This article has been written by Meghana Urs pursuing a Diploma in Business English Communication for International Professionals and Remote Workers from Skill Arbitrage.
This article has been edited and published by Shashwat Kaushik.
Table of Contents
Introduction
In today’s world of AI, where technology is constantly evolving and enhancing human beings’ experiences for the better than in previous decades, where ancestors or even baby boomers had to work harder to make better decisions, the world of AI has found the solution to this decade old challenge. The power of AI technology has upgraded our lives not just in the field of marketing but in every other sector like healthcare, resource/waste management, education, environmental challenges, and banking, to name a few.
Role of AI in ecommerce
In this world of digital marketing, where shopping online has become easier and the first choice for people living in urban cities and is rapidly spreading to remote towns /villages, AI has enhanced the shopping experience in the retail sector. This has encouraged millennials to Gen Z to become business owners in the field of ecommerce. The role of AI serves as a catalyst for many business owners to have ecommerce shop by seeking effective product recommendations. AI does provide effective solutions by providing end to end quality services to end users. It’s a delight for business owners when the power of data helps them make better decisions, whether its inventory management, cherry picking products of high quality, turn around ratios, logistics or shipments involved. AI has a presence in every sector of ecommerce starting with website design and identifying and providing recommendations for niche products based on which new products that are in demand or have the potential to become in demand are identified. Customers’ experiences have become easier and more trustworthy with the help of intelligent bots and virtual assistants who assist customers with all their queries around the clock.
Usage of data analytics and machine learning for product recommendations
Harnessing the power of machine learning algorithms, AI interprets petabytes of customer data by analysing their shopping behaviours, preferences, purchase history and likes and dislikes, which will help in understanding customer responses and generating product recommendations. This way, it improves the user experience and generates more leads, resulting in sales and overall customer satisfaction. By leveraging AI for overall automation, business owners’ lives get merrier as it enhances refining the recommendation process, optimising marketing, understanding rapidly changing consumer trends and adapting to changes with ease. Ultimately, it helps foster an efficient, customer centric approach to product sales. With the knowledge of understanding the usage of data, businesses can refine their recommendation engines based on the insights to make better strategic decisions, tailor product recommendations and enhance overall end user satisfaction. By harnessing data analytics, the organisation empowers the marketing team by enhancing marketing campaigns, content creations and operational processes. Machine learning helps in predictive analysis, which does enable the business to foresee potential challenges, risks and other new opportunities. This provides a 360 view of end to end product to consumer reviews, enhancing business owners ability to be proactive in their decision making. These data-driven insights enhance business growth and innovation by improving products based on innovation, cost optimization and providing efficient logistics services to stay ahead of competitors. With the use of data analytics and machine learning models, AI plays a pivotal role in revolutionising personalised recommendations by reshaping the way businesses engage with their customers and significantly impacting purchasing decisions. With continuous monitoring of data driven insights using AI, it leverages this to make better analyses of user interactions, allowing for optimal product recommendations, enhancing the customer experience and increasing the likelihood of conversions. This not only boosts sales but also fosters the customer relationship and builds loyalty by demonstrating a deep understanding of their needs and desires. Overall, based on these data, the use of product recommendations enables businesses to deliver precise, customer focused offerings that drive customer engagement, lead generations and revenue generation.
Understanding and analysis of customer behaviour using AI
AI for user behaviour analysis excels at processing and interpretation of user behaviour by tracking the user interaction with the platform. Using data analytics and advanced machine learning models, it can discern patterns, preferences and trends in user interactions on the website. This comprehensive understanding of the pages visited from time to time and the time spent on each page/product helps AI capture the intricate details to create a holistic view of the customer’s interests and their behavioural patterns, which forms the foundation for generating personalised recommendations. AI leverages the power of data to create personalised customer profiles. It’s becoming a driving force and revolutionising the way businesses engage with their audiences. By amalgamating data such as purchase history, search query options, and demographic information, it builds a detailed profile of the user. This information serves as the basis for tailoring recommendations to individual preferences by ensuring the suggested products, services and content align with customer specific needs and desires, thus significantly enhancing the customer experience. One of the other strengths of AI-driven personalisation is the ability to adapt to real time data. As customers navigate to web pages or apps, AI analyses their behaviour instantly and updates the recommendations accordingly. This dynamic approach ensures the customer’s relevance and current interests. This shows how AI extends personalisation across various channels and provides seamless experiences for customer interaction, not limiting it to the website or apps but also to social media or emails. This omnichannel approach ensures consistency in recommendations, enhancing customer engagement and loyalty. The integration of AI in a data driven world involves the personalisation of sophisticated machine learning algorithms that continuously learn and adapt based on user data. Some of the techniques are collaborative filtering, content based filtering and hybrid approaches. This is the backbone for identifying patterns, preferences, and trends and refining the recommendations as the customer engages with the platform over time. Websites and apps can dynamically adjust content, visuals, and layouts to cater to individual needs. This adaptation enhances the user experience, creating a more personalised and engaging interaction.
Approach to predictive analysis for product recommendations
AI employs predictive analysis to anticipate future customer behaviour. By analysing historical data, user preferences, and market dynamics, AI helps businesses understand by predicting the product or service a customer might be interested in and providing timely recommendations even before explicit preferences are expressed. The system can forecast potential preferences and predict which new offerings resonate with the customer. This proactive approach enhances the relevance of suggestions and increases conversions to successful sales, thus improving the quality of services. This not only streamlines the product development process but also ensures the new recommendations are tailored to individual choices. AI facilitates segmentation and targeting by categorising users into clusters based on shared characteristics and behaviours’. This segmentation does allow businesses to identify the right product precisely and suggest relevant recommendations. Users with similar preferences, purchase histories are grouped together, enabling AI to suggest products that have been well received and are in demand within the segment.
Approach of NLP for product recommendations
NLP (natural language processing) is a subset of AI, enhances personalisation by understanding and analysing textual data. AI systems can interpret reviews, product descriptions, and user comments, thus gaining insights into sentiments and preferences. This linguistic analysis contributes to more nuanced and context based aware recommendations, thus offering a deeper understanding of user preferences beyond numerical data. This, in turn, provides customers with confidence to trust the usage of the product and its quality and it builds a trustworthy relationship with many customers, as it’s similar to word by mouth approach in the digital world. NLP enables AI systems to grasp the semantic meaning of words and phrases. This deeper comprehension allows recommendation algorithms to interpret user reviews, feedback about the products. This helps AI offer more personalised recommendations. NLP helps AI understand the context of certain words or phrases being used. It can distinguish between positive, negative or neutral sentiments in reviews or by discerning the context of user queries, allowing the system to provide more contextually relevant product suggestions and also how they feel about those products or services offered. AI-driven product recommendations allow businesses to harness the wealth of information embedded in textual data. By understanding the nuances of language, sentiments, and user intent, the integration of NLP enhances accuracy, personalization and recommendations, ultimately contributing to a more effective and satisfying shopping experience for users.
Risk management and ethical considerations
AI driven personalisation also brings attention to ethical considerations. The balance between personalisation and privacy plays a pivotal role. Businesses should implement transparent data practices, ensuring users have control over their data and AI algorithms adhere to ethical guidelines to avoid compromising customer privacy. Sales engineers should regularly audit the AI algorithms to mitigate any decision making risks made on partial data and should opt for diverse datasets.
Conclusion
In conclusion, AI’s role in data driven personalisation is transformative, reshaping how businesses connect with their audiences. Through data analytics, user behaviour analysis, machine learning algorithms, predictive analytics, real-time adaptation, segmentation, multi-channel personalisation, NLP, dynamic content delivery, and ethical considerations, AI empowers businesses to offer tailored experiences, driving engagement, fostering customer loyalty and providing better quality services. As the technology continues to evolve, the era of data driven personalisation is poised to advance, providing increasingly customer focused, consumer centric individualised interactions to customers. The role of AI in product recommendations for businesses is transformative and instrumental in shaping an efficient customer experience. The use of AI has empowered businesses to not only meet but exceed customer expectations. Ultimately, it’s been beneficial in contributing to sustained business growth in the dynamic world of ecommerce. As AI technology continues to grow for the better, the future holds more opportunities for businesses to refine and innovate their recommendation strategies, further enhancing the customer experience and their journey to build successful long term relationships of trustworthiness and provide high quality services.
References
- https://www.forbes.com/sites/forbescommunicationscouncil/2024/01/05/ai-and-personalization-in-marketing/?sh=15cf458b6856
- https://hyscaler.com/insights/ai-advertising-potential-2024/
- https://readitquik.com/articles/ai/elevating-customer-engagement-the-impact-of-ai-and-machine-learning-in-marketing/
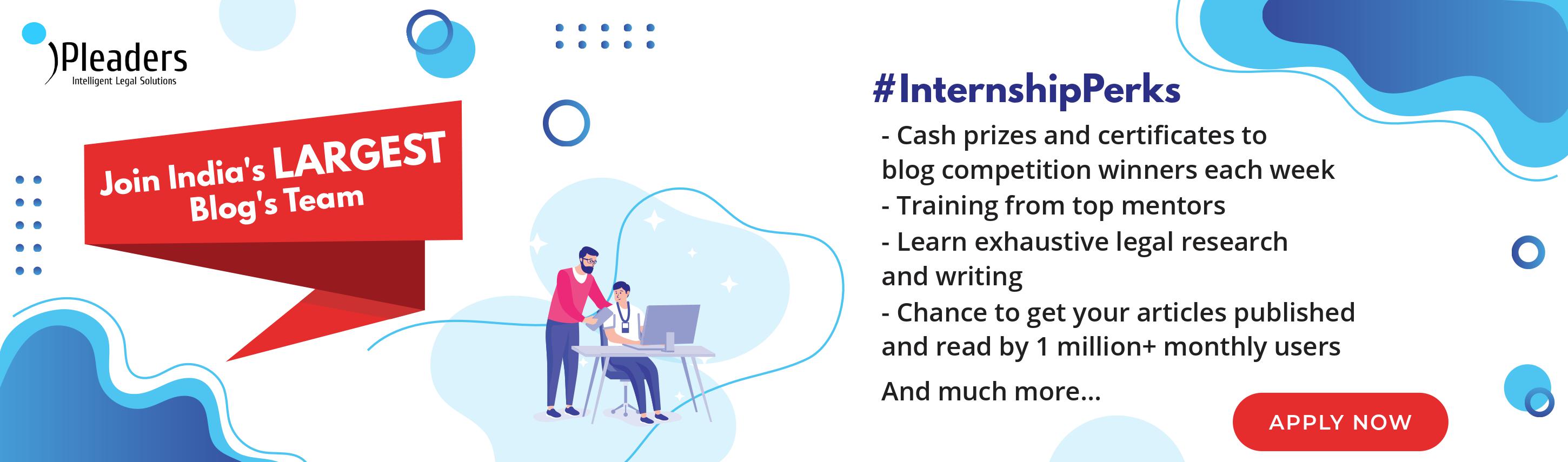