This Article is written by Utkarsh Singh, from Amity Law School, Noida. This is an exhaustive article that deals with Artificial Intelligence outpacing Moore’s Law.
Table of Contents
Introduction
Eight years back an AI calculation figured out how to recognize a feline and it shocked the world. A couple of years after the fact artificial intelligence could precisely interpret dialects and take down the best players on the planet. Now, artificial intelligence has started to do great work at difficult multiplayer video games like Dota 2 and Starcraft and the games which have subtitles like poker. Artificial intelligence, it would be seen, is getting upgraded fast. Yet, how quick is quick, and what’s driving the pace?
While better personal computer chips are vital, Artificial Intelligence research association OpenAI figures we should gauge the pace of progress of genuine artificial intelligence calculations as well. In a blog entry and paper— composed by OpenAI’s Danny Hernandez and Tom Brown and distributed on the arXiv, an open storehouse for pre-print (or not-yet-peer-surveyed) examines—the scientists state they’ve started following another measure for AI productivity (that is, accomplishing more with less).
Using this step, they show artificial intelligence has been getting better at the wicked pace. To evaluate progress, the specialists picked a benchmark picture acknowledgement calculation (AlexNet) from 2012 and followed how much registering power more current calculations took to coordinate or surpass the benchmark. They discovered algorithmic proficiency multiplied at regular intervals, outpacing Moore’s Law. Picture acknowledgement AI in 2019 required multiple times less processing capacity to accomplish AlexNet-like execution. In spite of the fact that there is less information focused, the creators found significantly quicker paces of progress over shorter periods in other famous capacities, for example, interpretation and game-playing.
The Transformer calculation, for instance, took multiple times less processing capacity to outperform the seq2seq calculation at English to French interpretation three years after the fact. DeepMind’s AlphaZero required multiple times less processing to coordinate AlphaGoZero at the round of Go just a year later. What’s more, OpenaAI Five Rerun utilized multiple times less figuring capacity to overwhelm the titleholder beating OpenAI Five at Dota 2 only three months after the fact.
What is Moore’s law
Moore’s law, a forecast made by American engineer Gordon Moore in 1965 that the number of transistors per silicon chip twice each year. For an uncommon issue of the diary electronics, Moore was approached to anticipate advancements throughout the following decade. Seeing that the absolute number of segments in these circuits had generally multiplied every year, he cheerfully extrapolated this yearly multiplying to the following decade, assessing that microcircuits of 1975 would contain a shocking 65,000 parts for each chip. In 1975, as the pace of development started to slow, Moore reexamined his time span to two years.
His reconsidered law was somewhat sceptical; over about 50 years from 1961, the number of transistors multiplied roughly like clockwork. Consequently, magazines routinely alluded to Moore’s law as if it were unyielding an innovative law with the confirmation of Newton’s laws of motion. What made this sensational blast in-circuit unpredictability potential was the consistently contracting size of transistors throughout the decades. Estimated in millimetres in the late 1940s, the components of a regular transistor in the mid-2010s were all the more normally communicated in many nanometres (a nanometre being one-billionth of a meter) a decrease factor of more than 100,000.
Transistor highlights estimating not exactly a micron (a micrometre, or one-millionth of a meter) were accomplished during the 1980s when dynamic irregular access memory (DRAM) chips started offering megabyte stockpiling limits. At the beginning of the 21st century, these highlights moved toward 0.1 microns over, which permitted the production of gigabyte memory chips and microchips that work at gigahertz frequencies. Moore’s law proceeded into the second decade of the 21st century with the presentation of three-dimensional transistors that were several nanometres in size.
A Moore’s Law for machine learning
Why track algorithmic productivity? The creators state that three information sources drive progress in Artificial Intelligence: accessible registering force, information, and algorithmic development. Registering power is simpler to follow, yet enhancements in calculations are more dangerous. Is there a sort of algorithmic Moore’s Law in AI? Possibly. In any case, there is insufficient data to state yet, as per the creators. Their work just incorporates some information focuses (the first Moore’s Law diagram also had not many perceptions).
So any extrapolation is simply theoretical. Additionally, the paper centres around only a bunch of well-known abilities and top projects. It’s not satisfactory if the watched patterns can be summed up more broadly. All things considered, the creators state the measure may really disparage progress, to some degree since it conceals the underlying jump from unreasonable to viable. The registering power it would have taken to arrive at a benchmark’s underlying capacity state, AlexNet in picture acknowledgement for earlier methodologies would have been so enormous as to be unrealistic.
The proficiency additions to essentially go from zero to one, at that point, would be faltering however aren’t represented here. The creators additionally call attention to other existing measures for algorithmic improvement can be valuable relying upon what you’re planning to realize. In general, the state, following various measures—incorporating those in equipment—can portray progress and help figure out where future exertion and venture will be best.
Why is Moore’s Law dying
As Chien-Ping Lu from NovuMind Inc so concisely put it in his paper:
The defining moment occurred in 2005, when the transistors while proceeding to twofold in numbers, were neither quicker nor more vitally effective at indistinguishable rates from previously.
Here’s the reason: The 21st century is the point at which the gadgets business was tied in with carrying the tech to the individuals. Organizations blasted, Samsung and Qualcomm being models for cell phones and processors separately. In any case, these days, rivals in the tech business are a lot genuine! All things considered, the equipment given by an organization needed to convey its inheritance: it needed to meet the specs, give better client involvement in each new form, and could in this manner not be forcefully altered to spare vitality.
There was a breakdown of the Dennard scaling perspective, so there has from that point on been a deviation from Moore’s law’s pattern. Enter Artificial Intelligence, and how it fixes the circumstance.
The future of AI
It’s important the investigation centres around profound learning calculations, the prevailing AI approach right now. Regardless of whether profound learning keeps on gaining such sensational ground is a wellspring of discussion in the AI people group. A portion of the field’s top specialists question profound learning’s drawn-out potential to understand the field’s greatest difficulties. In a previous paper, OpenAI demonstrated the most recent title text snatching AIs require a fairly stunning measure of registering capacity to prepare, and that the necessary assets are developing at a torrid pace.
Though development in the measure of figuring power utilized by AI programs preceding 2012 to a great extent followed Moore’s Law, the registering power utilized by AI calculations since 2012 has been growing multiple times quicker than Moore’s Law. This is the reason OpenAI is keen on the following advancement. In the event that AI calculations are getting increasingly costly to prepare, for instance, it’s critical to expand subsidizing to scholarly specialists so they can stay aware of private endeavours. What’s more, if effectiveness patterns demonstrate predictably, it’ll be simpler to foresee future expenses and plan venture appropriately. Regardless of whether progress proceeds unabated, Moore’s-Law-like for quite a long time to come or before long reaches a stopping point is not yet clear. Be that as it may, as the writers compose, if these patterns do proceed into the future, AI will get unmistakably progressively ground-breaking still, and perhaps sooner than we might suspect.
How A.I. is revamping Moore’s Law
We as whole expertise the media and the film business are overhyping AI with androids and over-canny frameworks. Some PC pioneers, Alan Turing (you might need to watch The Imitation Game to welcome the legend he is) at the bleeding edge, set off on ventures with the end goal of making machines that think. Turing, in any case, realized this would be horrifyingly troublesome, and in 1950 proposed:
Rather than attempting to deliver a program to recreate the grown-up mind, why not rather attempt to create one which reenacts the youngster? On the off chance that this was, at that point exposed to a proper course of training, one would get the grown-up cerebrum.
This thought developed to turn out to be Deep Learning. Quick forward to 2018: we have as yet assembling, enormous measures of information. We have been growing an ever-increasing number of cutting edge calculations (Generative Adversarial Networks and Capsule Networks remain as solid models.) But do we have the equipment to crunch every one of those estimations inside a sensible time? Furthermore, on the off chance that we do, should it be possible without having each one of those GPUs cause another dangerous atmospheric deviation all alone by actually warming up from all the preparing?
In that spot, Artificial Intelligence is forcing an imperative: keep the force consistent or decline it, however, increment execution doesn’t sound somewhat natural to some scaling rule we have quite recently observed? Precisely, by compelling the tech business to think of new processors which can perform more computations per unit time, while keeping up power utilization and cost, Artificial Intelligence is forcing Dennard Scaling once more, and henceforth constraining Moore’s Law back to life!
Hans A. Gunnoo is a Data Scientist, having started his profession in Electronic Engineering and later represented considerable authority in Machine Learning. Likewise makes commitments to Open-Source AI ventures and sites about the most recent patterns in the information science field in his spare time.
Artificial Intelligence Outpacing Moore’s law
The Stanford report, delivered in an organization with McKinsey and Company, Google, PwC, OpenAI, Genpact and AI 21 Labs, found that AI computational force is quickening quicker than conventional processor advancement. “Preceding 2012, AI results firmly followed Moore’s Law, with figures multiplying at regular intervals.,” the report said. “Post-2012, the register has been multiplying every 3.4 months.” The investigation took a gander at how AI calculations have improved after some time, by following the advancement of the ImageNet picture ID program.
Given that picture arrangement strategies are to a great extent dependent on directed AI procedures, the report’s creators saw to what extent it takes to prepare an AI model and related costs, which they said speaks to an estimation of the development of AI improvement foundation, reflecting advances in programming and equipment. Their examination found that more than a year and a half, the time required to prepare a system on cloud foundation for regulated picture acknowledgement tumbled from around three hours in October 2017 to around 88 seconds in July 2019.
The report noticed that information on ImageNet preparing time on private cloud cases was in accordance with the open cloud AI preparing time enhancements. The report’s creators utilized the ResNet picture order model to evaluate to what extent it takes calculations to accomplish a significant level of precision. In October 2017, 13 days of preparing time were required to arrive at simply above 93% precision. The report found that the preparation of an AI-based picture arrangement of more than 13 days to accomplish 93% exactness would have cost about $2,323 in 2017.
The investigation announced that the most recent benchmark accessible on Stanford DAWNBench, utilizing a cloud TPU on GCP to run the ResNet model to accomplish picture order precision somewhat above 93% exactness, cost simply over $12 in September 2018. The report likewise investigated how far PC vision had advanced, taking a gander at creative calculations that push the constraints of programmed movement understanding, which can perceive human activities and exercises from recordings utilizing the ActivityNet Challenge.
One of the errands in this test, called Temporal Activity Localisation, utilizes long video successions that delineate more than one action, and the calculation is approached to locate a given movement. Today, calculations can precisely perceive many complex human exercises continuously, yet the report found significantly more work is required.
“In the wake of sorting out the International Activity Recognition Challenge (ActivityNet) throughout the previous four years, we see that more examination is expected to create techniques that can dependably segregate exercises, which include fine-grained movements or potentially inconspicuous examples moving signals, items and human-object corporations,” said Bernard Ghanem, a partner teacher of electrical designing at King Abdullah University of Science and Technology, in the report. “Looking forward, we foresee the next generation of algorithms to be one that accentuates learning without the need for excessively large manually curated data. In this scenario, benchmarks and competitions will remain a cornerstone to track progress in this self-learning domain.”
Conclusion
Today, the amount of data that is generated, by both humans and machines, far outpaces humans’ ability to absorb, interpret, and make complex decisions based on that data. Artificial intelligence forms the basis for all computer learning and is the future of all complex decision making. As an example, most humans can figure out how to not lose at tic-tac-toe (noughts and crosses), even though there are 255,168 unique moves, of which 46,080 ends in a draw. Far fewer folks would be considered grand champions of checkers, with more than 500 x 1018, or 500 quintillions, different potential moves. Computers are extremely efficient at calculating these combinations and permutations to arrive at the best decision. AI (and its logical evolution of machine learning) and deep learning are the foundational future of business decision making.
References
LawSikho has created a telegram group for exchanging legal knowledge, referrals and various opportunities. You can click on this link and join:
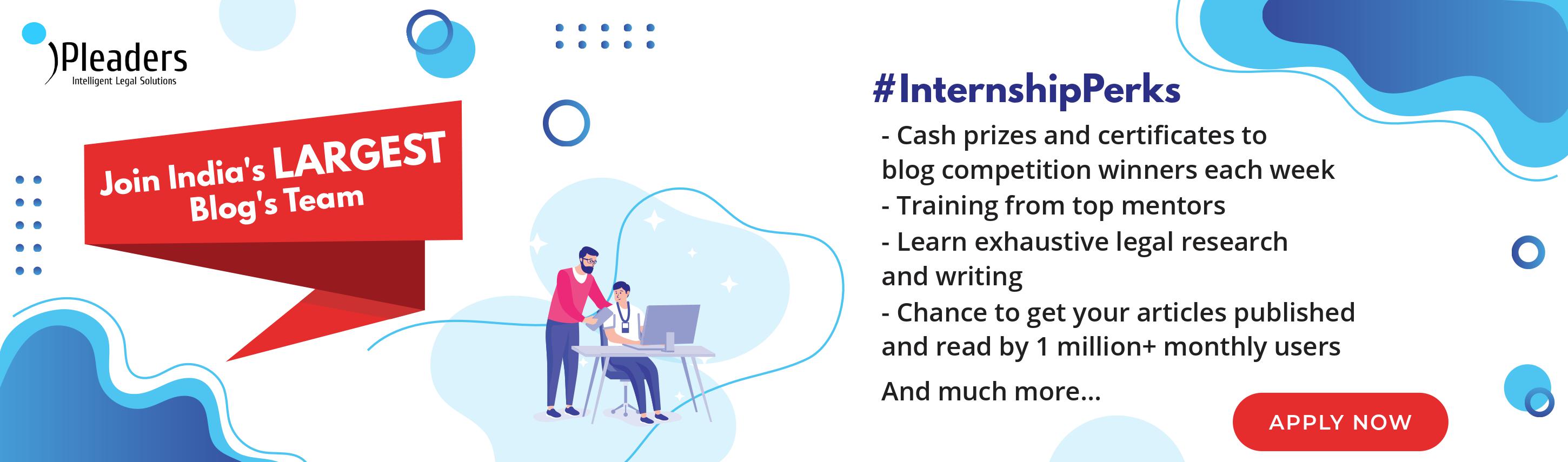