This article is written by Anupam Bhaduri, from Jogesh Chandra Chaudhuri Law College, Calcutta University. This is an exhaustive article that deals with algorithm bias and the ethical issues of artificial intelligence.
Table of Contents
Introduction
Each day, there are scores of decisions that are taken by an algorithm on our behalf. These algorithms enter our life dressed as in-app voice assistants, chat-bots or search analysis data or any other form of computer-based data search result one can think of. Algorithms help make decisions by keeping one informed. It would not be falsehood if someone claims that algorithms make decisions on our behalf. Algorithms are trusted more than human beings since they are impartial. Or are they?
Detection of algorithmic bias
When talking about machine learning, it is better to consider it as a means of training a computer. The computer is fed lots of data based on different metrics to identify a particular search result. For example, a program can be made to identify a book based on the data that has been fed to it. This process takes lots of trials and the process is then honed based on errors made by the program. The process is however not this simple because the desired result is seldom this objective. Often these algorithms are incomplete, or their decision-making systems are not entirely balanced which gives rise to the phenomenon known as ‘algorithmic bias’. This results in instances of rampant discrimination such as one where Google image identification algorithm identified two black-skinned people as gorillas. Perhaps a more appalling one is one where Facebook identified a Palestinian posing by a bulldozer with “attack them” written in Hebrew. The man was questioned for hours before someone pointed out that the Hebrew translated to “good morning”. Although both Facebook and Google apologized, the problem persists.
Ethical dimensions associated with AI
The introduction of AI also carries the baggage of ethical dimensions with it. While it is argued that AIs are impartial, it is also to be noted that impartiality alone cannot answer the argument for robust functioning. An AI after all is only an instrument, and thus begs the question that in case of a malfunction, on whom will the onus of accountability lie? Secondly, an AI cannot be said to be entirely free of bias. AI is only a program. If the data fed to it is biased, the AI will further lead to biased decision making in already prevalent places.
AI is also the reason many people have become wary about their online safety especially after the data breach scandal by Cambridge Analytica. Katie Evans rightly laid bare her doubts when she said that the data collected by AI can certainly help a lot in explaining how a world is, but it does little to explain how the world should be. Hence, if this data is wrongly interpreted due to biased programming, the day-to-day errors will cement discrimination.
Thirdly an AI cannot be relied upon for making moral decisions. Moral status in humans originates from the obligations that we share towards our peers. In cases of practical ethics, disputes about moral permissibility cannot be resolved by an AI. for instance, abortion disputes and the moral dilemma of it cannot be simply resolved through weighing out pros and cons as done by an AI.
Ethical issues in AI
The world in 2020 has several ethical issues when it comes to AI. The following elucidates on the major ethical questions that are yet to be resolved:
-
Loss of jobs
The primary fear with the introduction of AI is that a lot of people will lose their jobs. However, that is not the entirety of it. With the advent of AI, a whole sector of jobs will disappear. However, the loss of jobs is argued by many. Since robots will be used to complete tasks, a large group of men will find employment in making and maintaining the robots.
-
Increasing inequality in the distribution of wealth
The issue of economic inequality has been one of the most common problems of the present political scenario. With the advent of AI, that will further increase. In a factory, for instance, the present labour laws provide the foundation for decent pay and fixed working hours for the workers. However, with a robot, the company saves on wages. Also, with minimal maintenance, the production lines are capable of manufacturing round the clock. Added to that, small scale industries which are unable to acquire these robots will face stiffer competition while resulting in further accumulation of wealth in the hands of a few.
-
Accountability issues
Machine learning is a long process. With increased demand in the market, there will be an abundance of half-finished AI taking on day-to-day tasks. These improperly fed AIs will make discriminatory choices which might give rise to a violation of certain important rights, similar to the Palestinian mistake by Facebook. In these situations, it is still unclear as to who will bear the liability of the AI’s fault. While it is argued that without being exposed to a vast bundle of data and AI cannot ‘learn’, the law needs to decide what follows if the AI makes a costly mistake.
-
AI bias
The whole credibility of AI is based on the central idea that an AI is unbiased. However, in most scenarios that is not the case. AIs are made by biased people. Moreover constant exposure to a specific metric of discriminative data develops biases in the AI.
-
Data privacy
Digital theft is a new and dangerous form of criminal activity that only increases with increasing reliance on artificial intelligence. A rogue AI or one that has been made with malevolent intentions can cause significant damage. In a TEDx speech, Jay Tuck mentions that an AI automatically updates its programming. This means that the enhanced AI is not a result of the original programming but a result of the data material that it was subjected to. Hence, a sufficiently well equipped AI could impersonate the online identity of any individual, snoop into conversations and be a potent method to commit money laundering and bank frauds.
-
Singularity
Although this has only been majorly depicted in Hollywood movies, the technological singularity is a real issue. A lot of the information revolution that is going on around us is dependent on how fast a machine learns to adapt and learn from new data. Based on this, a significant amount of change has appeared in the hardware to facilitate such expedited learning of an AI. There has been an increasing tendency to fit the regular-sized machines inside chips, to create a replica of the human brain circuitry. These advancing neuromorphic chips will not only help an AI to learn faster, but it will also help them predict plausible futures and resist the potential threats to its systems. Humans are on top of the food chain not because of sheer brawn, but because of the superior intelligence we possess. However, at this rate, there will come a day when an AI will be far more superior in mind than the average human.
Kinds of bias in artificial intelligence
A pertinent question is if AI can be biased. It is often held that an AI is the result of a neutral set of algorithms that keeps on learning from real-life experiences. However, this is not entirely true. An AI may learn but the necessary skill to process certain data is already in the programming. Hence, the way the particular AI functions may cause a waste of resources or be discriminatory against some minorities.
-
Personal bias
AI can and does cause a significant amount of personal bias based on historic trends. This problem has surfaced with prominent institutions and has laid bare that the scope of training oneself through patterns is insufficient, and without human intervention, can cause disastrous results. A potent example of this is when Amazon had to turn off their recruitment AI since it categorically removed applications of women as potential candidates. This blemish was picked up by the AI, while it crunched data and came to know that men were hired more than women, which is interpreted as men being better suited for the post than women. There have been other instances where the police database have wrongfully predicted that black-skinned people are habitually repeat-offenders or where a hospital refused taking patients because the AI would not allow the entry of people with names of non-European origin.
-
Environmental bias
To think of an AI going against nature would not be a threat to environmentalists before 2005. Today all weather stations use AI to predict and thus mitigate damage caused by natural disasters. However, now the entire world is also well aware of how taxing it is on the environment to train an AI. The World Economic Forum report of 2019 demonstrated that the training of an AI causes energy consumption at rates that are beyond sustainable. The carbon footprint of training an AI is five times more than that of an average American car for its entire life, including the manufacture. In numbers that would be 626,000 pounds of Carbon Dioxide equivalent. A further error and a trial method called the neural architecture of BERT (one of the costliest models) yielded 1400 pounds of carbon dioxide equivalent. For the uninitiated, that is the amount of carbon waste generated by a round trip trans-America flight for one person. Major houses such as Google strived to adopt renewable resources of energy.
Reasons behind AI bias and its subsequent reduction
AI algorithms are biased due to the creators of the algorithm being biased. An AI comes across huge volumes of data metrics and crunches them to identify a pattern. This is how an AI learns. Although it is self-educating in nature, in theory, we realise that the AI ‘educates’ itself by comparing the data to some set specific standards. This is where the biased opinions of the creator come into play. If the standards against which the AI compares an input is not fair, the AI will adapt unfair patterns, thus resulting in discriminatory actions.
A prominent and perhaps the only way to reduce bias in an AI is to bring algorithm writers from all parts of the community. Other than a more inclusive approach, it will be nigh impossible from making AI truly unbiased.
Another method to prevent algorithm bias is to subject the AI to regular audit aimed at identifying bias. Since audits prompt the review of both input data and output decisions, a third-party evaluation will force remediation and provide detailed analysis of the algorithm’s behaviour. Developing a thorough audit report should then be subjected to different sectors of society to better detect and deter biases. In this regard, Facebook undertook a civil rights audit to determine how its AI identified and dealt with the issues raised by the users, the majority of the users being from protected groups. After a thorough analysis, Facebook has pledged to commit regular audits to handle civil rights issues with better redressal systems. The increased audit has shown results by banning white nationalist contents.
The surest method to keep AI abreast of biases is to ensure that there are proper redressal systems in case some biased decisions are taken by an AI. The issue then needs to be taken up and solved with a sense of duty towards the wronged individual/community. The solution to this problem lies in employing more human intervention in the form of data interpretation moderators.
Indian laws to prevent algorithmic bias
Concerning AI, the efforts by the government have mostly been a separate and a parallel approach to identifying the problems posed by AI. The NITI Aayog was tasked to prepare a detailed analysis on this front. In a discussion paper published in June 2018, the scope of AI bias was left almost completely untouched. The report fails to make any significant comment on the ethical, social and technical difficulties faced due to AI bias. Matters regarding data protection and privacy issues were lightly brushed upon. The possibility of having a well-intentioned algorithm address issues disproportionately against minorities and marginalised communities are also left unconsidered.
Regarding regulatory bodies, there seems to be some consensus between the conclusions drawn by MeitY and NITI Aayog. Both have agreed that no good shall come by opening up the codes of the AI to public scrutiny but to rather have repeated feedback by conveying the explainability of the AI. The process of explainability opens up the methods used by the AI to reach a certain result based on the inputs of the user. This will help to garner concise feedback in cases of bias.
To enhance transparency in the dealings of the AI, the #AIForALL campaign by NITI Aayog focuses on technical solutions to fix discriminatory bias. Usage of regulatory checks through applications like ‘AI Fairness 360’ of International Business Machines can help bring regulatory checks and transparency in the process. User-end tools like Google’s ‘What-if’ application could also be used since the user will not need the knowledge of a programming language to run regulatory checks on the AI.
The basis of equality across the country is articulately held in Article 15(2) of the Indian Constitution. It stipulates that no discriminations shall be made against the citizens based on caste, creed, sex, place of birth and religion and no individual can be restricted from entering a public place. It is yet to be seen how the Indian lawmakers adapt this to the ever-evolving technological laws.
International legislations to combat AI bias
The European Union in its white paper sought to outline measures that would address the ongoing biases observed in scores of AIs. The guiding principles of the paper were human oversight and principles of non-discrimination, accountability, transparency and robustness. The report sought to highlight the AIs operating in healthcare, transportation, energy employment and biometric identification sectors as ‘high risk’ AIs. A large reformative measure was focused on the employment of regular government audits and meeting defined standards of high-quality representational data. Regulatory bodies could be set up that would deal with complaints of bias towards marginalised communities.
The EU has also agreed that to account for the robustness of the high-risk AIs, a certain depth of technical knowledge is required that is lacking in the government regulatory bodies. The EU has acknowledged that the complexity could cause the AIs to trick the federal regulatory structures and in turn essential legislation like the Civil Rights Acts, the Americans with Disabilities Act, Fair Housing Act, etc.
Negative impacts of algorithmic bias
Algorithms being biased pose severe problems. A resemblance of this problem is found in the regulation of AI in matters of life and death. Drones are operated by AI and have the power to conduct aerial warfare should the need arise. Although the drones are manned and the decision of whether to kill the enemy ultimately lies with the operator, it can be easily stated that this decision cannot and should not be handed over to an AI. The onus of judgement calls cannot be left to an AI that treats data by set specific codes if yes and no.
A similar example can be drawn in AIs that find the names of Europeans to be ‘more beautiful’. This is an intensely biased view that the AI has inculcated owing to the data it has been exposed to and the metrics against which it judges this data. Algorithm bias leads to systemic discrimination against communities. Especially marginalised communities.
Implications of AI on health systems
Application of AI in healthcare is a revolutionary approach. It is to be noted that the AI treatment is not restricted to the ‘high income’ group but can also help resolve problems in remote areas. However, algorithm bias does occur even in healthcare AI, due to ethnicity and age differences. An AI is only as trustworthy as the data that has been fed to train the AI. Since healthcare facilities require the usage of phenotype and genotype information to treat patients, the AI can sometimes lead to false diagnosis and then render treatment that is overall ineffective for a particular subpopulation. For instance, AI that treats skin cancer primarily focused on Caucasian origin can provide ineffective modes of treatment for patients with the same ailment but of Afro-American origins. Another problem with AI in the healthcare sector can be seen in case of deployment of AI. An AI that is developed for resource-rich settings if deployed in remote areas will not provide the best results where economic inequality plays a vital factor.
The implications of AI on health systems can be varied. Some of them are as follows:
-
Lack of a clear standard of fairness
A consumer-oriented study suggested that on searching ‘CEO’ on a popular search engine, only 11% of the results showed female CEOs when the resident female CEOs of big corporations was 20% in America alone. The problem with the AIs search results mirrors the inequalities embedded in society. Bias in AI creeps up from the repeated trends that it notices while crunching data. This is where human intervention is sought to prevent the AI from learning immaterial and discriminatory trends. This process is however easier said than done as there is no defined quantitative metric against which evaluation can be done. Also, even with human oversight, the evaluation is entirely qualitative depending upon the biases of the reviewer.
-
Lack of contextual specificity
Health Systems are customized to suit the various economic and geopolitical issues of a population and subpopulation. Different lifestyles bring in different genetic endowments and economic imbalance. It will be wrong and to assume that a ‘generally applicable’ AI can uniformly provide solutions across all classes and categories. In the case of underrepresented groups, the data available shall be insufficient for proper diagnosis and shall lead to incorrect treatment.
-
The black-box nature of deep learning
The most important and pertinent problem with AI is that it is a black box structure. The most capable neural chip structures are notoriously opaque in the sense that it is difficult to identify how exactly an AI arrived at that particular result. In practice, multiple layers of numbers are crunched to create a diagnostic report or category. The process is important to healthcare sectors since the results yielded are powerful, but the nature of hiding or making inaccessible the procedure of identification poses problems when it comes to making rectifications for AI bias. Since it is difficult to pinpoint the source of bias, the task to relieve the bias becomes tiresome and humongous. Regarding this, the data scientists and the healthcare workers, as well as the patients, have a right to know the process of identification of the procedure ultimately chosen by an AI.
Actions are undertaken to counter the risk of AI bias in the health system
To deliver a positive and fruitful experience, policy intervention needs to be adopted to contribute data during the training of the AI. A proper representation should be made to better the tune the AI to the needs of the societal category it tends to serve. In this regard, it is important to establish the context to which the algorithms shall be aimed at. A generalisation of algorithms will continue to display biased behaviour. It should be noted that if bias is present in the society, there will be the presence of bias in the data that the AI crunches to identify the search results. Simply masking the effect of data bias in some algorithms will not yield the desired result.
It is also to be noted that experts need to stop treating AI bias as a technical issue only. The focus should be made to identify that proper representation is made. The inequities in the data that generate the algorithm bias are the same inequity that decides which socio-economic class shall bear the brunt of the diseases and which shall receive state of the art treatment. Hence, the teams should concentrate on the collective efforts of policymakers as well as engineers to drive away bias from algorithms. A multidisciplinary approach will benefit all communities equally and will ensure the basic democratic right of equal representation guaranteed across all constitutions.
It is also to be noted that for a non-expert, it is certainly difficult to elucidate or understand the intricacies of a deep learning algorithm. To ascertain the necessary transparency, the algorithmic inputs, its parameters, outputs and data crunching metrics will need to be made available to the public so that proper feedback on the information used to reach an outcome can be gathered. The publication of these datasets will also help in controlling the exposure to a particular kind of data or in employing the qualitative analysis of the AI.
Solutions
The most prominent methods to solve the growing tendencies of AI bias are:
- Narrowing down the scope of utility of the AI is a primary method to counter the effects of AI bias. The developers need to realise that no AI can be called generally applicable. No AI should be made generally applicable if one wants to ensure that discriminatory actions against underrepresented communities are minimised.
- The training data should be curated in such a manner that it allows the representation of a broader spectrum on the issue rather than marginalising the opinions of the few. Policymakers and stakeholders can run open-end feedback loops to properly identify and subsequently rectify the biased metrics by taking in regular inputs from the general mass.
- Stringent laws should be made to compel the stakeholders into taking definitive actions to rectify Ai bias. In this regard, the AI could be subjected to third party regulatory checks apart from the ones issued by the government and company regulatory bodies.
Difficulties in resolving AI bias
The repetitive cycle of identifying and rectifying is easier said than done. Constant checking and improvement might be the cause for rendering the AI useless at a crucial time, thus defeating the very purpose of acquiring the AI. It should also be noted that during the construction of the model dataset, it is difficult to identify the impact of the data and the choices entered by the user. Thus, retroactively identifying the source of the problem becomes cumbersome and often becomes impossible.
In case of an AI, the data entered is broadly tested and identified into two streams- data that is used for training, and the other set for testing. If a bias is present, it is present in both these sets of data. Added to that, the data multiplication also causes the multiplication of the instances of bias in the dataset, thus making it difficult to remove these faulty instances.
AI ethics coalition condemn criminality prediction algorithms
The Coalition for Critical Technology has repeatedly bashed the criminality prediction algorithms, stating that scholars should stop making such algorithms. Countless instances have indicated that algorithms aimed at identifying criminal patterns are harshly biased towards minorities. The efficacy of face recognition and criminal policing algorithms are highly questionable and adds to the discrepancies in the identification systems. The coalition argues that sometimes the entire notion of criminality is directed against a particular race. Although researchers time and again claim neutrality in the training and testing datasets, no neutrality exists.
Need of the hour: ethics as the core of AI
It cannot be properly stressed enough, but the need for an AI having a metric in ethics evaluation is a must for AIs across all sectors. The independent, as well as inherent evaluation process, needs to identify and then qualitatively compare the data against ethical standards. For these to be achieved, the algorithm needs to encapsulate the essence of triggers that sparks the need for proper recognition statuses and minority representation along with gender-based representation. Narrowing down AI based on socio-economic and ethnic grounds and adding wider variety will help the AI in ‘learning’ the difference between unfeeling result-oriented searches and results that cater to an individual taking into consideration their likes and dislikes.
Conclusion
It is visible that the algorithm bias in the systems around us is an increasing menace and calls for swift actions. India on this front needs to develop better and more insightful laws. As a country that has subpopulations rich with cultural diversity, AI bias will cause significant discriminatory behaviour if not tethered by law. Apart from enacting appropriate legislation, proper regulatory bodies need to be set up on an expedited basis to counter the ill-effects of bias as and when reported. Further, the law could seek to make the stakeholders of the AI liable for the actions and the continued bias of the AI. Stricter and more tangible laws along with proper human intervention and oversight are the only way forward against AI bias.
References
- https://www.brookings.edu/research/algorithmic-bias-detection-and-mitigation-best-practices-and-policies-to-reduce-consumer-harms/
- https://www.vox.com/recode/2020/2/18/21121286/algorithms-bias-discrimination-facial-recognition-transparency
- https://towardsdatascience.com/how-are-algorithms-biased-8449406aaa83
- https://www.forbes.com/sites/cognitiveworld/2020/02/07/biased-algorithms/?sh=4f87ff5476fc
- http://interactions.acm.org/archive/view/november-december-2018/assessing-and-addressing-algorithmic-bias-in-practice
LawSikho has created a telegram group for exchanging legal knowledge, referrals and various opportunities. You can click on this link and join:
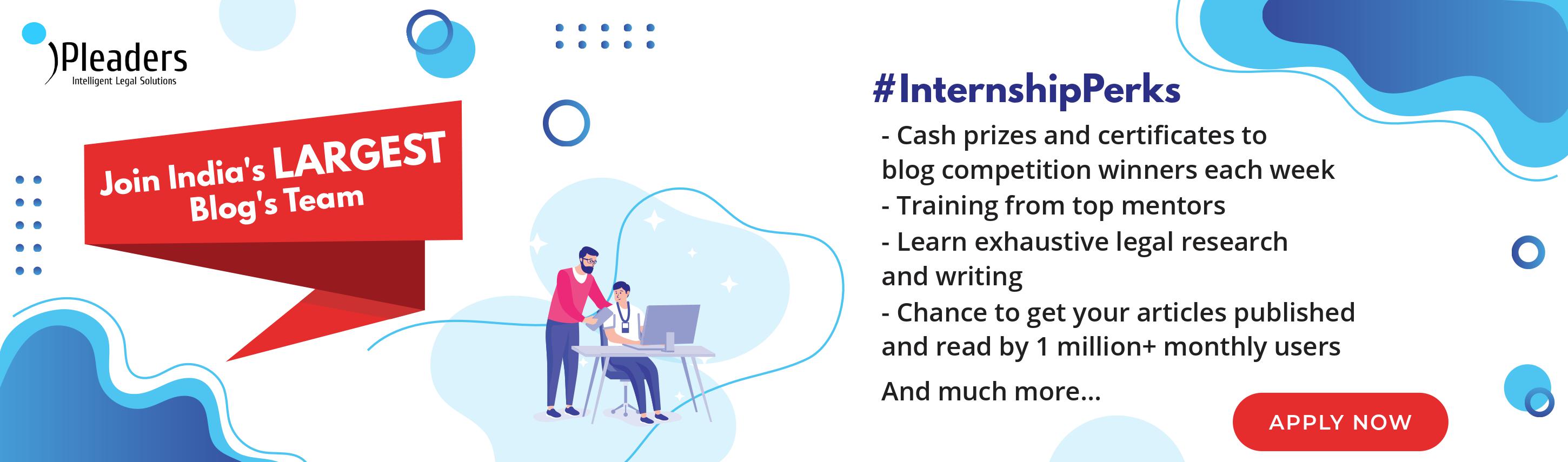