This article has been written by Ashapurna Roy pursuing a Startup Generalist & Virtual Assistant Training Program from Skill Arbitrage.
This article has been edited and published by Shashwat Kaushik.
Table of Contents
Machine learning : an overview
The amount of time it takes for the Uber vehicle to reach your destination, the automated chatbots of the plethora of service companies, the advertisements made-to-order just for you, and Netflix, YouTube, or Instagram content recommendations are customised to your preferences. All that and more are feasible today only because of machine learning.
In fact, machine learning, in its various forms and in association with other General-Purpose Technologies (GPTs), has become so ubiquitous that modern day life is quite unimaginable without it. Yet most of us, the everyday patrons of machine learning, are unaware of what this technology entails. In this article, we shall aim to answer that question as well as unravel the unique opportunities that machine learning presents, in particular in sales.
It was through a seemingly innocuous game of Checkers that the bedrock of machine learning was laid. Arthur Samuel, an American computer scientist and pioneer in the field of artificial intelligence, created the Samuel Checkers Playing Programme, the world’s first self-learning programme to play games. He is also credited with coining the term “machine learning.” Through his seminal paper titled “Some studies in machine learning using the game of checkers,” published in 1959, inspired by his seeming defeat at a game of checkers by an IBM computer and his later works, Samuel founded the initial principles of machine learning. He defined machine learning as “the field of study that gives computers the capability to learn without being explicitly programmed.”
Machine learning (ML) can be broadly defined as a discipline of artificial intelligence (AI) that, through the analysis of statistics, data, algorithms, and optimisation actions, identifies patterns, makes accurate predictions, and enables the performance of automated tasks, i.e., without human intervention.
Application in real life
ML algorithms make the assessment of data simpler by making predictions and classifications. Its actions are quicker and have more accuracy than those of humans. ML assists in uncovering patterns often hidden to the human eye. What’s more is that ML can continuously learn from its actions, optimising results for better future performance.
Ease in data assessment can positively impact business decisions such as pricing and marketing strategies, lead generation, and customer segmentation, ideally generating substantial economic value.
ML algorithms that rely on Natural Language Processing (NLP) can be used in Automatic Speech Recognition (ASR) such as those integrated in new-age mobile phones, computer speech recognition, speech-to-text, and automated text translation. Customer support, virtual agents, and messaging chatbots that rely on NLP are paving the way for cost-effective engagement with customers, resolving customer issues, and offering personalised choices to customers.
Among other uses of ML are image and object recognition, automated robotics, fraud detection, the assessment of medical scans and self-driving cars.
Machine learning in sales
Sales are a set of actions undertaken by a company with the aim of generating profit. It involves selling products and services to customers. Sales are an important metric of business growth and, ultimately, revenue creation. Often, though not exhaustively, sales can involve prospecting new clients and leads, catering to customer requirements through the creation, supply, and sale of products and services, account servicing, market evaluation, product demonstrations, closing negotiations, and transactions. However, the traditional approach to sourcing potential customers is not only time and resource consuming but also largely contingent on luck. Sales teams often end up devoting valuable time to leads that fail to convert. R&D teams frequently expend valuable resources on products that miss the mark and fail to sell. Relying merely on human prowess for such tasks is rapidly proving to be redundant. This redundancy has provided the most crucial stimulus for companies to seek reinforcement from machine learning.
Machine learning can enable a constructive understanding of customer behaviour, purchase history, and consumption patterns. Such actionable insights aid sales teams to identify potential leads, increase conversion rates, predict and reduce close times, and create new products to cater to market demand.
The sales process relies heavily on making accurate sales forecasts and using insights. Unsurprisingly, then, companies that use artificial intelligence and machine learning algorithms in their sales process see their lead numbers rise by 50%, their average talk times drop by 60-70%, and their costs reduced by 40-60%. Needless to say, advances in technology have historically been key drivers of enhanced economic efficiency. Machine learning is a transformative tool that can be used to optimise the sales operations of a company.
Ways in which machine learning can be used to improve sales and revenue
Leading the way – lead scoring
Scoring leads is an exasperating process. It involves the analysis of customer data like age, job description, disposable income, and industry, as well as customer behaviour such as spending pattern, responsiveness to emails, site clicks, and so on – to discern consumers with potential for conversion into customers generating high yields for the company.
Machine learning algorithms can process enormous historical data to eliminate phoney leads and dead ends. They can define and identify specific traits of high potential leads. So, instead of manually filtering consumer data or relying on blind luck, gut feelings, or hit-and-miss approaches, sales teams can focus on data driven quality leads with higher conversion-potential from Marketing Qualified Leads (MQL) into Sales Qualified Leads (SQL). Both the time and effort of the sales staff can be optimised by focusing on people who have a higher probability of being converted into paying customers for the business, resulting in faster closing rates and improving sales productivity.
Oracle of the future – sales forecasting
Traditionally, a sales team is required to spend countless hours manually sifting through spreadsheets, customer information, and industry updates to predict the demand for products and services. However, ML predictive analysis tools can be directed towards demand forecasting at exponential speed. Machine learning generates accurate sales predictions based on time-series analysis, regression analysis, and decision trees. Since machine learning can analyse huge data sets, its predictions are much more accurate than those performed by humans.
The algorithm can be used to evaluate buying and consumption patterns, market trends, and historical sales figures to predict future sales and trends. Reliable information about future sales and trends can equip businesses to supervise investment, adapt to market fluctuations, set achievable targets, and make informed decisions. Armed with such data, businesses can be optimally positioned to plan supply, manufacturing, and investment strategies to cater to future demand and circumvent uncertain investments in risky products or selling strategies. This can further improve customer satisfaction, thereby enhancing sales and revenue.
Rolling the dice on price – optimising pricing strategy
Product pricing can be a grey area conventionally founded on trial-and-error methods. Additionally, the multifaceted influence of the internet has galvanised consumers’ ability to effortlessly compare the prices of competitive products. AI and machine learning can analyse factors such as pricing data, operating costs, inventory, competition, promotions, discounts, occasion, and purchase history to compute price elasticity vis-à-vis demand for consumer groups, enabling companies to arrive at optimal pricing for the widest customer base. Optimal pricing tends to be a decisive factor in gaining one’s competitive edge and driving revenue.
Personalised campaigns and customer segmentation
In 2019, JPMorgan Chase partnered with an AI company after its ML tool increased Chase’s email outreach by a historic feat. ML was used to generate more effective marketing copy, i.e., the tool created new email campaigns to attract higher click engagement and email responsiveness based on existing email drives. In its pilot, Chase saw as high as a 450% lift in click-through rates on ads rendered by the ML tool, compared with others in the 50-200% range. As evident, targeted marketing campaigns ensure increased conversion, thereby expanding revenue.
Similarly, ML technologies can process behavioural data such as click patterns and purchase histories to uniquely tailor marketing and pitching campaigns for customers. Personalising a marketing campaign or pitch can realistically address customer pain points and provide a customised experience. Adopting a more customer-centric approach to sales is vital for increasing customer satisfaction while lowering attrition rates. Machine driven analysis can also be used for customer segmentation based on demographics, social media engagement, and other criteria to create targeted outreach campaigns.
Spurning the churning – churn rate and customer lifetime value
Churn rate or rate of attrition, is the percentage of customers that cease to generate revenue for a company (e.g., stop purchasing products or engaging in business activity) within a designated period. Customer lifetime value (CLV or CLTV) is a metric that represents the total net profit a company can expect to generate from a customer throughout their entire relationship. Machine learning models can apply techniques to isolate customer categories at risk of churning, as well as patterns relating to customer disengagement and dissatisfaction (eventually leading to attrition). The information can then be leveraged into interception actions by sales teams, such as by providing proactive engagement and customised incentives, to reduce or even prevent customers from exiting. This allows for maintaining the health of customer relationships and fostering customer loyalty. Strengthening customer satisfaction and CLV is essential for the long term growth of businesses.
Testing the water – sentiment analysis
Natural Language Processing (NLP) algorithms can evaluate texts like social media posts to gauge consumer attitudes towards a product, brand, person, or company. Customers tend to buy from companies they associate the most with or from companies with established goodwill. Targeted campaigns can use sentiment analysis and feedback data to educate customers, build a wider customer-base and improve brand image and credibility. The result is an increase in marketability (of a product, brand, or company) and long-lasting customer patronage, enhancing business productivity and maximising revenue.
Destination automation – mechanisation of routine tasks
An average sales representative’s time is spent more on mundane tasks such as scheduling appointments, responding to emails, systemizing data, and so on. Such tasks are often low-value, costing time and resources for the company. Smart process automation (SPA) and machine learning processes can mechanise routine yet time consuming tasks.
Automation can also rely on NLP to create chatbots and AI virtual assistants that cater to customer grievances, Frequently Asked Questions (FAQs) about various products and services, provide personalised customer service for tasks such as product browsing, providing account statements, updating personal information, and even cross-selling products specially identified with potential for purchase by customers. Amelia, the “cognitive agent” developed by IPsoft, can parse natural language to understand customers’ questions, handling up to 27,000 conversations simultaneously and in multiple languages. Amelia is also programmed to involve a human agent when she can no longer assist.
Automation will create more pockets of time for sales teams to focus on networking, pitching, building customer relations, finalising deals, and innovating new business strategies. This will undoubtedly enhance sales teams’ efficiency, thereby leading to increased sales and revenue generation.
In addition to the above, ML can be used for optimising landing pages, data mining projects, text, speech, and image recognition, predicting cart abandonment, and gaining wider knowledge into customer behaviour, all of which can play a vital role in enhancing sales and maximising revenue.
Conclusion
From that first computer victory over a human at a game of checkers in 1959, to the more recent defeat of grandmaster Hikaru Nakamura by Stockfish in 2021, machine learning has evolved only to become more intelligent. Businesses that have embraced AI and machine learning are already reaping the rewards in terms of an increase in leads and appointments, cost reductions, and call time reductions. Millions more are being saved each year through automation alone. Servion Global Solutions has predicted that by 2025, 95% of customer interactions will be powered by AI.
However, as ML develops, there are important questions, such as those relating to its legal and ethical implications, that still need to be addressed. Machine learning technology can and will transform many jobs in the economy, but automation of entire jobs will be less significant than the reengineering of processes and the reorganisation of tasks. The focus of researchers, as well as managers and entrepreneurs, should, therefore, be not just on automation, but on job redesign. Human intelligence, imagination, and empathy cannot be automated and should be at the core of all human endeavour. Machine learning should therefore serve as a supplemental tool to augment revenue, albeit an important one.
The verdict is therefore out and the unanimous decision is that AI and machine learning are ushering in an era of unprecedented technological advancements. Transforming the business landscape and the roles in it, AI and ML prove the old adage, work smarter, not harder. Industry leaders and decision makers will play crucial roles in determining how well and in what manner these technologies are adapted. Companies harnessing these tools can only stand to gain, provided AI and ML are implemented with a humane approach.
References
- https://www.ibm.com/topics/machine-learning
- https://ischoolonline.berkeley.edu/blog/what-is-machine-learning/
- https://www.leadfuze.com/machine-learning-in-sales/
- https://www.akkio.com/post/machine-learning-for-sales-a-practical-guide
- https://www.zendesk.com/in/sell/features/ai-for-sales/
- https://blog.hubspot.com/sales/sales-forecasting-machine-learning
- https://www.persado.com/press-releases/jpmorgan-chase-announces-five-year-deal-with-persado-for-ai-powered-marketing-capabilities/
- https://hbr.org/2016/06/why-salespeople-need-to-develop-machine-intelligence
- https://www.forbes.com/sites/louiscolumbus/2018/12/26/10-ways-machine-learning-is-revolutionizing-sales/?sh=10a8037b3fd1
- https://sales-mind.ai/blog/machine-learning-in-sales
- https://www.spiceworks.com/tech/artificial-intelligence/articles/what-is-ml/
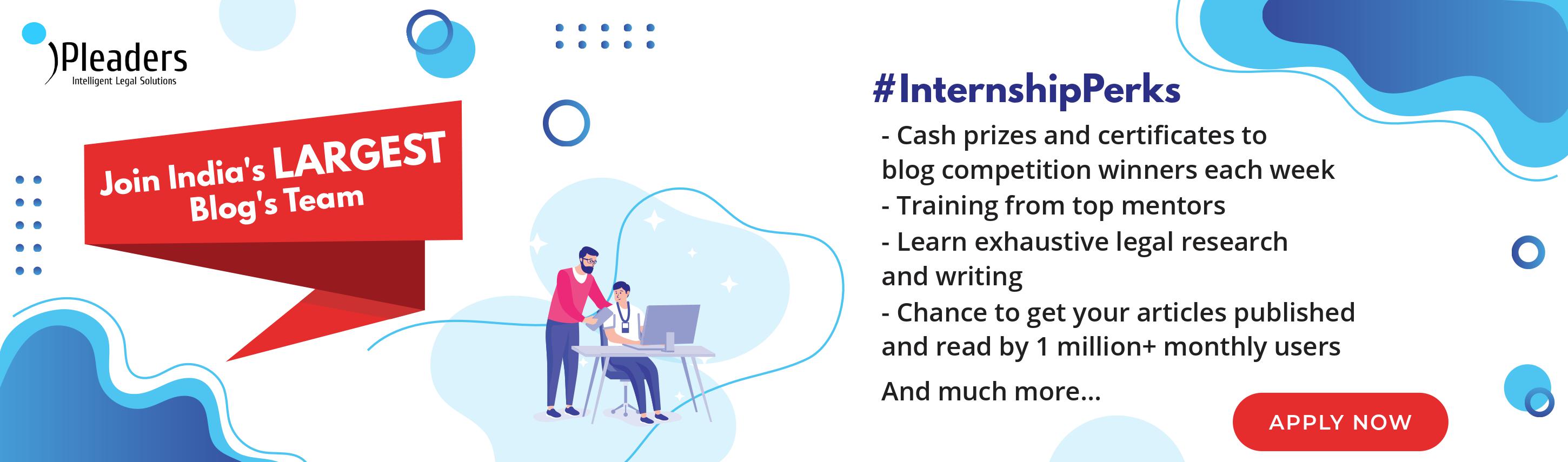