This article has been written by Aathira Ajith pursuing a Diploma in Corporate Law & Practice: Transactions, Governance and Disputes course from LawSikho.
This article has been edited and published by Shashwat Kaushik.
Introduction
The inclusion of AI in the financial sector made the whole process very dynamic, effective, and cost-effective; it expanded the dimension for extending financial services to one and all with ease. Fast change is, however, an uphill task that faces a challenge in the legal arena, especially problems relating to regulating the AML model. What makes the crossroads of AI and company law so critical is the AI technologies is used for
- Risk assessment
- Transaction monitoring
- Customer due diligence.
Below are outlined opinions associated with the point of view of relevant law, be it in relation to AML requirements or the potential impact it could cause in the frame of company law.
From algorithmic trading to the development of something as hard as customer service chatbots, already, AI technologies are something integral in many processes related to finance. Efficiency and speed built into these systems can process a very large volume of data and make real-time decisions.
On the other hand, this gives rise to another serious problem:
- accountability,
- transparency
- icy ethical concerns.
- the potential for a data breach
- complexity in verifying compliance with prevailing laws—milestone concerns that will always have to do with AI algorithms.
The matter does thus call for great care and attention.
It, therefore, follows that a successful system taking into account provisions of detection and prevention of activity of money laundering in any financial institution should be built within the framework of AML regulation.
The risk-based approach, according to the Financial Action Task Force, is the definition of institutions defining their vulnerabilities and taking proper steps to correct the same in order to reduce the identified risk. By developing the capability for higher automation in transaction monitoring with the advancement of AI, flags can be raised on suspicious activities. Nonetheless, such applications of AI need to be done as prescribed by the legislators and policymakers in a manner that would not have such institutions fall into the default of privacy requirements or do acts that might be seen to be discriminatory.
The legal landscape associated with the use of AI in finance is quite complex and dynamic. Regulators are only starting to shape new frameworks that would recognise unique challenges associated with developed AI technologies, for instance by issuing guidance on best practices in the use of artificial intelligence in investment—going as far as requiring emphasis on transparency and preventing misguiding of investors. The FTC went further to the extent of noting that applications of AI had to be non-discriminatory and accountable.
With AI starting to creep into working practices, the discussion now is on how that impacts internal governance and compliance within financial institutions. It would be the case, at any one time, an expectation to oversee AI workflows from top management and the board of directors to ensure that risks are put under check. This also implies the capacity for monitoring and evaluating AI setups continuously because biases are inherent in such systems to conform to the AML requirements.
Overview of artificial intelligence applications in finance
It has even reached customer service automation and fraud detection. AI systems analyse big data, which enables such institutions to identify the kind of patterns and make decisions at a speed not seen before. The robo-advisors are going to be using the same AI algorithms for issuing investment advice on a client-specific basis to know the level of aversion to risk that their individual clients have and the actual market conditions.
Benefits and drawbacks of AI utilisation
Benefits of AI utilisation in finance
- Efficiency: AI can automate repetitive tasks, such as data entry and processing, freeing up human workers to focus on more strategic and value-added activities. This can lead to significant improvements in efficiency and productivity.
- Reduced operational costs: By automating tasks and improving efficiency, AI can help financial institutions reduce their operational costs. This can free up resources that can be invested in other areas, such as innovation and customer service.
- Improved customer experience: AI can be used to provide customers with a more personalised and convenient experience. For example, AI-powered chatbots can be used to answer customer questions and provide support 24 hours a day, 7 days a week. AI can also be used to analyse customer data to identify their needs and preferences, which can help financial institutions tailor their products and services accordingly.
Drawbacks of AI utilisation in finance
- Personal data protection: The use of AI in finance raises concerns about personal data protection. Financial institutions collect and store large amounts of customer data, which can be used to train AI models. However, if this data is not properly protected, it could be used for malicious purposes, such as identity theft or fraud.
- Algorithmic bias: AI algorithms can be biased, which can lead to unfair or discriminatory outcomes. For example, an AI algorithm that is used to make lending decisions could be biased against certain groups of people, such as minorities or low-income individuals.
- Transparency in decision-making: The use of AI in finance can make it difficult to understand how decisions are made. This lack of transparency can make it difficult to identify and address any biases or errors in the decision-making process.
Legal framework for AI in finance: existing regulations and directives
The legal environment remains rather in evolution, referring to the domain of finance. There are several regulatory bodies, such as the SEC and FTC, that have created guidelines related to the use of AI technologies. Such principles, including transparency, accountability, and non-discrimination, are incorporated into the guidelines and guide us while using AI applications.
Role of the regulatory bodies
The last among these would be the considerable role the regulatory bodies would play in the governance of applications in financial services using the AIs. They will play their part to ensure that the financial institutions abide by the developed laws and agree to substantial change in the integration of AIs in these services. They would further monitor and conclude whether the use of AIs is made pertinent to the AML regulation and any kind of risk associated after implementation of the AIs by algorithms in decision-making about financial affairs.
AI for ensuring AML compliance
Real-time monitoring is one of the key functional capabilities of AI when it comes to AML compliance. In the past, this often involved huge amounts of manpower, which tends to be unwieldy and not quite agile enough for the ever-increasing threat. On the contrary, tracking voluminous transaction data in a fraction of a second, the AI systems trace patterns and track all through to bring forth suspicious activities for further digging. This will further allow financial organisations to track in real time any emerging risks well in advance, providing them the capability to act at the right time in the face of any illicit financial behaviour. Moreover, it is said that AI with complex patterns and anomalies can play a big role in non-financial crime against these financial crimes. Machine learning algorithms offer the advantage of learning from the historical data approaches developed to detect money laundering. Such a proactive system allows detection of those risks that would pass the check of the porous traditional systems. Most rely on static rules, which do not evolve as fast as the development of criminal tactics. Indeed, due diligence on customers can be very effective with AI technologies. Artificially intelligent technologies can help automatically judge the risks accompanying a customer based on his transactional behaviour and geographical location, among other things. In fact, such an approach helps in focused risk-mitigating strategies wherein resources are directed specifically towards high-risk customers and transactions. Other than the one-time assessment of risk, AI could also be used for ongoing monitoring of customer behaviour. Real-time analysis of transactions means an AI system can pick up changes in the latter behaviour, which could potentially bring out evidence of money-laundering activity if ideally the changes belonged to a pattern that was established prior to it. This enterprise shall require vigilance to go on with day-to-day oppression through AML regulation and make it overtake all the risks newly developed. With evolving AI technologies, one of the most obvious advancements will be from machine learning algorithms, which will certainly provide greater efficiencies in complying with AML. In this regard, such algorithms should thus enable financial institutions to improve their detection capabilities to correspondingly match new methods of money laundering and generally improve overall compliance frameworks. In this regard, future AML compliance will be even more based on artificial intelligence-based solutions; it is highly likely it would prove to be an inescapable factor for financial institutions in fighting financial crime. Big data analytics is yet another emergent role AI acquires in the field of AML compliance. It is only through accessing vast volumes of structured and unstructured data that any financial institution will have more light thrown on customer behaviours and trends in transactions. In the process, an organisation is likely to establish suspicious money laundering activities and thus apply appropriate risk mitigation measures.
Importance of AML compliance
The significance of anti-money laundering (AML) compliance extends far beyond mere adherence to legal obligations. It serves as a vital safeguard against illicit financial activities that threaten the integrity of financial systems worldwide. Without robust AML regulations in place, criminal elements could exploit vulnerabilities to launder ill-gotten gains, finance terrorism, and undermine economic stability.
At the core of AML compliance lies the Financial Action Task Force (FATF), an intergovernmental body that sets global standards and recommendations for combating money laundering and terrorist financing. FATF’s guidance provides a comprehensive framework upon which financial institutions can develop and implement effective AML programs.
One of the key principles emphasised by FATF is the risk-based approach. This approach acknowledges that not all customers and transactions pose the same level of risk. By identifying and assessing their vulnerabilities, financial institutions can allocate resources and implement controls accordingly. This risk-based approach allows for more targeted and efficient use of AML resources, ensuring that high-risk activities receive the necessary scrutiny.
The onus of AML compliance ultimately rests with financial institutions themselves. They are responsible for developing and implementing robust AML programs that comply with applicable laws and regulations. These programs typically involve a combination of policies, procedures, and technologies designed to detect and prevent money laundering and terrorist financing.
Effective AML compliance requires a multi-faceted approach that involves collaboration among various stakeholders. Financial institutions must work closely with law enforcement agencies, regulators, and other relevant authorities to share information and best practices. International cooperation is also crucial, as money laundering and terrorist financing often transcend national borders.
Furthermore, AML compliance is not a one-time exercise. It is an ongoing process that requires continuous monitoring and adaptation to evolving threats and regulatory changes. Financial institutions must invest in ongoing training and education for their staff to ensure they are equipped with the knowledge and skills necessary to effectively combat money laundering and terrorist financing.
By embracing AML compliance, financial institutions can contribute to the global fight against financial crime, protect their customers and stakeholders, and maintain the integrity of the financial system.
Role of AI in enhancing AML operations
Artificial intelligence (AI) is revolutionising the way businesses operate, and the anti-money laundering (AML) industry is no exception. AI-powered solutions are transforming AML operations by enhancing efficiency, accuracy, and risk detection capabilities.
One of the critical roles of AI in AML is automating routine and repetitive tasks. AI algorithms can process large volumes of transaction data, identify suspicious patterns, and generate alerts for further investigation. This automation frees up AML analysts to focus on more complex and high-risk cases, maximising their productivity and effectiveness.
AI also plays a vital role in enhancing the accuracy of AML operations. Natural language processing (NLP) helps extract and analyse information from unstructured data sources such as emails, reports, and social media posts. This enables AML analysts to gain a deeper understanding of customer behaviour and identify potential risks that may have been missed using traditional methods.
Furthermore, AI enhances risk detection capabilities by leveraging machine learning algorithms. These algorithms can identify unusual patterns and anomalies in transaction data, allowing AML analysts to prioritise high-risk cases for further investigation. AI-powered risk scoring models utilise historical data to assign risk levels to customers, enabling AML teams to allocate resources more efficiently.
AI’s ability to process large volumes of data in real-time enables AML professionals to monitor transactions continuously. This real-time monitoring significantly reduces the risk of financial crimes and allows for timely intervention to prevent suspicious activities.
Regulatory compliance is another critical area where AI can assist AML professionals. AI-powered solutions can help organisations meet regulatory requirements by identifying and reporting suspicious transactions. They also provide audit trails and documentation to support compliance efforts.
Board and management functions
One such is that the financial corporations must review corporate governance principles on AI in financial services; these are developed at the strategic levels by the board of directors and senior management with respect to AI monitoring implementation and determining requirements of risk management. Such requirements are drafted by outlining policies and closely observing adherence to ethical use and AML policies.
Therefore, the banks should have in place risk management processes that will ensure monitoring and, at the same time, the development of frameworks for assessing AI systems, identification of bias, and mitigation of bias, where applicable, also to meet regulatory and legal requirements.
Countering AI bias and discrimination: a critical step for financial institutions
In the rapidly evolving era of artificial intelligence (AI), financial institutions face a significant challenge in addressing AI bias and discrimination. This ethical concern calls for proactive measures to ensure objective decision-making and maintain trust in the financial system.
AI algorithms, designed to process vast amounts of data and make decisions, can inherit the biases present in the training data or the underlying models. These biases can result in unfair or discriminatory outcomes, affecting individuals’ access to financial services, creditworthiness assessments, and investment opportunities.
Financial institutions must take a proactive approach to identifying and mitigating AI bias. This involves conducting thorough risk assessments, regularly auditing AI systems, and implementing robust governance frameworks. Additionally, fostering a culture of diversity and inclusion within AI development teams is crucial to ensuring a broad range of perspectives and experiences are considered in the design and implementation of AI systems.
As the adoption of AI deepens in the finance sector, regulatory frameworks specifically tailored to mitigate the unique risks posed by AI technologies become imperative. These frameworks should provide clear guidelines on the responsible use of AI, including principles for data governance, model validation, and accountability mechanisms.
Business news channels play a vital role in raising awareness about AI bias and discrimination. Reporting on cases of bias and the efforts of financial institutions to address these issues can help hold organisations accountable and drive positive change. By embedding ethical considerations into their practices, financial organisations can leverage AI’s potential to enhance fairness, transparency, and inclusivity in the financial system.
Addressing AI bias and discrimination is not only an ethical imperative but also a business necessity. Financial institutions that prioritise responsible AI practices will gain a competitive advantage, inspire trust among customers and stakeholders, and pave the way for a more just and equitable financial future.
Conclusion
The inclusion of AI in the world of finance implicates a mixture of implication and opportunity. In this case, AI technologies can promise efficiency and effectiveness in driving AML; however, they are massive viewpoints that raise legal and ethical concerns. The finer detail will have to be walked through by the financial institutions with an appropriate governance framework ensuring conformance to the existing laws with a connotation of potentially mitigating the biases in AI systems. This requires doing so with the infusing of AI into the architectural fabric of the institution in a forward and cautious manner that would not compromise such basic qualities as transparency, accountability, and fairness.
References
- Legal regulation of artificial intelligence in the financial services market: a comparative analysis | SHS Web of Conferences (shs-conferences.org)
- Artificial Intelligence, Finance, and the Law (fordham.edu)
- AI In Financial Services: Legal Risk – Financial Services – Finance and Banking – United States (mondaq.com)
- Legal regulation of artificial intelligence in the financial services market: a comparative analysis (shs-conferences.org)
- Artificial Intelligence, Finance, and the Law” by Tom C.W. Lin (fordham.edu)
- The Current (and Future) State of AI in Legal Finance | New York Law Journal
- Artificial Intelligence, Finance, and the Law – The Temple 10-Q
- Financial law in the era of artificial intelligence: key topics and themes: Law and Financial Markets Review: Vol 15 , No 3-4 – Get Access (tandfonline.com)
- AML Surveillance: AI’s Impact on Compliance & Risk – Transform FinCrime Operations & Investigations with AI (lucinity.com)
- AI revolution in AML Unlocking the future of financial compliance (ixsight.com)
- Enhance AML Compliance with AI-native Solutions (flagright.com)
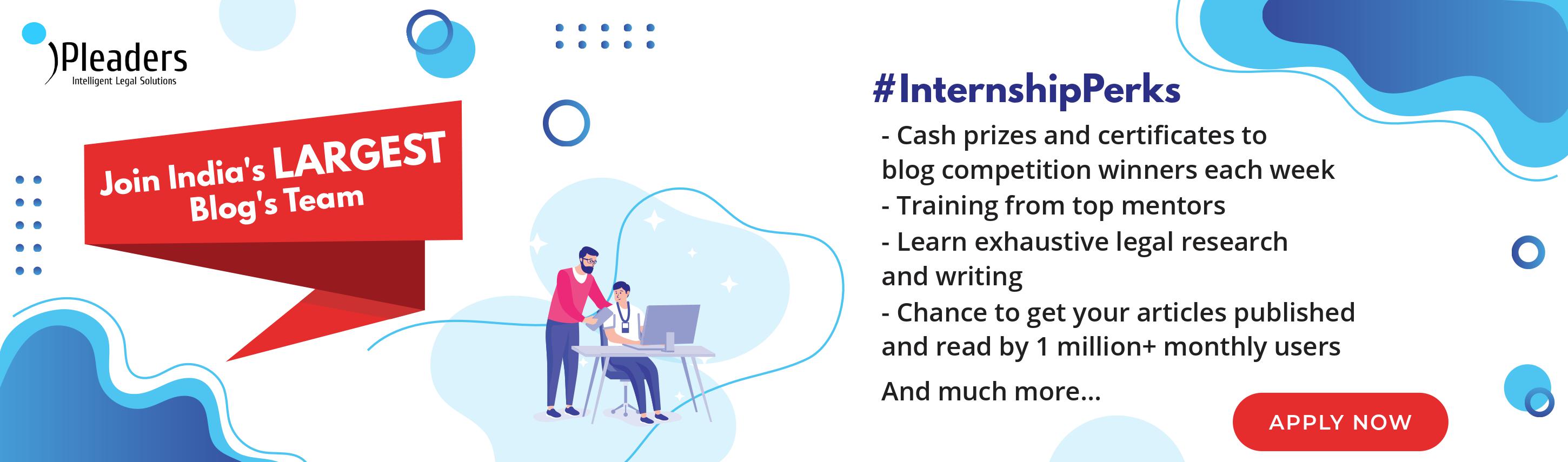