This article has been written by Yash Vardhan Singh pursuing a Diploma in Technology Law, Fintech Regulations and Technology Contracts course from LawSikho.
This article has been edited and published by Shashwat Kaushik.
Table of Contents
Introduction
With all the constant and ever-increasing buzz around AI, it’s natural to develop a huge interest in AI and its interaction with the legal system. So, one might start reading the EU AI Act, the everyday news about AI, various podcasts on AI and every relevant material out there regarding AI and where it intersects with the law. However, it’s also equally natural to face the problem that any lawyer with a non-science/tech background would face, of not understanding all the critical technical terms and keywords that are necessary to understand AI substantially. One cannot really understand the AI laws and the need for their regulation unless one understands AI itself. So, let’s acquire a basic, fundamental understanding of AI in simple language with examples for anybody who wants to understand it and bridge that knowledge gap.
What does it even mean to be artificially intelligent
AI, in simple words, is basically the simulation of intelligent behaviour in computers for performing human-like tasks.
Now, what do we mean by something being intelligent? And more importantly, are the so-called “intelligent” machines there yet? Are they really intelligent in a real sense? Let’s figure it out. Let’s see, if one put up a number like 3467 and multiplied it by say 234 and if he/she could tell you after a calculation in head, just like that, the answer to that multiplication is xyz, is that intelligence? It is indeed really impressive. But, you know, you’ve got a calculator. It can do the same thing. And you can really not declare that your calculator is an intelligent sentient. So, it’s not artificially intelligent.
Well, how about our school days? Our science teacher considered intelligence to be if we knew all the elements in the periodic table, where they went, what their atomic numbers were, how to spell them and their abbreviations, and if we could place all of them where they belonged. And that would have been considered intelligent.
Was she right?
With the greatest respect to all science teachers, that’s not intelligence either. What they just described as intelligent is a database lookup.
How about, then, something a bit more advanced than that? Something that takes years and years to get good at, like a chess grandmaster. Now, think of all of the time that you have to invest to learn all of the patterns, all of the moves, and all of the strategies. Is a chess grandmaster a real example of intelligence? Yeah, that’s what most people would say, somebody who is the best in the world at chess is a genius. So, you would think, if you’re a genius, you’re intelligent.
But guess what? IBM did this trick in 1997 when they created a computer called “Deep Blue.” And Deep Blue was able to beat the best chess player in the world, Gary Kasparov, handily. So, already, if that’s your bar, we’ve passed it. Yet again, we kind of feel like we’re left empty. We’re still not feeling there yet.
So, what is the classic question—the classic problem to solve? The answer to this could be the Turing test.
For those who don’t know, with the Turing Test, you basically have a user on one side of a wall and they are typing to another person behind a wall and they cannot see who it is that they are typing to.
So, they’re typing messages back and forth, and they may be talking to another person, or they may be talking to a computer. And if it’s indistinguishable when they have their communications and they can’t tell if they’re talking to another person or talking to a computer, then we would declare “game over.” The computer has achieved artificial intelligence. So, if we could ever do that, then we’d be there, right?
Well, this one is a little bit disputed as to whether we passed the Turing test or not. But in 2014, technically, some people say that we did it with a chatbot that simulated a 13-year-old boy. Well, so that’s setting the bar kind of low, 13-year-old boy, we need to set the bar higher.
But would we consider other technologies that have raised the bar further towards the Turing Test? One might talk to Chat GPT daily, and sometimes one could be convinced that this is a real person and there have been a lot of advances in that kind of area. Clearly, the bar has been raised.
If we ask the question, “Are the machines there yet or not?” it appears like we move the finish line every time we get close to it. As soon as we cross it, then we say, “Yeah, but that’s not quite there yet.”
So, we kind of had this sense that, if we look at all of these things together, we have these sorts of compartmental intelligence. We have something that’s really great at arithmetic, we have something that’s really great at memorization and recall, something that’s great at a narrowly bounded game, that is quite complex, but still has very specific rules that can be mathematically described.
So, we continue to have these developments in individual areas, but putting them all together so that one machine can do it all—that’s your AGI or artificial general intelligence—is certainly not there yet.
But it’s also worth pointing out that the development curve for AI just seems to have changed, even from last year; it’s just going faster and faster than ever before. And we are getting closer and closer to this idea of general intelligence, moving away from where we have everything siloed, like Deep Blue, which is deeply siloed to just chess, to now these AI systems that are really getting closer and closer to being able to accomplish much more of what we do as humans.
When most of us were in school, we talked about artificial intelligence, and it was always about something that was about five or ten years away. And then, ten years later, it was another five or ten years away. But now it really feels like we’re actually narrowing the gap. In the last few years, it’s been this steep curve towards what we would consider general artificial intelligence.
So, are we there yet? No, but we’ve never been closer.
Types of AI
All of artificial intelligence or AI, is classified into seven types. And that’s a tall order. But these seven types of AI can largely be understood by examining two encompassing categories. The two categories are “AI Capabilities,” and then there’s “AI Functionalities.” So, let’s start with AI capabilities, and there are three subcategories.
The first of which is known as artificial Narrow AI, which also goes by the rather unflattering name “Weak AI.” Now, on its face, that doesn’t sound like Narrow AI is a very interesting capability to start us off. But actually, narrow AI is the only type of AI that exists today and it’s all we currently have. Any other form of AI is theoretical. So, we can think of Narrow AI as ‘Realised AI’ as that’s the artificial intelligence we have today and theoretical AI, which is the artificial intelligence we may have in the future.
Now narrow AI can be trained to perform a narrow task, which, to be fair to narrow AI, might be something that a human could not do as well as the AI can but it cannot perform outside of its defined task. It still needs us humans to train it. So, if narrow AI represents all the AI capabilities we have today, well, what else is there?
Well, a favourite of memes, science fiction movies and books is Artificial General Intelligence, also known as AGI. And also known as “Strong AI.” To be clear, general AI or AGI, is currently nothing more than a theoretical concept. But here’s the idea: AGI can use previous learnings and skills to accomplish new tasks in a different context, without the need for us human beings to train the underlying models. If AGI wants to learn how to perform a new task, it will figure it out by itself. This sounds disconcerting but we haven’t even talked about the third type of AI capability yet and that’s artificial “super AI.”
If super AI is ever realised, it would think, reason, learn, make judgments and possess cognitive abilities that surpass those of human beings. The application’s possessing super AI capabilities would have evolved beyond the point of catering to human sentiments and experiences, and would be able to feel emotions, have needs and possess beliefs and desires of their own. Yes, you guessed it right, it can be like Vision or maybe even Ultron (the hero or villain that the AI will itself decide as it has its own cognitive abilities) from your Marvel comics.
Yeah. So, let’s park that scary (or exciting) thought for now and consider the four types of AI based on functionalities. And we’re back in the real world of realised AI here, at least initially. So, we can think of narrow AI as having two fundamental functions.
Reactive AI
These machine systems are designed to perform a very specific, specialised task. Reactive AI stems from statistical maths, and it can analyse vast amounts of data to produce a seemingly intelligent output. We’ve had reactive AI for quite a while. Back in the late 1990s, IBM’s chess playing supercomputer Deep Blue beat chess grandmaster Garry Kasparov by analysing the pieces on the board and predicting the probable outcomes of each move. That’s a specialised task with a lot of available data to create insights, the hallmark of reactive AI. The self-driving car is also an example of reactive AI.
Reactive AI is widely utilised in numerous applications, including:
- Natural Language Processing (NLP): Reactive AI powered chatbots, virtual assistants, and language translation tools, allowing them to comprehend and respond to human language.
- Recommendation systems: Reactive AI analyses user preferences and behaviours to provide personalised recommendations for products, movies, or music.
- Image and speech recognition: Reactive AI enables devices to identify and interpret visual and auditory information, enhancing features such as facial recognition and voice commands.
- Predictive analytics: Reactive AI can analyse historical data to make predictions about future outcomes, aiding in decision-making processes.
While reactive AI exhibits impressive capabilities, it has certain limitations. It lacks the ability to learn and adapt over time, making it suitable for tasks that require consistent and predictable responses. Additionally, reactive AI systems are often trained on specific datasets and may not perform optimally when presented with unfamiliar or out-of-scope data.
As technology advances, reactive AI continues to evolve, becoming even more adept at handling complex tasks and interacting with humans in a more natural way. It plays a vital role in automating routine processes, enhancing user experiences, and driving innovation across various sectors.
Limited memory AI
We can really think of other narrow AI functionalities as being classified as “limited memory AI.” This form of AI can now recall past events and outcomes and monitor specific objects or situations over time. It can use past and present moment data to decide on a course of action most likely to help achieve a desired outcome. And as it’s trained on more data over time, limited memory AI can improve in performance. Think of your favourite generative AI chatbot, which relies on limited memory AI capabilities to predict the next word, the next phrase or the next visual element within the context it’s generating. Hence, in a way, our autocorrect, sentence-completing suggestions in email, and Youtube’s copyright detection algorithm are examples of it.
Now, what about our two theoretical AI capabilities? Well, if we look at AGI, we have to think about “theory of mind AI.” Now, this would understand the thoughts and emotions of other entities so it could infer human motives and reasoning and personalise its interactions with individuals based on their unique emotional needs and intentions. And actually, emotion AI is a theory of mind AI currently in development. AI researchers hope it will have the ability to analyse voices, images and other kinds of data to understand and respond to human feelings. Finally! Somebody (even though artificial) really understands you.
And then, under super AI, we have “Self-Aware AI,” the scariest AI of all, if you would like to call it that. It would have the ability to understand its own internal conditions and traits, leading to its own set of emotions, needs and beliefs. Vision and Ultron fans, assemble.
We’ve covered seven types of AI, and only three of them actually exist today! There is still so much to be learned and discovered. But as those advancements happen, at least here we have a taxonomy of AI types that will tell us how far along we are on our AI journey.
Machine learning
There’s no doubt that this is an incredibly hot topic with significant interest from both business professionals and technologists. So, let’s talk about what machine learning or ML is.
Differences between machine learning, AI, and deep learning
So, before we get too far into the details, let’s talk about some terms that are often used interchangeably but have certain differences. Terms like “artificial intelligence,” “machine learning,” and even “deep learning”.
So, at the highest level, AI is defined as leveraging computers or machines to mimic the problem-solving and decision-making capabilities of the human mind. Machine learning is a subset within AI that’s more focused on the use of various self-learning algorithms that derive knowledge from data in order to predict outcomes. And then, finally, deep learning is a further subset within even machine learning, and deep learning is often thought of as scalable machine learning because it automates a lot of the feature extraction process and eliminates some of the human intervention involved to enable the use of some really, really big data sets.
But let’s just focus on machine learning, so we’ll get rid of the other two and dive one level deeper and talk about the different types of machine learning.
Supervised and unsupervised learning
This is when we use labelled data sets to train algorithms to classify data or predict outcomes. And when we say labelled, we mean that the rows in the data set are labelled, tagged, or classified in some interesting way that tells us something about that data. So, it could be a yes or a no, or it could be a particular category of some different attribute.
So how do we apply supervised machine learning techniques?
Well, this really depends on your particular use-case. We could be using a classification model that recognises and groups ideas or objects into predefined categories. An example of this in the real world is customer retention.
So, if you’re in the business of managing customers, one of your goals is typically minimising and identifying customer churn, which are customers that no longer buy a particular product or service, and we want to avoid churn because it’s almost always more costly to acquire a new customer than it is to retain an existing one, right?
So, if we have historical data for the customer, like their activity – whether they churned or not—we can build a classification model using supervised machine learning and our labelled dataset that will help us identify customers that are about to churn and then allow us to take action to retain them.
Ok, so the other type of supervised learning is “regression.” Now, this is when we build an equation using various input values with their specific weights determined by the overall value of their impact on the outcome and we use these to generate an estimate for an output value.
So, let’s see another example here. So, airlines rely heavily on machine learning, and they use regression techniques to accurately predict how much they should be charging for a particular flight, right? So, they use various input factors, like the days before departure, the day of the week, the departure, and the destination, to predict an accurate dollar value for how much they should be charging for a specific flight that will maximise their revenue.
Now let’s move on to the second type of machine learning, which is “unsupervised learning.”. This is when we use machine learning algorithms to analyse and cluster unlabeled data sets, and this method helps us discover hidden patterns or groupings without the need for human intervention.
So, we’re using unlabeled data here. So, again, let’s talk about the different techniques for unsupervised learning. One method is “clustering.” And a real-world example of this is when organisations try to do customer segmentation.
So, when businesses try to do effective marketing, it’s really critical that they really understand who their customers are, so that they can connect with them in the most relevant way. And, oftentimes, it’s not obvious or clear how certain customers are similar to or different from one another. Right, clustering algorithms can help take into account a variety of information on the customer, like their purchase history, their social media activity, or their website activity, which could be their geography, and much more, to group similar customers into buckets so that we can send them more relevant offers, provide them better customer service, and be more targeted with our marketing efforts.
Reinforcement learning
The last type of machine learning we need to talk about is called “reinforcement learning.”. This is a form of semi-supervised learning where we typically have an agent or system take actions in an environment. Now the environment will either reward the agent for correct moves, or punish it for incorrect moves. And, through many iterations of this, we can teach a system a particular task.
A great example of this method in the real world is with self-driving cars. So, autonomous driving has several factors, right? There’s the speed limit, there are drivable zones, there are collisions, and so on. So, we can use forms of reinforcement learning to teach a system how to drive by avoiding collisions, following the speed limit, and so on.
Foundation models and generative AI
In the past couple of months, large language models, or LLMs, such as ChatGPT, have taken the world by storm. Whether it’s writing poetry or helping plan your upcoming vacation, we are seeing a step change in the performance of AI and its potential to drive enterprise value.
Now, large language models or LLMs, are actually part of a different class of models called foundation models. Now, the term “foundation models” was actually first coined by a team from Stanford when they saw that the field of AI was converging to a new paradigm. Whereas before AI applications were being built by training, maybe a library of different AI models were trained on very task-specific data to perform very specific tasks.
They predicted that we were going to start moving to a new paradigm, where we would have a foundational capability, or a foundation model, that would drive all of these same use cases and applications.
So, the same exact applications that we were envisioning before with conventional AI, and the same model could drive any number of additional applications. The point is that this model could be transferred to any number of tasks and this gives this model the superpower to be able to transfer to multiple different tasks and perform multiple different functions.
It’s been trained on a huge amount, in an unsupervised manner, on unstructured data and what that means, in the language domain is basically that you’ll feed a bunch of sentences and we are talking terabytes of data here to train this model. For example: the start of my sentence might be “no use crying over spilled,” and the end of my sentence might be “milk.” And I’m trying to get my model to predict the last word of the sentence based on the words that it saw before. This prediction comes from feeding an enormous amount of data where the last word would be “milk.”
Now, talking about generative AI, it’s this generative capability of the model predicting and generating the next word based off of previous words that it’s seen beforehand. That is why foundation models are actually a part of the field of AI called generative AI, because we’re generating something new in this case (as something new is generated), the next word in a sentence.
All of these examples that we’ve talked through so far have just been on the language side. But the reality is, there are a lot of other domains that foundation models can be applied to. Famously, we’ve seen foundation models for vision looking at models such as DALL-E 2, which takes text data, and that’s then used to generate a custom image.
We’ve seen models for code with products like Copilot that can help complete code as it’s being authored. There are also models for climate change, including Earth Science Foundation models using geospatial data to improve climate research. So, basically, generative AI is everywhere now.
Conclusion
With this description, it is hoped a non tech savvy lawyer will be able to at least grasp the understanding of this ever-changing enigma called AI. It’s very important to understand its fundamentals to be able to advise on legislation and regulation around it, as with its bloom comes the responsibility to put a lid on the devil’s casket. The excessive use of the word “data” in the entire article is enough to point out that data is the bedrock of AI and with lots of data it is meant as data with a capital D as it consumes terabytes of data and most of the time we don’t know where that data came from; it could be your personal data or mine and we would never know. Hence, to have an AI law, we need to have a very strong data privacy law first.
References
- https://research.ibm.com/blog
- https://www.techtarget.com/searchenterpriseai/definition/AI-Artificial-Intelligence
- https://cloud.google.com/learn/what-is-artificial-intelligence
- https://www.ibm.com/topics/artificial-intelligence
- https://www.britannica.com/technology/artificial-intelligence
- https://techcrunch.com/2024/1/4/chatgpt-everything-to-know-about-the-ai-chatbot/
- https://www.forbes.com/advisor/in/business/ai-statistics/
- https://www.fiercebiotech.com/biotech/ai-not-panacea-all-drug-discovery-challenges-partnerships-can-be-generates
- https://acuraip.com/the-intersection-of-artificial-intelligence-and-intellectual-property-law/
- https://www.researchgate.net/publication/320057692_Intersections_between_Law_and_Artificial_Intelligence
- https://tclf.in/2020/07/13/intersection-of-artificial-intelligence-and-copyright-law/
- https://hackernoon.com/embracing-the-human-touch-the-intersection-of-ai-ml-and-legal-compassion
- https://hackernoon.com/embracing-the-human-touch-the-intersection-of-ai-ml-and-legal-compassion
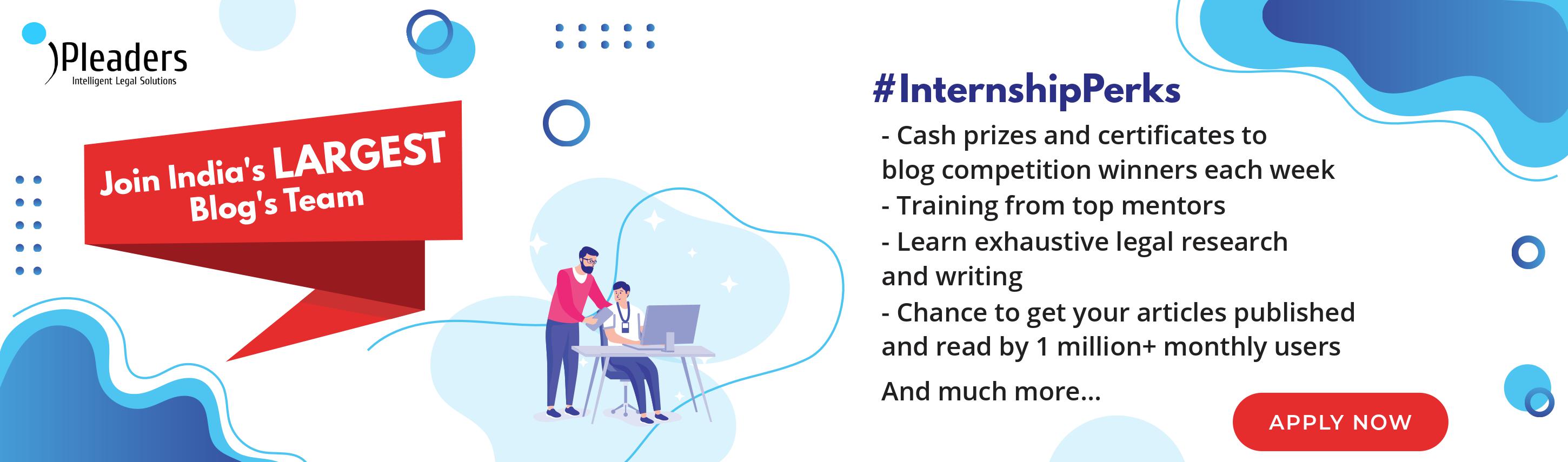