This article has been written by Nagesh Karale pursuing a Diploma in US Intellectual Property Law and Paralegal Studies course from LawSikho.
This article has been edited and published by Shashwat Kaushik.
Table of Contents
Introduction
Artificial intelligence (AI) is a computer science field that studies and develops intelligent machines. These machines are able to think, learn, and solve problems similarly to humans. AI’s goals include computer-enhanced learning, reasoning, and perception. AI systems can process information, identify patterns, and make decisions autonomously. The progression of data science and computing has simplified the decision-making process. It is used across different industries, from finance to healthcare. Deep learning is a method in AI that teaches computers to process data in a way that is inspired by the human brain.
AI technology has widespread applications across various fields, including speech recognition, surgical robots, image analysis, autonomous vehicles, and natural language processing. It is reshaping industries such as business, finance, chat bots, robotics, cyber security, manufacturing, health, education, transport, farming, and public administration.
AI generated inventions and patentability
The patentability of AI-generated inventions raises complex questions in law and policy. AI- created inventions, such as applications or devices created by smart computers, can perform difficult tasks autonomously. They keep learning and improving themselves over time. Courts and patent offices have rejected applications for AI-generated inventions, with a few exceptions. This is because the patent law is based on the assumption that inventors are humans. Some government bodies and courts have also stated that inventions made with the help of AI cannot be patented.
An American scientist and inventor, Dr. Stephen Thaler, created an AI system called DABUS (Device for the Autonomous Bootstrapping of Unified Sentience). DABUS is a type of ‘connectionist AI’. It uses multiple neural networks to generate new ideas, the novelty of which is then assessed by a second system of neural networks. Through this process, DABUS has autonomously generated two “inventions.” The first was a fractal container (a food container) and the second was a neural flame (a search and rescue beacon). Dr. Thaler’s patent applications have been unsuccessful in New Zealand, Taiwan, Israel, the Republic of Korea, Canada, Brazil, and India. To date, South Africa and Saudi Arabia are the only exceptions, although in both of those jurisdictions, the patents have not yet undergone substantive examination.
The inventorship issue of AI generated inventions has created problems for patentability. There is no clear cut criteria for deciding “autonomously generated” inventions by artificial intelligence (AI). AI systems are playing an increasingly important role in innovation. The question has been asked about how the patent system will protect AI-generated inventions. The traditional patent laws have failed to distinguish between AI as a tool and AI as the primary developer of inventions. The big companies are ready for investment in AI development. But the uncertainty about patenting AI inventions could affect innovation and economic growth.
If AI inventions can’t be patented, there might be less investment in AI technology. Some propose placing AI-generated creations in the public domain for open access and shared benefits. On the other hand, there are arguments in favour of protecting AI work through patents, which incentivise investment and innovation. Some have raised concern that an excessive number of patents for AI inventions could potentially badly affect research and development. The use of AI in innovation introduces risks to trade secrets.
However, the Full Court of the Australian Federal Court suggested a number of options regarding the inventorship of the relevant patents, namely:
- the owner of the machine upon which the AI software runs;
- the AI software developer;
- the owner of the copyright in its source code; and
- the person who inputs the data used by the AI to develop its output.
The UK Intellectual Property Office consultation report, Year 2022, identified several options for patent law reform, including:
- expanding the definition of ‘inventor’ to include the humans responsible for an AI system that generates inventions;
- allowing AI to be identified as the inventor; or
- protecting AI-devised inventions by means other than the patent system.
Copyright protection for AI created works
There is an emergence of generative AI systems like ChatGPT, Bard, DALL-E, Midjourney, and Stable Diffusion, which allow people to prompt AI to create literary, dramatic, and artistic works. AI, like humans, can now create art and music by learning from data and patterns. As per existing copyright laws, only humans are treated as creators. In one famous case (Naruto vs. Slater), where a monkey took selfies and PETA claimed copyrights to them, the US Ninth Circuit Court of Appeals rejected the claim of the monkey as creator. So animals cannot own copyright. The authorship of a work is associated with the examination of originality and creative input. The same is the hurdle to getting copyright for AI-created work.
As far as EU copyright is concerned, the work must be original. It must show the author’s intellectual creation and personality. The law is silent about the threshold of originality but emphasises the author’s freedom and creative choices. It must have a personal touch. In most European countries, the work that is human created gets copyright. Only the AI generated work that has a human author’s impact on the outcome is copyrighted. It may not be copyrighted if the AI-created work does not meet a sufficient threshold of originality.
If AI is used as a tool, then humans can retain creative freedom .So the AI-created work becomes copyrightable. If the created work is carried out independently by AI, then the AI generated work faces challenges for copyright. There must be clarity about the legal aspect of AI –generated work. In the U.S., copyright office guidance states that works containing AI-generated content require evidence of a creative contribution from a human author.
Midjourney, an AI tool, serves as an example of generative AI used for generating images from natural language descriptions. Midjourney raises new questions about copyright and fair use. Generative AI (GAI) mainly uses text-based artificial intelligence to create diverse content. GAI models use vast textual input data for learning. It analyses input data patterns to answer user queries. Unauthorised copying and use of data is treated as copyright infringement. GAI’s use of input works for training raises questions about whether it’s permitted or constitutes the preparation of a derivative of work.
Getty Images vs. Stability AI is a classic example of fair use defence. The legal framework for GAI-related IP issues is vague. It significantly impacts the growth of the generative AI industry and intellectual property law in this evolving landscape. This evolving landscape underscores the need for a comprehensive legal understanding of how GAI interacts with copyright law.
AI and trademark
AI algorithms serve the following primary purposes.
- AI in trademarks helps applicants register trademarks more easily by suggesting ways to improve applications.
- It identifies conflicts between new trademarks and existing rights to prevent potential legal issues.
- AI plays a crucial role in simplifying trademark processes and ensuring successful registrations.
- In trademark law enforcement, AI is instrumental in spotting unauthorised online use, including social media infringement.
So far, there is one famous case involving the intersection between AI and trademarks, known as Lush vs. Amazon. In this case, Amazon was found guilty of trademark infringement against Lush. Amazon had bid on the “Lush” keyword on Google. It caused people searching for “Lush” to be directed to Amazon. Amazon did not sell actual Lush products. Amazon’s AI system suggested similar products, which led to the court ruling that Amazon was responsible for infringement.
In e-commerce, the increasing use of AI raises concerns about brand manipulation. As AI takes on a more consumer-like role, legal battles may intensify. Courts might need to adapt traditional concepts such as the “average consumer” and “likelihood of confusion” for AI involvement. Due to the involvement of AI in online product ordering, the retail model is transitioning to predictive models. Still, there is a strong emotional connection between consumers and brands.
AI and trade secrets
Generative AI (GAI) is a powerful tool for data analysis and content creation. But it requires extensive input data for training and learning purposes. However, its capability to store vast amounts of information raises concerns about the potential exposure of private and sensitive data. Trade secrets that provide companies with a competitive edge require confidentiality. The US Defend Trade Secrets Act (DTSA) of 2016 mandates “reasonable measures” to keep such information secret. Due to many industries using GAI to create content autonomously, it increases the risk of a trade secret. The specific measures that are considered “reasonable” will vary depending on the circumstances of each case, but may include:
- Limiting access to trade secrets: Businesses should restrict access to trade secrets to employees and other individuals who need to know the information in order to perform their jobs. This may involve using access control systems, such as passwords or biometric identification, and requiring employees to sign non-disclosure agreements.
- Educating employees about trade secrets: Businesses should educate their employees about the importance of protecting trade secrets and the consequences of misappropriation. This education should include information about the legal definition of a trade secret, the company’s trade secret policies, and the potential penalties for misappropriation of trade secrets.
- Implementing physical security measures: Businesses should implement physical security measures to protect their trade secrets from unauthorised access. These measures may include installing security cameras, controlling access to buildings and offices, and using alarms and other security devices.
- Monitoring for misappropriation: Businesses should monitor for any signs of misappropriation of trade secrets. This may involve regularly reviewing employee emails and computer files, conducting background checks on employees and contractors, and investigating any suspicious activity.
Companies employing generative AI face challenges in safeguarding proprietary information and sensitive data. It may be vulnerable to leaks. In response, some businesses opt for bans or restrictions on the use of AI to minimise risks. However, the total ban on the use of GAI may have significant costs and potential competitive disadvantages. So the safe use of generative AI requires the implementation of robust security measures and clear policies. To minimise the risks associated with generative AI, it requires creating a culture of awareness within the organisation.
Following are the best practices for secure implementation of generative AI in business
- It is essential to implement limitations on access and carefully control the types of data inputs to safeguard proprietary information and sensitive data.
- Enterprise versions of generative AI applications, coupled with well-crafted End-User Licence Agreements (EULAs), can offer additional security measures. These agreements should mention the protection or deletion of any data collected by the AI.
- It is important to make sure that employees, contractors, and third parties follow the policies on generative AI. Employee education and awareness are essential for reducing risks related to generative AI.
- Taking proactive measures and regularly updating and reviewing security measures are essential steps to minimise the risk related to generative AI.
- For data protection, encryption and secure data transmission methods should be implemented. There should be a comprehensive incident response plan to address potential breaches promptly.
- The security policies should be formulated with the help of legal experts to ensure compliance with data protection laws and regulations. Generative AI applications should be regularly monitored and audited to detect any anomalies or unauthorised access.
- Culture of ethical AI use and responsible data handling should be encouraged within the organisation. The risks associated with generative AI use should be assessed regularly in the business context.
- To validate the security and compliance of generative AI systems, organisations should consider external certifications or audits. The organisation should engage in industry collaboration to share best practices and stay informed about emerging threats.
Data ownership and AI development
In the digital age, data and AI play central roles in various fields. Data is often compared to oil for its value in decision-making and innovation. AI needs extensive datasets to transform raw data into meaningful insights. The quality and accuracy of data are crucial for achieving business success. AI models heavily rely on the integrity of the input data to generate reliable output. Organised and searchable structured data becomes the foundation for the prediction of emerging trends. Unstructured data provides valuable insights that drive innovation and competitive advantage. Privacy concerns and ethical considerations are important issues in data usage in AI systems.
Adaption to evolving data patterns and maintaining relevance, continuous monitoring and updates of AI algorithms are necessary. Collective efforts by data sharing organisations can lead to industry-wide innovation. To minimise the risk of cyber threats, cyber security measures must be robust to safeguard sensitive data.
GAI models like GPT-4 pose challenges in terms of data ownership and privacy. Privacy related issues include data biases, mishandling risks, and potential identity theft. Privacy can be invaded by AI’s ability to generate realistic images and manipulate misleading material. Advanced AI voice technology increases the risk of impersonation and deception. Privacy rights may be compromised due to a lack of clear consent mechanisms and transparency. To safeguard privacy, there is a need for strong regulations and legal frameworks.
AI relies heavily on carefully selected data sets, especially in machine learning (ML), large language models (LLM), and deep learning (DL) for accuracy. Due to the analysis of vast amounts of input data, AI systems pose privacy and copyright risks.
The “black box” nature of AI makes it challenging to prove intellectual property infringement. Due to the significant data requirements of AI systems, it could potentially lead to legal issues and privacy concerns. Compliance with privacy laws is crucial for responsible AI use for content creation.
Ethical and legal considerations in AI and IPR
Ethical considerations in AI and IPR
Ethical considerations in AI and IPR are:
- Algorithmic bias and discrimination based on factors such as gender, race, etc. can occur when systematic errors in computer systems result in discriminatory outcomes, affecting individuals based on protected characteristics.
- The responsibility for AI infringement on IP rights for AI-generated content is not clearly fixed by the laws.
- To avoid ethical worries and prevent discrimination in AI work, human control is a must.
- AI systems should be adapted, considering technology gaps between countries, to ensure fairness, transparency, and accountability.
- Present AI systems are unable to understand and incorporate emotional depth and cultural context in generated works.
- There is a risk of power concentration in the hands of a few who control AI technology. It might raise ethical concerns about equity and access.
- There is debate over AI’s role in creativity, which raises questions about whether AI can fully replace human innovation and artistic expression. Some are afraid that the excess use of AI systems may demotivate humans to invent new things.
Legal considerations in AI and IPR
Legal considerations in AI and IPR are:
- Due to rapid evaluation of AI, traditional patent laws are facing challenges in determining inventorship, ownership, and law enforcement.
- There are different global views about the requirement of collaboration for organisations to establish legal frameworks and standards for AI technologies.
- Worldwide use of AI in Intellectual Property Offices (IPOs) is saving time for examiners, expediting processes, and enhancing trademark application procedures.
- For AI adoption globally, there is a requirement for a legal framework addressing technology gaps, energy consumption and job creation challenges. Intellectual property laws are required to accommodate the evolving nature of AI-generated content and inventions.
- AI systems require vast data sets, so legal frameworks are needed to regulate data sharing, responsible use and prevent misuse.
Future implications and policy recommendations
The changing landscape of AI and intellectual property calls for proactive policy measures to ensure fair, responsible, and beneficial outcomes for creators, users, and the public.
Ownership of AI-generated creations
There are debates revolving around whether AI developers or users should hold intellectual property rights for AI-generated content. Existing laws allow only work created by human skills for copyright. Patent law only allows humans to be inventors. The law is silent and not clear about AI assisted or AI created work.
Adaptation of IP laws for AI
Recognising AI’s potential as a creator may require adaptations to intellectual property laws. One of the proposals is to include broad user agreements allowing developers to define and negotiate ownership of AI creations. IP laws need updates to regulate works created solely by AI.
Liability challenges in AI evolution
AI systems are involved in diverse tasks. The liability of AI created work poses challenges. It has become difficult to fix responsibility for legal claims, such as copyright infringement or privacy breaches.
Human control and responsibility
There should be a clear cut law regarding the responsibility for harmful actions by AI, especially when operating autonomously. It highlights the need for clear legal status. Legislation should ensure humans can control AI decisions. So creators should be held responsible and pay penalties for AI mistakes.
Recognition and citizenship for AI
Instances like Sophia’s citizenship in Saudi Arabia raise questions about the recognition and legal status of AI. There should be a balance between commercialization and public benefit, considering IP laws and liability concerns.
Ethical considerations in AI development
Ethical issues related to AI-generated content, such as bias and fairness, must be addressed in policy frameworks. AI development practices should be focused on positive societal impacts.
International cooperation on AI policies
The formulation of policies and standards regarding AI creations needs collaboration among nations. There is a need to establish international standards for AI-generated content.
Conclusion
AI and Intellectual Property Rights (IPR) present challenges and opportunities in inventions, copyrights, trademarks, and trade secrets. It highlights key questions on patenting AI creations, copyright protection, and trademark impacts. Raising important issues such as data ownership, ethics, and policy frameworks should be properly resolved. There is a great need for clear regulations to recognise AI as a creator. There is a need for global collaboration for a balanced approach to innovation and intellectual property protection. In this evolving AI landscape, effective laws are essential to ensure human control over AI decisions.
References
- https://books.google.co.in/books?hl=en&lr=&id=R-AeEAAAQBAJ&oi=fnd&pg=PP1&dq=Overview+of+AI+advancements+and+their+impact+on+intellectual+property+rights&ots=yQATGHWLwc&sig=_dlxM3mJ6aljKy7WxKeYHIxJTJw#v=onepage&q=Overview%20of%20AI%20advancements%20and%20their%20impact%20on%20intellectual%20property%20rights&f=true
- https://www.legalserviceindia.com/legal/article-12485-impact-of-artificial-intelligence-on-intellectual-property.html#:~:text=AI%20is%20both%20a%20tool,infringements%2C%20across%20various%20online%20platforms.
- https://academic.oup.com/grurint/article/69/5/443/5854752
- https://blogs.worldbank.org/opendata/inventors-and-innovations-era-ai
- https://cms.law/en/media/local/cms-cmno/files/publications/publications/ai-created-works-creative-original-or-just-sweat-off-the-brow
- https://www.herbertsmithfreehills.com/insights/2023-05/the-ip-in-ai-does-copyright-protect-ai-generated-works
- https://techpolicy.press/copyright-fair-use-regulatory-approaches-in-ai-content-generation/
- https://www.universalcreativesolutions.com/blogs/post/harnessing-the-power-of-data-and-ai-driving-business-success-in-the-digital-age
- https://thepaymentsassociation.org/article/data-ownership-and-privacy-in-the-age-of-generative-ai/
- https://www.evalueserve.com/blog/data-privacy-and-ai-securing-intellectual-property-in-the-modern-digital-landscape/#:~:text=AI%20also%20risks%20trade%20secrets,inadvertently%20reveal%20this%20protected%20information.
- https://www.tilleke.com/insights/challenges-future-intellectual-property-issues-artificial-intelligence/
- https://www.wipo.int/export/sites/www/about-ip/en/artificial_intelligence/call_for_comments/pdf/ind_revella.pdf
- https://www.jonesday.com/en/insights/2023/06/trade-secrets-and-generative-ai#:~:text=To%20avoid%20the%20consequences%20of,uses%20of%20generative%20AI%20applications.
- https://www.herbertsmithfreehills.com/insights/2023-09/the-ip-in-ai-can-patents-protect-ai-generated-inventions
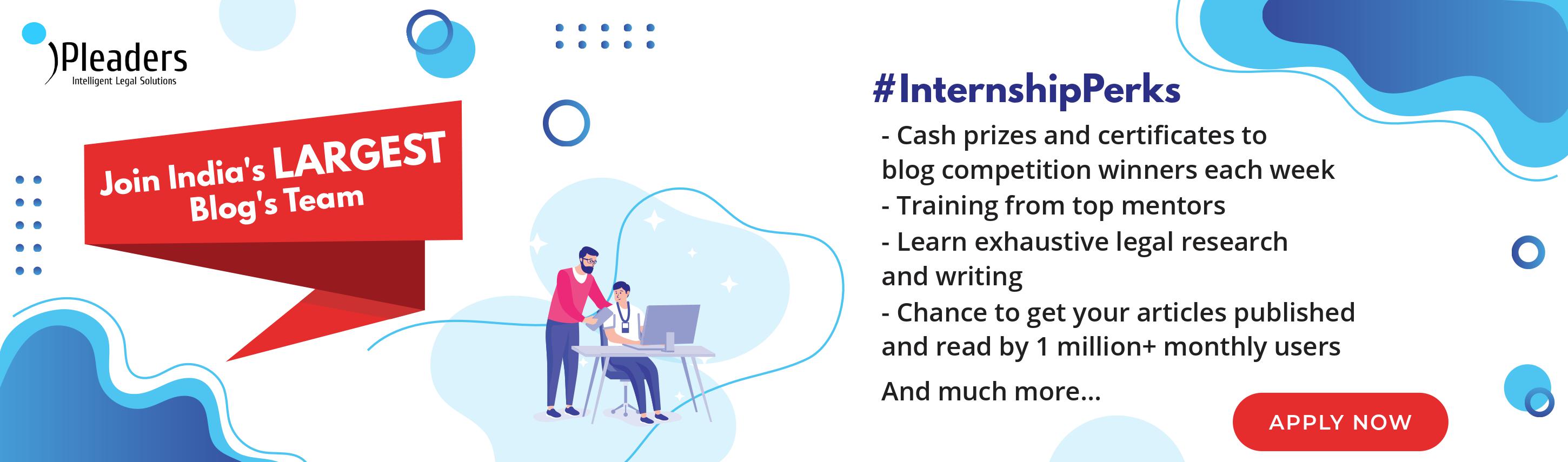