This article has been written by Yash Vardhan Singh pursuing a Diploma in Technology Law, Fintech Regulations and Technology Contractscourse from LawSikho.
This article has been edited and published by Shashwat Kaushik.
Table of Contents
Introduction
We live in an era dominated by rapid technological advancements. Artificial Intelligence (AI) stands at the forefront of innovation, promising unprecedented capabilities and efficiencies. As AI systems become increasingly integrated into various aspects of our lives, the ethical implications surrounding their development and deployment become more pressing. This article delves into the intricate relationship between AI and ethics, exploring the challenges, concerns, and imperative considerations that arise as we navigate the uncharted territory of intelligent machines shaping our future.
The first thing that should keep you up at night is that people may have no idea that artificial intelligence is making a decision that directly impacts their lives, like what percentage interest rate you get on your loan, whether you get that job that you applied for, whether you get into that college that they really want to go to, and so on and so forth. Today, AI is making most of these decisions, decisions that directly impact you in a big way.
The second thing that should keep you up at night is that, even when people know that an AI is making a decision about them, they may assume that, because it’s not a fallible human with bias, somehow the AI is going to make a decision that’s morally or ethically squeaky clean, and that could not be farther from the truth.
So, if you think about organisations dealing with AI, what happens over 80% of the time is that the ‘proof of concepts’ associated with artificial intelligence actually gets stalled in testing and more often than not, it is because people do not trust the results of that AI model.
Pillars of trust and principle of AI
So, we’re going to talk a lot about trust and when thinking about trust there’s actually five pillars. When you’re thinking about what it takes to earn trust in an artificial intelligence that’s being made by your organisation or procured by your organisation,. There are five pillars of trust in this realm.
The first thing to be thinking about is ‘fairness’. How can you ensure that the AI model is fair to everybody, in particular historically underrepresented groups?
The second is ‘explainable’. Is your AI model explainable in such a way that you’d be able to tell somebody, an end user, what data sets were used in order to curate that model, what methods were used, what expertise the data lineage in provenance was associated with, and how that model was trained.
The third is ‘robustness’. Can you assure end users that nobody can hack such an AI model that a person could disadvantage other people and/or make the results of that model benefit one particular person over another?
The fourth is ‘Transparency’. Are you telling people, right off the bat, that the AI model is indeed being used to make that decision and are you giving people access to a fact sheet or metadata so that they can learn more about that model?
And the fifth one and maybe the biggest pillar, is ‘Data Privacy’. Are you ensuring people’s data privacy? So, those are the five pillars.
Apart from these 5 pillars, there are three principles when thinking about AI in an organisation.
- The first is that the purpose of artificial intelligence is really meant to augment human intelligence, not replace it.
- The second is that data and the insights from those data belong to the creator alone.
- The third is that AI systems and the entire AI life cycle really should be transparent and explainable.
Now, the next thing we should remember in this space of earning trust and using artificial intelligence is that this is not a technological challenge but more of a question of willingness or unwillingness. It can’t be solved with just throwing tools and tech. This is a Socio-Technological challenge, and it must be addressed holistically.
Holistically, there are three major things that you should think about. First are people; another is the culture of the organisation; and third is the diversity of the entire AI ecosystem team.
Let’s say your data science team is curating that data to train that model. How many women are on that team? How many minorities are on that team? Think about diversity. One must have heard of the phrase “wisdom of crowds.” That’s actually a proven mathematical theory, the more diverse your group of people, the less chance for error, and that is absolutely true in the realm of artificial intelligence.
The second thing is process or governance, what/who is it that uses your organisation, What are you going to promise to both your employees and the market with respect to what standards you’re going to stand by for your AI model in terms of things like fairness, explainability, accountability, etc.
Risk of LLM (large language models)
With all the excitement around ChatGPT, it’s easy to lose sight of the unique risks of generative AI. Large language models (LLM), a form of generative AI, are really good at helping people who struggle with writing English prose. It can help them unlock the written word at a low cost and sound like a native speaker.
Since they’re so good at generating the next syntactically correct word, large language models may give a false impression that they possess an actual understanding or meaning of the language. The results can include a flagrantly false narrative directly as a result of its calculated predictions versus a true understanding. Let us see an example of the same. When we translate from one language to another, we need to understand the context of that sentence. It’s not just a case of taking each individual word from, say, English and then translating it into another language. We need to understand the overall structure and context of what’s being said. Assume if you take the phrase “spirit is willing, but the flesh is weak” and translate that from English to Russian and then you translate that Russian translation back into English, you’re going to go from “spirit is willing, but the flesh is weak” to something a bit more like “vodka is good, but the meat is rotten,” which is really not the intended context of that sentence whatsoever. It translated spirit into vodka and flesh into meat while changing the whole context.
So ask yourself: What is the cost of using an AI that could spread misinformation? What is the cost to your brand, your business, individuals or society? Could your large language model be hijacked by a bad actor?
Let us see how risk can be reduced.
It falls into four areas: hallucinations, biases, consent, and security.
AI hallucinations
Let’s start with the falsehoods, often referred to as “AI hallucinations.” However, I really don’t like the word “hallucinations” because I fear it anthropomorphizes AI. Let’s understand it a bit. You’ve probably heard the news reports of large language models claiming they’re human, claiming they have emotions, or just stating things that are factually wrong.
What’s actually going on here? Well, large language models predict the next best syntactically correct word and usually not accurate answers based on understanding of what the human is actually asking for, which means it’s going to sound great, but might be 100% wrong in its answer.
LLMs are trained on a plethora of texts that may contain noise, errors, biases or inconsistencies. For example, some LLMs were trained by scraping all of Wikipedia and all of Reddit. Is everything on Reddit 100% accurate?
So LLMs may generalise from data without being able to verify its accuracy or relevance. And sometimes it just gets it wrong
This wrong answer is a statistical error. Let’s take a simple example. Who authored the poems A, B, and C? Let’s say they were all authored by the poet X, but there’s one source claiming it was the author Z. And since we know that the source of all this data is the internet and all other data we feed the LLMs, we can have conflicting sources in the training data, like you would have seen conflicting answers to something you would have googled recently.
Which one actually wins the argument? Even worse, there may not be a disagreement at all, but again, a statistical error. The response could very well be incorrect because, again, the large language models do not understand, meaning these inaccuracies can be exceptionally dangerous. It’s even more dangerous when you have large language models annotating their sources for totally bogus answers.
Why? Because it gives the perception that it has proof when it just doesn’t have any. Imagine a call centre that has replaced its personnel with a large language model, and it offers a factually wrong answer to a customer.
This brings us to our first mitigation strategy: Explainability
Now, you could offer inline explainability and pair a large language model with the system that offered real data and data lineage and provenance via a knowledge graph.
Why did the model say what it just said? Where did it pull its data from? Which sources? The large language model could provide variations on the answer and explain different choices it made in its answer via a knowledge graph.
Bias
Imagine you asked your LLM for a list of the best poets in modern history. Do not be surprised if the output for your original query only lists white male Western European poets. Want a more representative answer? Your prompt would have to say something like, “Can you please give me a list of poets that include women and non-Western Europeans?”
Don’t expect the large language model to learn from your prompt. This brings us to the second mitigation strategy: Culture and Audits
It starts with approaching this entire subject with humility, as there is so much that has to be learned and, in some cases, unlearned. You need teams that are truly diverse and multidisciplinary in nature working on AI because AI is a great mirror into our own biases. Let’s take the results of our audits of AI models and make corrections to our own organisational culture when there are disparate outcomes. Audit pre-model deployment as well as post-model deployment.
Consent
Is the data that you are curating representative? Was it gathered with consent? Are there any copyright issues? These are things we can and should ask for.
This should be included in an easy to find, understandable fact sheet. Often, we subjects have no idea where the training data came from for these large language models, where it was gathered from. Did the developers hoover the dark recesses of the Internet to get some of that data?
To mitigate consent-related risk, we need the combined efforts of auditing and accountability. Accountability includes establishing AI governance processes, making sure you are compliant to existing laws and regulations and offering ways for people to have their feedback incorporated.
Security
Large language models could be used for all sorts of malicious tasks, including leaking people’s private information, helping criminals phish, spam, and scam. Hackers have gotten AI models to change their original programming, endorsing things like racism, suggesting people do illegal things. It’s called jailbreaking.
Another attack is an indirect, prompt injection. That’s when a third party alters a website, adding hidden data to change the AI’s behaviour, since we know AI devours the internet data to train and hence can end up suggesting all sorts of wrong information that the malicious actor intended. The result? Automation relying on AI could potentially send out malicious instructions without you even being aware.
This brings us to our final mitigation strategy, and the one that actually pulls all of this together is education. Let’s see an example.
Training a brand-new large language model produces as much carbon as over 100 roundtrip flights between New York and Beijing. This means it’s important that we know the strengths and weaknesses of this technology.
It means educating our own people on the principles for the responsible curation of AI, the risks, the environmental cost, the safe guardrails, and what the opportunities are.
Today, some tech companies are just trusting that large language model training data has not been maliciously tampered with. However, one can buy a domain and fill it with bogus data. By poisoning the dataset with enough examples, you could influence a large language model’s behaviour and outputs forever.
Conclusion
This tech isn’t going anywhere. We need to think about the relationship that we ultimately want to have with AI. If we’re going to use it to augment human intelligence, we have to ask ourselves the question:
What is the experience like for a person who has been augmented? Are they indeed empowered? We need to make education about the subject of data and AI far more accessible and inclusive than it is today. We need more seats at the table for different kinds of people with varying skill sets to work on this extremely important topic.
References
- https://www.techtarget.com/searchenterpriseai/tip/Generative-AI-ethics-8-biggest-concerns
- https://deepmind.google/discover/blog/evaluating-social-and-ethical-risks-from-generative-ai/
- https://hbr.org/2023/05/how-to-avoid-the-ethical-nightmares-of-emerging-technology
- https://www.coe.int/en/web/bioethics/common-ethical-challenges-in-ai
- https://arxiv.org/abs/2311.17228#:~:text=The%20ethical%20concerns%20associated%20with,and%20explainability%2C%20and%20environmental%20impact.
- https://www.dqindia.com/earning-trust-in-ai-is-it-a-technological-challenge-or-socio-technological-challenge/
- https://techinsiderbuzz.com/blog/ai-ethics-societal-issue/
- https://www.ibm.com/topics/ai-ethics#:~:text=Examples%20of%20AI%20ethics%20issues,%2C%20trust%2C%20and%20technology%20misuse
- https://penntoday.upenn.edu/news/social-scientists-must-address-chatgpts-ethical-challenges-using-it-research
- https://news.mit.edu/2023/bringing-social-ethical-responsibilities-computing-forefront-0608
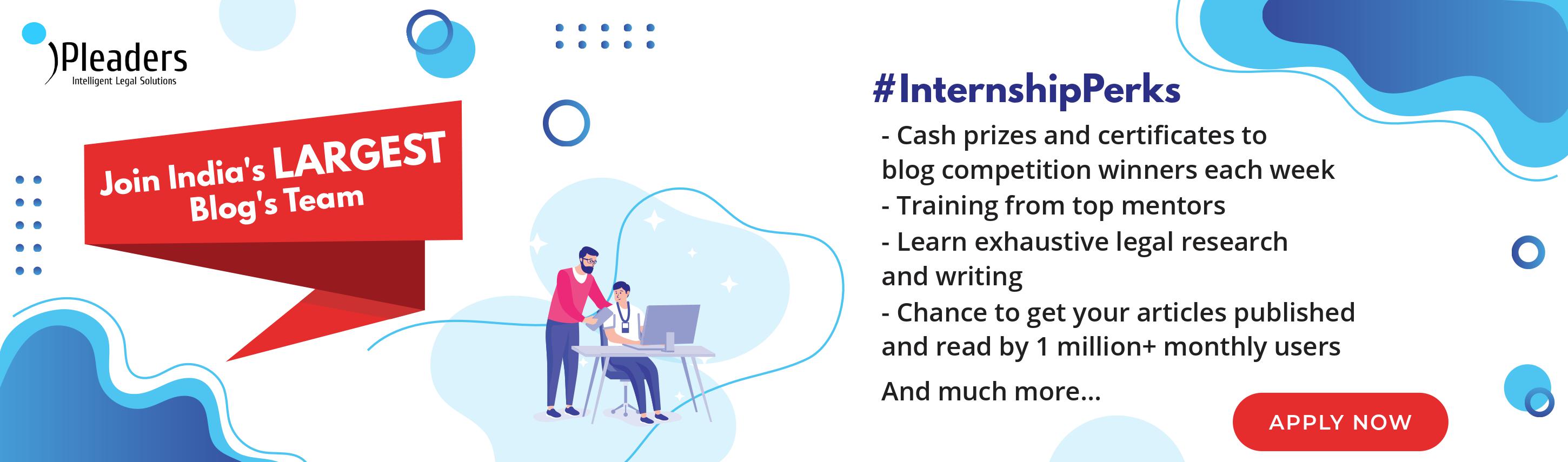