right here, in this very instant, fast-paced and transforming our lives and the way we perform daily tasks.
What are business analytics
Business analytics refers to skills and processes used to examine and investigate data to make informed decisions to solve problems in business.
What is the role of AI in business analytics
Artificial intelligence in business analytics involves the application of various AI methods, such as data mining, predictive analysis and statistical analysis to detect patterns, trends, correlations and opportunities that may be hard for a human to detect. This results in more efficient and thorough data analysis of large and complex data sets, leading to informed business decisions. AI enables companies to achieve competitive advantage through efficiency, accuracy and forecasting trends.
Top AI techniques in business analytics
Machine learning algorithms
Machine learning algorithms form the backbone of AI in business analytics. These are computer programmes that learn from data and make predictions or decisions, rather than explicitly coding the rules.
Supervised learning
In supervised learning, the algorithm is presented with known output labels and the goal is to learn the mapping between input data and output labels, so as to make predictions on new data. Regression and classification are common applications. Sales forecasting is an example where supervised learning can be applied to predict tomorrow’s sales based on past data.
Unsupervised learning
Unsupervised learning algorithms operate on data for which we have no prior knowledge about the output variable. The goal is to discover patterns, structures, or anomalies in the data. Clustering and association are common techniques used in market segmentation. Clustering essentially groups together customers with similar feature values and is commonly used for targeted marketing.
Reinforcement learning
In reinforcement learning, the algorithm is rewarded for the correct action and penalised for the wrong action. The goal is to learn the strategy to follow that achieves the desired outcome. Association rule learning and dynamic programming are common techniques used in optimising business flows, for example, in supply chain management to learn the best strategy to minimise cost and maximise value.
Natural language processing (NLP)
Natural language processing reverses the process usual in human interaction by making machines understand and interpret ways in which humans communicate.
Sentiment analysis
By analysing customer reviews, social media and feedback forms, sentiment analysis helps businesses understand the attitude of customers towards their products. This can, in turn, be used to enhance products, customer service, etc.
Text mining
Text mining or text classification, extracts valuable insights from text data (unstructured data).
Chatbots and virtual assistants
By using AI techniques, businesses can now provide enhanced customer service through chatbots and virtual assistants that promptly respond to customer inquiries and perform useful tasks like booking appointments, answering frequently asked questions (FAQs), and so on.
Predictive analytics
Predictive analytics is the use of statistical methods for forecasting future events based on historical data.
Predictive modelling
Predictive modelling builds models using historical data to predict future outcomes. For example, market demand for products in various locations can be predicted using time series forecasting, which is in turn derived from historical sales data. Customer behaviour in certain demographic regions and likely sales performance of products in the market can also be predicted using clustering and classification techniques.
Anomaly detection
An anomaly or outlier, is an observation that lies outside the expected range of data. Sometimes anomalies are irrelevant, but sometimes they may point to operational issues, fraud or other irregularities. For example, in banking and financial services, an anomaly detection algorithm can flag a transaction as suspicious and potential fraud if it lies outside a certain range considered normal for that particular account. Another example is manufacturing, where detecting anomalies can point to defective products.
Data visualisation tools
Data visualisation tools are used to represent data in graphical or pictorial forms for better understanding and interpretation.
AI-enhanced dashboards
By using AI techniques, enhanced dashboards can provide real-time visualisation of important metrics in business. Users can also slice and dice the dashboard to gain quick insights.
Interactive visual analytics
Interactive visual analytics tools provide the ability to manipulate visualisations to explore different scenarios and gain faster insights. These advanced visual analytics tools support better decision-making, as they allow users to get a clear and complete understanding of the data.
Robotic process automation (RPA)
Businesses use RPA, which uses AI, to automate simple, repetitive tasks and speed up business processes.
Routine task automation
RPA is useful for automating routine and repetitive tasks like data entry, invoice processing and report generation. This allows employees to dedicate their time to more creative work.
AI integration
RPA integrated with AI has the capability to perform complex, intelligent tasks that require judgement and flexibility. For instance, AI-powered RPA can interpret customer service requests and offer personalised solutions.
AI-driven business analytics tools
Sisense
Sisense is a next generation AI based business analytics platform that allows users to analyse complex datasets and derive actionable insights from them. It employs machine learning algorithms to handle large data sets and provide interactive visualisations.
Microsoft Power BI
Microsoft Power BI is a business analytics tool that leverages AI to derive valuable insights from raw data. It can be integrated with multiple data sources and offers real-time analytics.
Tableau
Tableau data visualisation and analytics software leverages generative AI to automate routine tasks, recommend questions, and deliver answers in plain English. Perfect for business leaders and non-technical employees to drive complete business insights and become more data driven.
Qlik Sense
Qlik Sense is an AI powered analytics platform that enables businesses to make data driven decisions. It employs associative data indexing to reveal hidden patterns in the data.
ChatGPT
ChatGPT assists business analysts by mining datasets, detecting patterns and using predictive analytics for decision-making. It can detect risk factors, explain data in plain English and even write SQL commands. Whitelist data for privacy and review results for relevance and accuracy.
Polymer
With Polymer, users can upload Excel and Google Sheets spreadsheets, then see data visualisations and interactive dashboards without writing a single line of code or using any other technical skill.
MonkeyLearn
MonkeyLearn is an AI tool that visualises text data, conducts sentiment analysis, organises it into categories, and generates charts without requiring any coding skills.
Akkio
Akkio is an easy-to-use AI tool that helps you forecast, analyse, and visualise your data. It helps create forecasts and reports for business planning, sales forecasting, and marketing analysis, and no technical expertise is needed.
Google Looker
Google Looker is a data modelling and analysis tool that runs in the browser and allows users to build custom applications with embedded analytics. Part of Google Cloud, Looker processes large volumes of data but does not produce scheduled or unscheduled reports.
Splunk
Splunk is a data management platform that enables users to monitor, search, analyse, and visualise data from any source. Splunk is suitable for security and observability use cases and can process large volumes of data in real-time.
TIBCO Spotfire
TIBCO Spotfire provides a single, unified source of truth for both structured and unstructured data. Point and click, no-code tools for data exploration and visualisation provide rapid insights from all your data. Spotfire applies machine learning to streaming data to alert you to changes and developments as they occur in your business.
RapidMiner
RapidMiner is a data science platform for pattern detection and solving business challenges. It includes text mining, data preparation, data analysis, data visualisation, predictive analytics, and full-scale automation. RapidMiner provides no-code tools but also allows users to write code to create their own custom models.
Challenges and ethical considerations
Data privacy
Data privacy and security are the biggest areas of concern when it comes to applying AI in business analytics. Enterprises need to ensure they comply with data privacy regulations and take due diligence in implementing appropriate security controls to safeguard sensitive user information.
Bias in AI models
AI algorithms can learn bias if the data they are trained on is biassed. Learning can result in unfair and discriminatory outcomes. Enterprises need to ensure diverse and representative data samples are used to train the AI model to reduce any bias in the results.
Regulatory compliance
Enterprises need to ensure their AI implementations comply with industry regulations and standards. Transparency in AI processes and AI explainability and accountability are key considerations here.
AI and business analytics future trends
AI and big data
The integration of big data and AI technologies will intensify to help enterprises leverage larger and more complex data sets for better predictions and insights.
AI driven decision making
AI will play a bigger role in strategic decision-making for businesses. AI systems will help enterprises make data driven decisions aligned with their business goals and objectives.
Emerging technologies
Future advancements in AI, such as quantum computing and other advanced neural networks, will amplify business analytics capabilities. Businesses will be able to solve complex business problems and expand their business horizons using advanced AI technologies.
Conclusion
AI applications in business analytics should be seen not only as technology, but as a shift in the paradigm that helps gain a superior competitive edge. For example, to improve customer satisfaction and retention, Netflix leverages AI to understand viewing patterns and predict the shows users are most likely to enjoy on their platform. Coca-Cola uses AI to track social media mentions in real-time, thereby enabling them to take prompt actions based on consumers’ reactions to their campaigns, and so on. AI techniques and tools find their use in business applications ranging from data visualisation and predictive analytics to automating mundane operations and optimising decision-making processes.
Deeper insights, improved efficiency and innovation will enable organisations to make rapid progress, once they get accustomed to these tools and techniques. For example, Amazon employs AI-based algorithms to manage its inventory and supply chain, thereby ensuring on-time deliveries and minimising costs. Undoubtedly, the face of business analytics is changing, and AI is at the heart of this transformation. Data that could previously only answer ‘what’ happened will now cater to ‘what’s next’ and aid in creating value for stakeholders, thereby driving growth. AI in business analytics has thus become indispensable for organisations today.
References
- AI in BI: Role, Benefits, Examples & Future | TEC (technologyevaluation.com)
- 15 Top Trending Artificial Intelligence(AI) Tools List in 2024 (geeksforgeeks.org)
- 15 Business Intelligence Tools (BI Tools) to Use in 2024 | Sprout Social
- AI In Business Analytics: Turning Data To Decision (adamfard.com)
- How AI Is Revolutionizing The Role Of The Business Analyst (forbes.com)
- What is Artificial Intelligence (AI) in Business? | IBM
- MIT Sloan Management Review
- Harvard Business Review
- MIT Sloan Management Review
- McKinsey & Company
- Forbes
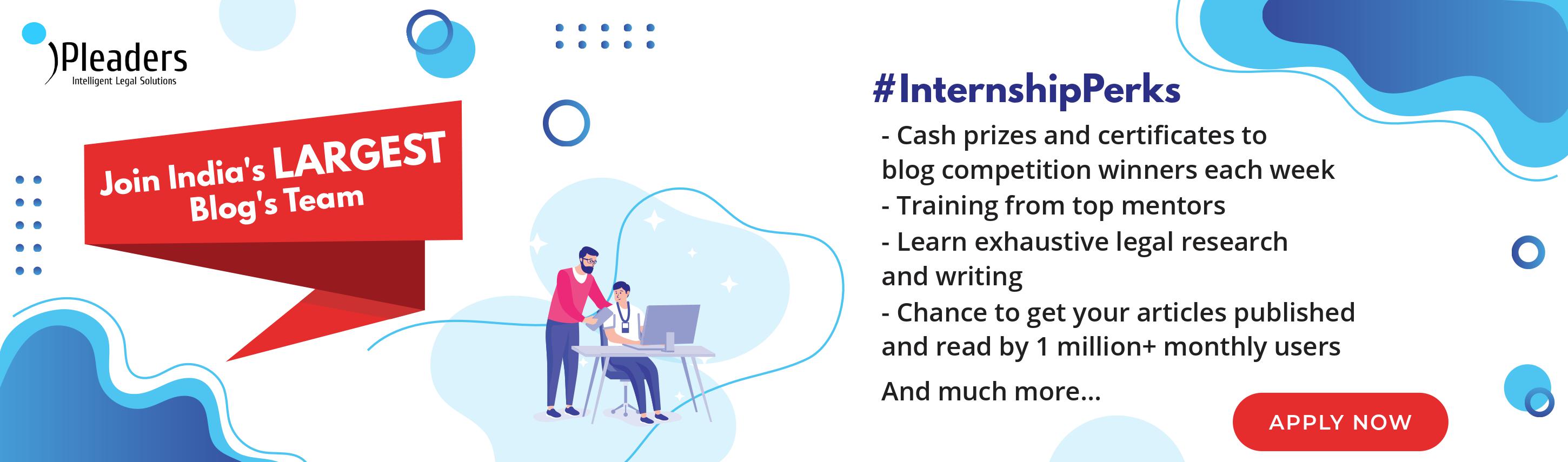